Neighbourhood inequity: Exploring the factors underlying racial and ethnic disparities in COVID‐19 testing and infection rates using ZIP code data in Chicago and New York
Regional Science Policy & Practice(2020)
摘要
This paper compares ZIP code‐level data on observed COVID‐19 testing and case rates for the City of Chicago and New York City to better understand both: (i) the extent to which racial and ethnic disparities in COVID‐19 testing and case rates exist at the neighbourhood level; and (ii) the most important neighbourhood‐level drivers of these observed disparities. Through exploratory spatial mapping and econometric approaches, the paper finds that, across both cities, Hispanic‐majority neighbourhoods have significantly lower testing rates than other racial/ethnic neighbourhood types, even when controlling for observed infection rates—which are also significantly higher for Hispanic‐majority neighbourhoods. At the same time, White‐majority neighbourhoods have significantly higher testing rates and lower observed infection rates. Given this observed disparity, the paper also examines a range of underlying factors that are potentially driving observed neighbourhood‐level COVID‐19 case rates. The findings suggest that higher socio‐economic status and the provision of healthy, active built environments are significantly negatively associated with COVID‐19 infection rates, while several aspects of social vulnerability are significant positive predictors of COVID‐19 infection rates. These findings suggest that the health benefits from higher density, walkable built environments may play a larger “protective” role from observed COVID‐19 case rates at the neighbourhood‐level than previously assumed, while at the same time indicating that the increased prevalence of COVID‐19 in Hispanic‐ and Black‐majority neighbourhoods may be in part due to their greater risk of occupational exposure and multi‐generational household structure (particularly for Hispanic‐majority neighbourhoods).
更多查看译文
关键词
cross‐sectional models,economics of minorities and races,health, education, and welfare,land use patterns,non‐labor discrimination,quantile regressions,spatial models,treatment effect models
AI 理解论文
溯源树
样例
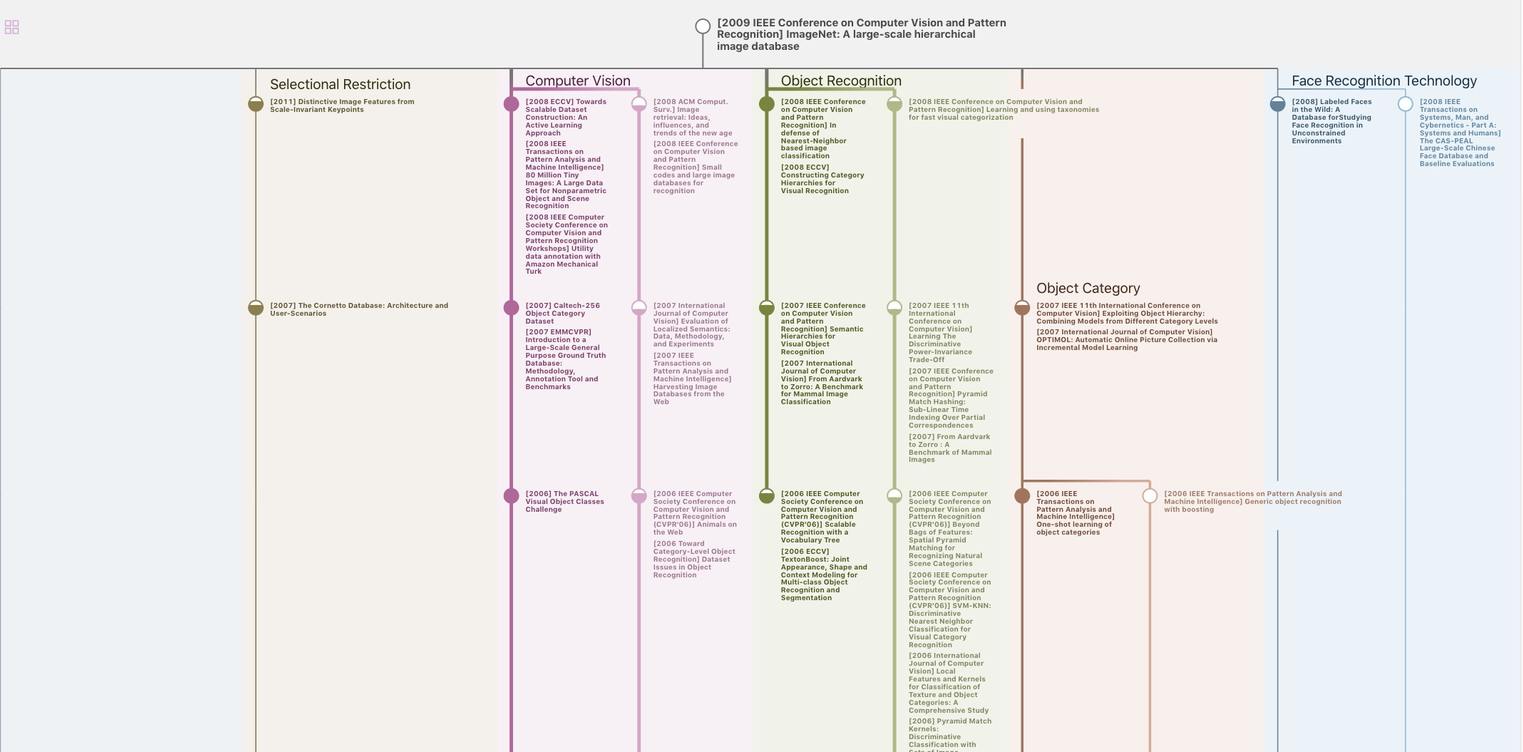
生成溯源树,研究论文发展脉络
Chat Paper
正在生成论文摘要