Influence of Coronary Calcium on Diagnostic Performance of Machine Learning CT-FFR
JACC: Cardiovascular Imaging(2020)
摘要
This study was conducted to investigate the influence of coronary artery calcium (CAC) score on the diagnostic performance of machine-learning–based coronary computed tomography (CT) angiography (cCTA)–derived fractional flow reserve (CT-FFR). CT-FFR is used reliably to detect lesion-specific ischemia. Novel CT-FFR algorithms using machine-learning artificial intelligence techniques perform fast and require less complex computational fluid dynamics. Yet, influence of CAC score on diagnostic performance of the machine-learning approach has not been investigated. A total of 482 vessels from 314 patients (age 62.3 ± 9.3 years, 77% male) who underwent cCTA followed by invasive FFR were investigated from the MACHINE (Machine Learning based CT Angiography derived FFR: a Multi-center Registry) registry data. CAC scores were quantified using the Agatston convention. The diagnostic performance of CT-FFR to detect lesion-specific ischemia was assessed across all Agatston score categories (CAC 0, >0 to <100, 100 to <400, and ≥400) on a per-vessel level with invasive FFR as the reference standard. The diagnostic accuracy of CT-FFR versus invasive FFR was superior to cCTA alone on a per-vessel level (78% vs. 60%) and per patient level (83% vs. 73%) across all Agatston score categories. No statistically significant differences in the diagnostic accuracy, sensitivity, or specificity of CT-FFR were observed across the categories. CT-FFR showed good discriminatory power in vessels with high Agatston scores (CAC ≥400) and high performance in low-to-intermediate Agatston scores (CAC >0 to <400) with a statistically significant difference in the area under the receiver-operating characteristic curve (AUC) (AUC: 0.71 [95% confidence interval (CI): 0.57 to 0.85] vs. 0.85 [95% CI: 0.82 to 0.89], p = 0.04). CT-FFR showed superior diagnostic value over cCTA in vessels with high Agatston scores (CAC ≥ 400: AUC 0.71 vs. 0.55, p = 0.04) and low-to-intermediate Agatston scores (CAC >0 to <400: AUC 0.86 vs. 0.63, p < 0.001). Machine-learning–based CT-FFR showed superior diagnostic performance over cCTA alone in CAC with a significant difference in the performance of CT-FFR as calcium burden/Agatston calcium score increased. (Machine Learning Based CT Angiography Derived FFR: a Multicenter, Registry [MACHINE] NCT02805621).
更多查看译文
关键词
coronary calcium,diagnostic performance,ct-ffr
AI 理解论文
溯源树
样例
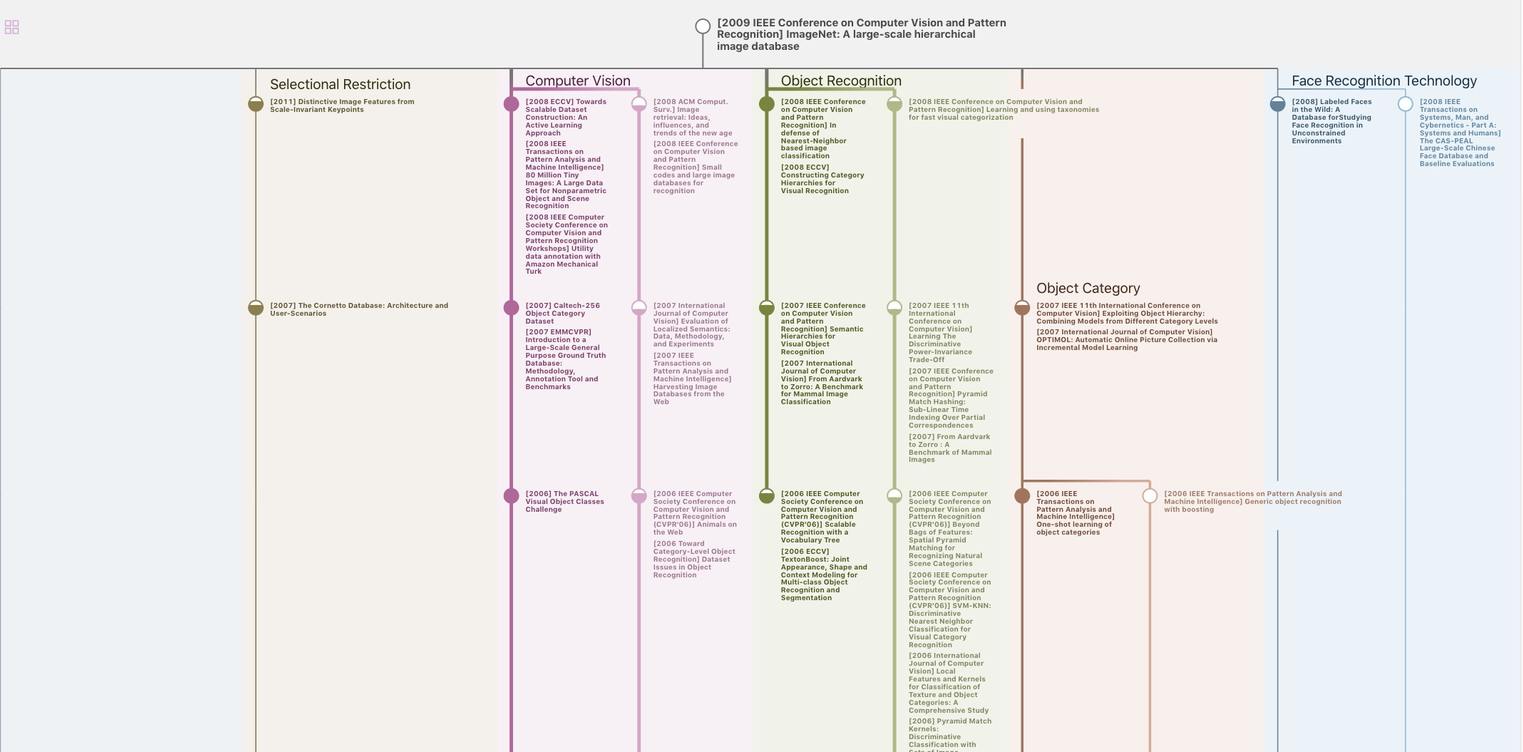
生成溯源树,研究论文发展脉络
Chat Paper
正在生成论文摘要