Cyber-Physical GNN-Based Intrusion Detection in Smart Power Grids.
2023 IEEE International Conference on Communications, Control, and Computing Technologies for Smart Grids (SmartGridComm)(2023)
Abstract
The smart power grid is a critical infrastructure that has been targeted recently by several cyber-attacks. Hence, it is important that advancements are made in intrusion detection systems (IDSs). Recently, promising results have been reported using deep machine learning techniques to develop effective IDSs. However, the existing studies suffer from the following limitations: (a) The adoption of either only physical features (power system measurements) or only cyber features (network logs) in the development of IDSs; (b) The adoption of deep learning techniques that operate on 2D data, while power system measurements are graph-structure data. In this paper, we address these limitations and propose an effective IDS against false data injection and ransomware attacks. Our proposed IDS improves the attack detection performance by (a) fusing cyber-physical features collected from a practical testbed and (b) adopting a topology-aware model based on a graph neural network (GNN) to exploit the spatial and temporal correlation within the data. Our experimental results demonstrate the superior performance of our IDS compared with benchmarks that are based on topology-unaware models and use solely cyber or physical data.
MoreTranslated text
Key words
Intrusion detection systems,false data injection attacks,ransomware attacks,and graph neural networks.
AI Read Science
Must-Reading Tree
Example
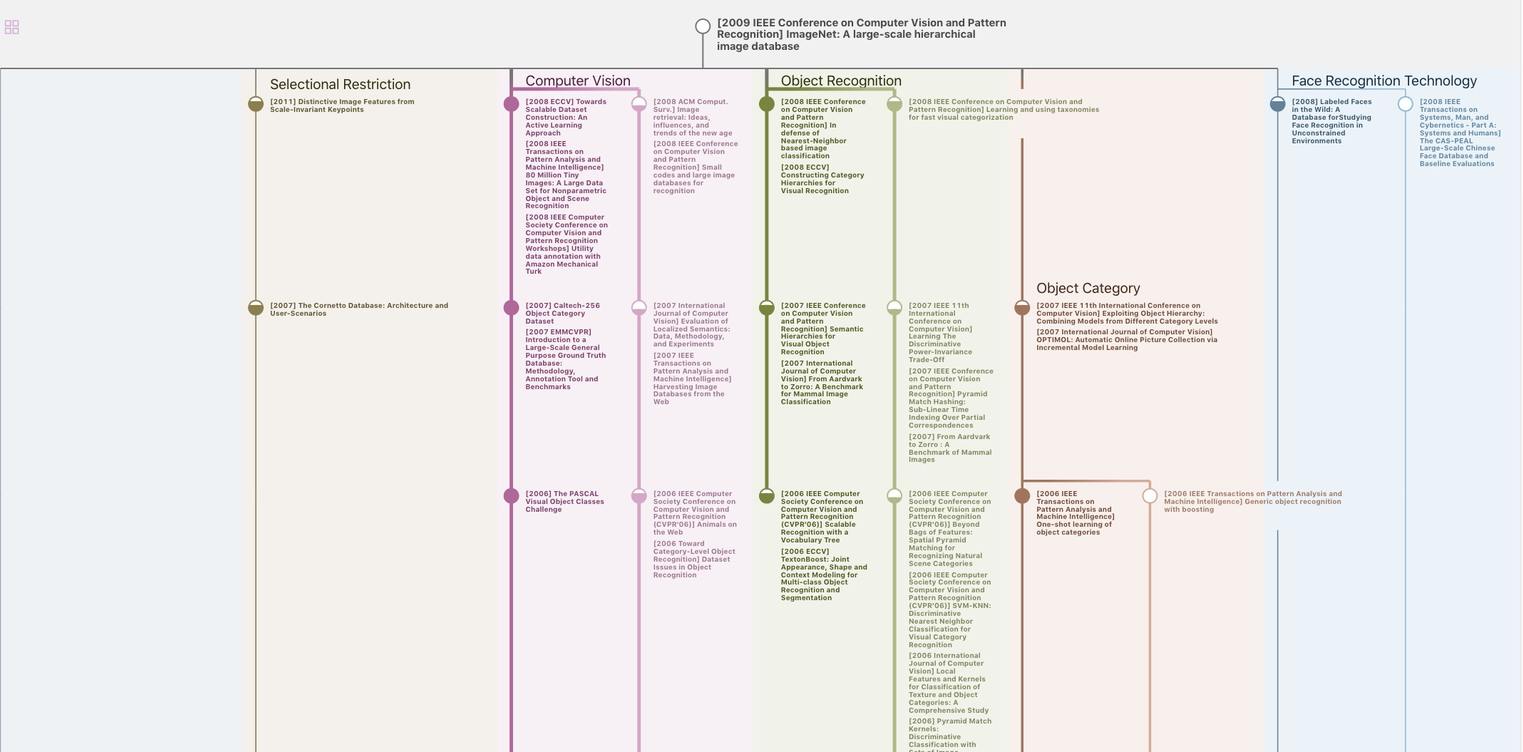
Generate MRT to find the research sequence of this paper
Chat Paper
Summary is being generated by the instructions you defined