Model Reduction by Least Squares Moment Matching for Linear and Nonlinear Systems
IEEE TRANSACTIONS ON AUTOMATIC CONTROL(2023)
摘要
The paper studies the model reduction problem using the notion of least squares moment matching. For linear systems, the main idea is to approximate a transfer function by ensuring that the interpolation conditions imposed by moment matching are satisfied in a least squares sense. The paper revisits this idea using tools from output regulation theory to develop a nonlinear enhancement of the notion of least squares moment matching and a unifying model reduction framework both for linear and nonlinear systems. The proposed framework allows for the direct computation of models through optimization, the use of weights to modulate the quality of approximation, and the possibility of enforcing prescribed properties, such as stability and passivity, via additional constraints. Parameterized families of models achieving least squares moment matching are also determined and shown to admit natural geometric and system-theoretic interpretations. The theory is illustrated by numerical examples.
更多查看译文
关键词
Interpolation,least squares methods,reduced or-der systems,systems modeling
AI 理解论文
溯源树
样例
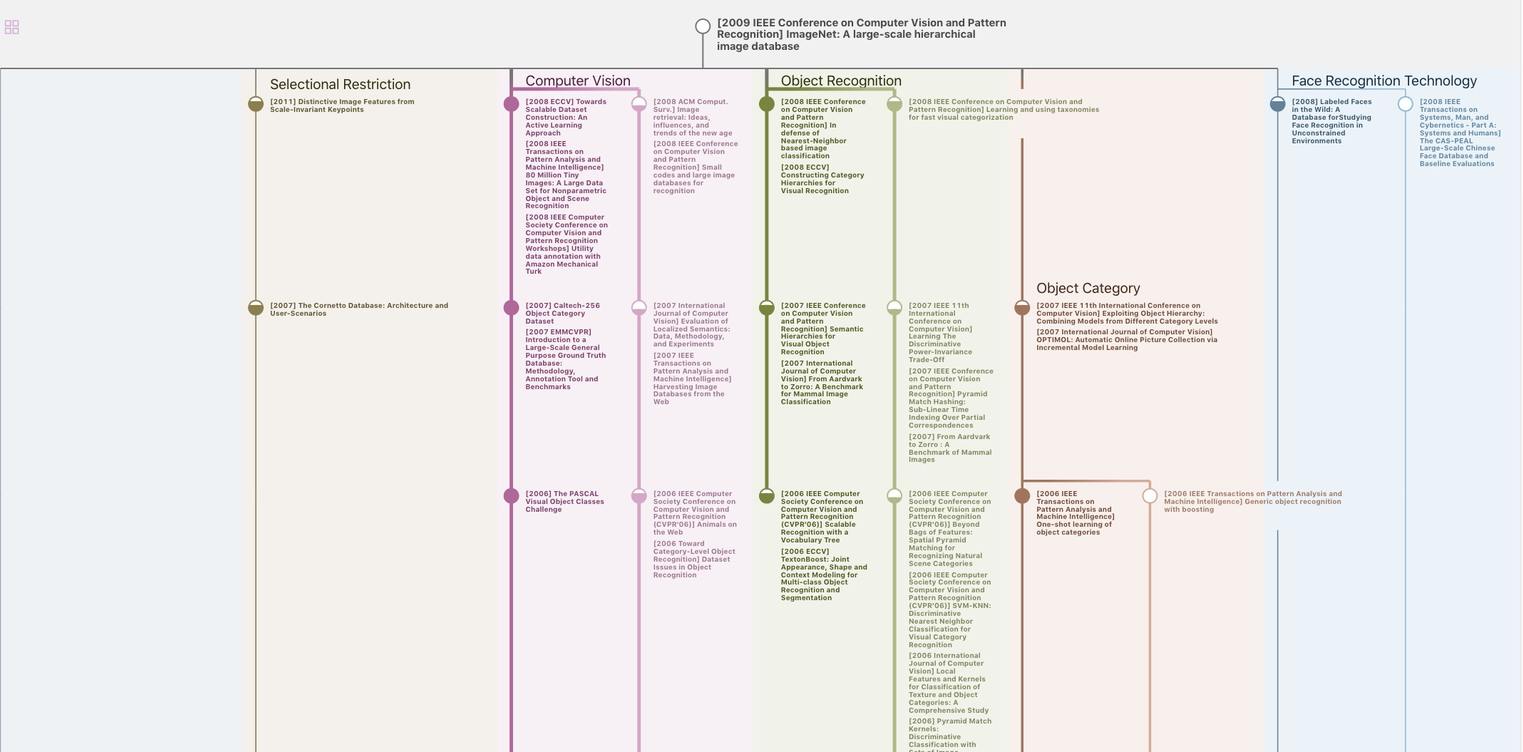
生成溯源树,研究论文发展脉络
Chat Paper
正在生成论文摘要