Predicting Crop Yield Using 3D Convolutional Neural Network with Dimension Reduction and Metric Learning
2023 IEEE 6th International Conference on Pattern Recognition and Artificial Intelligence (PRAI)(2023)
Abstract
Crop yield prediction using remote sensing data during the growing season is helpful to farm planning and management, which has received more and more attention. Information mining from multi-channel geo-spatiotemporal data brings many benefits to crop yield prediction. However, most of the existing methods have not fully utilized the dimension reduction technology and the spatiotemporal feature of the data. In this paper, a new approach is proposed to predict the yield from multi-spatial images by using the dimensionality reduction method and the 3D convolutional neural network. In addition, counties with similar crop yields should have similar features learned by the network. Thus, metric learning and multitask learning are used to learn more discriminating features. We evaluate the proposed method on county-level soybean yield prediction in the United States and the experimental results show the effectiveness of the proposed method.
MoreTranslated text
Key words
crop yield prediction,metric learning,3D convolutional neural network,feature constraint,multitask learning
AI Read Science
Must-Reading Tree
Example
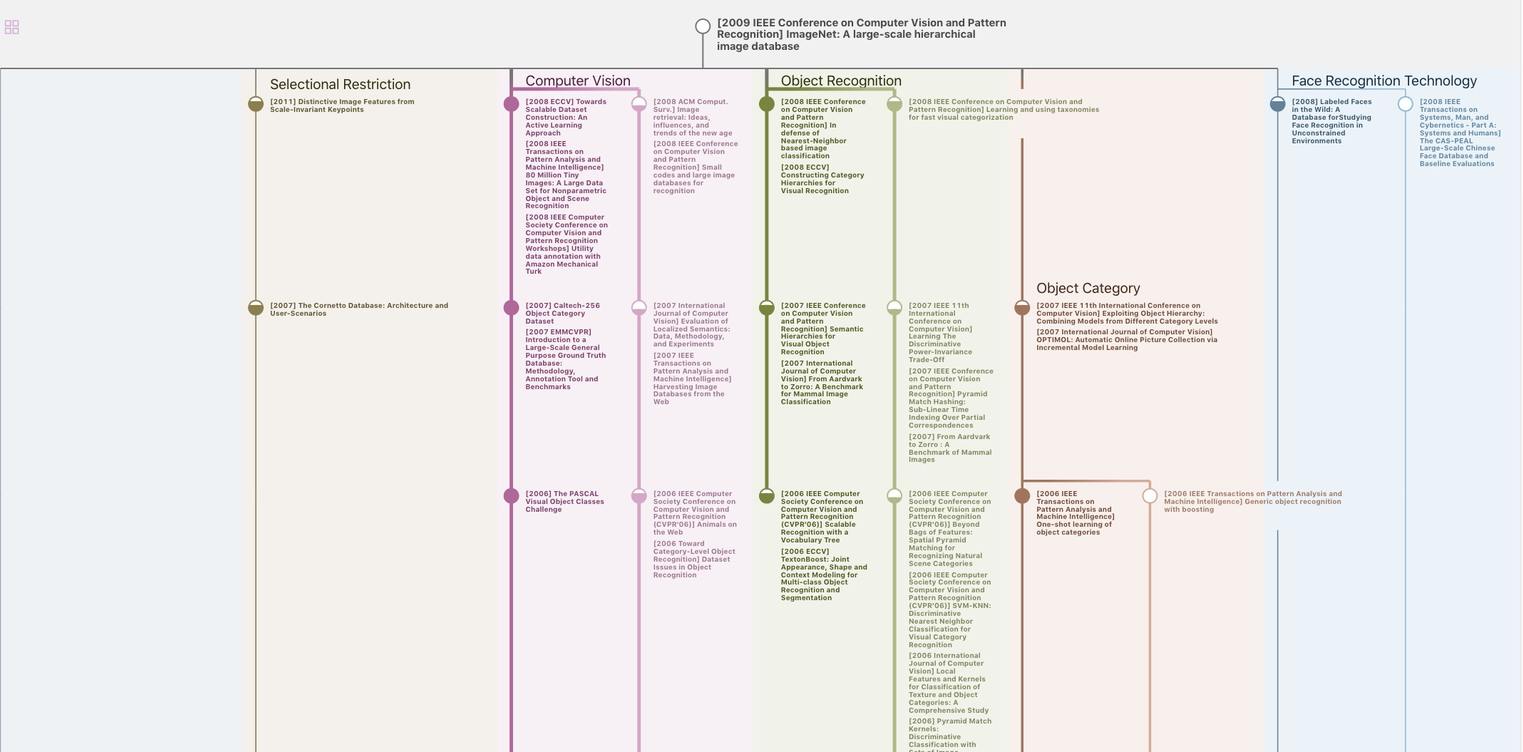
Generate MRT to find the research sequence of this paper
Chat Paper
Summary is being generated by the instructions you defined