Early Alarm: Robust Event Analysis for Power Systems using 1-D Fully Convolutional Network.
2023 IEEE International Conference on Communications, Control, and Computing Technologies for Smart Grids (SmartGridComm)(2023)
摘要
This work presents a novel deep learning model for early, accurate, and robust detection, recognition, and temporal localization of multi-type events in large-scale power systems. The proposed method develops a unified 1-D fully convolutional network (FCN) model that takes time series of raw frequency signals measured from a power system as input, extracts distinguishing features, and predicts at every temporal point in the time series if an event is happening and what the type of the event is. Compared to existing methods, the proposed model eliminates the necessity for hand-crafted feature extraction or complicated data pre-processing, can flexibly handle input signals of arbitrary length, and precisely infer the event occurrence time. Most importantly, the model is intentionally trained with incomplete patterns, such that it is more robust to partial features of an event which is common in real-world online recognition, resulting in early alarm for power system failures. Extensive experimental results demonstrate that the proposed method achieves superior performance to the state-of-the-art, and also shows strong robustness to noise and system oscillations.
更多查看译文
AI 理解论文
溯源树
样例
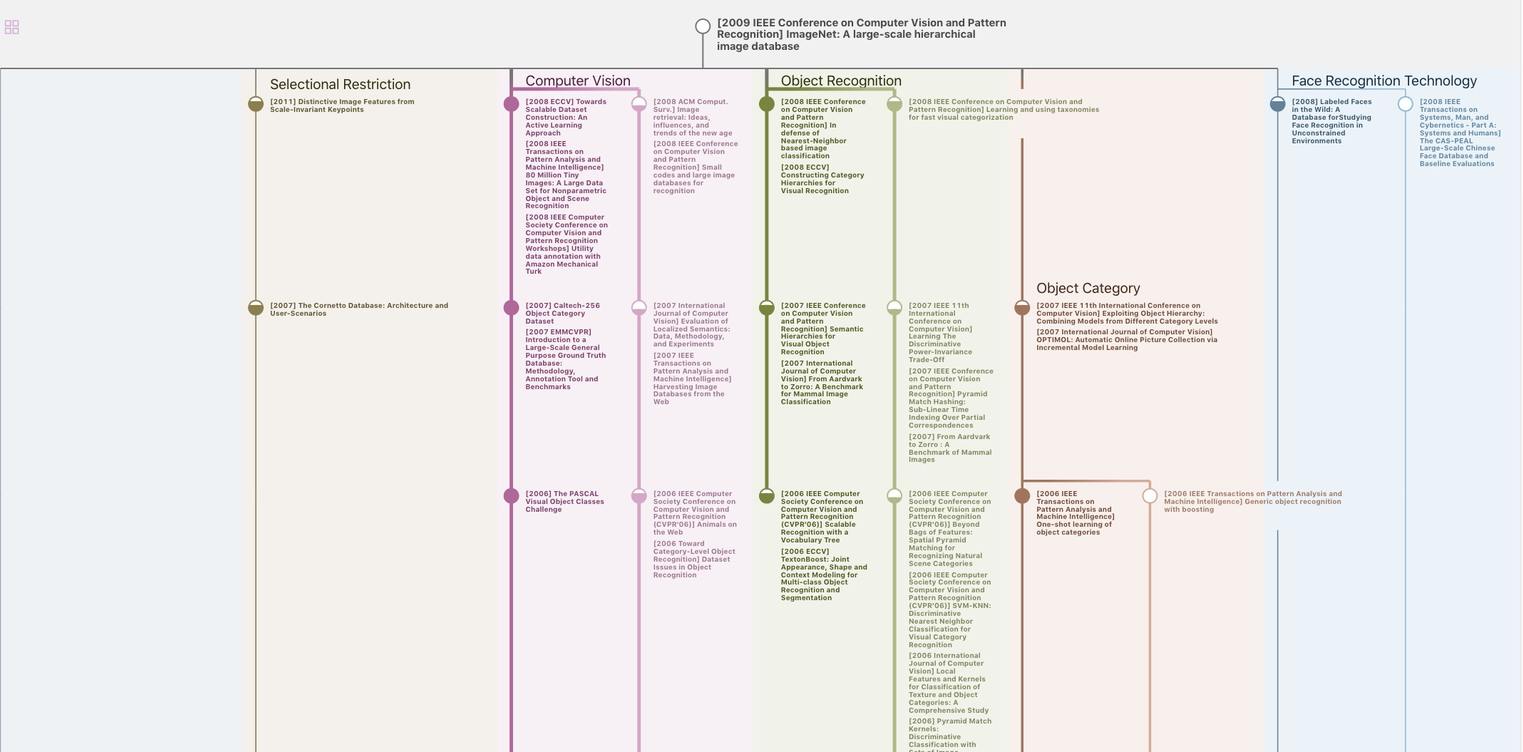
生成溯源树,研究论文发展脉络
Chat Paper
正在生成论文摘要