Asymptotic Theory for Regularized System Identification Part I: Empirical Bayes Hyperparameter Estimator
IEEE TRANSACTIONS ON AUTOMATIC CONTROL(2023)
摘要
Regularized techniques, also named as kernel-based techniques, are the major advances in system identification in the last decade. Although many promising results have been achieved, their theoretical analysis is far from complete and there are still many key problems to be solved. One of them is the asymptotic theory, which is about convergence properties of the model estimators as the sample size goes to infinity. The existing related results for regularized system identification are about the almost sure convergence of various hyperparameter estimators. A common problem of those results is that they do not contain information on the factors that affect the convergence properties of those hyperparameter estimators, e.g., the regression matrix. In this article, we tackle problems of this kind for the regularized finite impulse response model estimation with the empirical Bayes (EB) hyperparameter estimator and filtered white noise input. In order to expose and find those factors, we study the convergence in distribution of the EB hyperparameter estimator, and the asymptotic distribution of its corresponding model estimator. For illustration, we run Monte Carlo simulations to show the efficacy of our obtained theoretical results.
更多查看译文
关键词
Asymptotic distribution,asymptotic theory,empirical Bayes (EB),hyperparameter estimator,regularized least squares (RLS),ridge regression
AI 理解论文
溯源树
样例
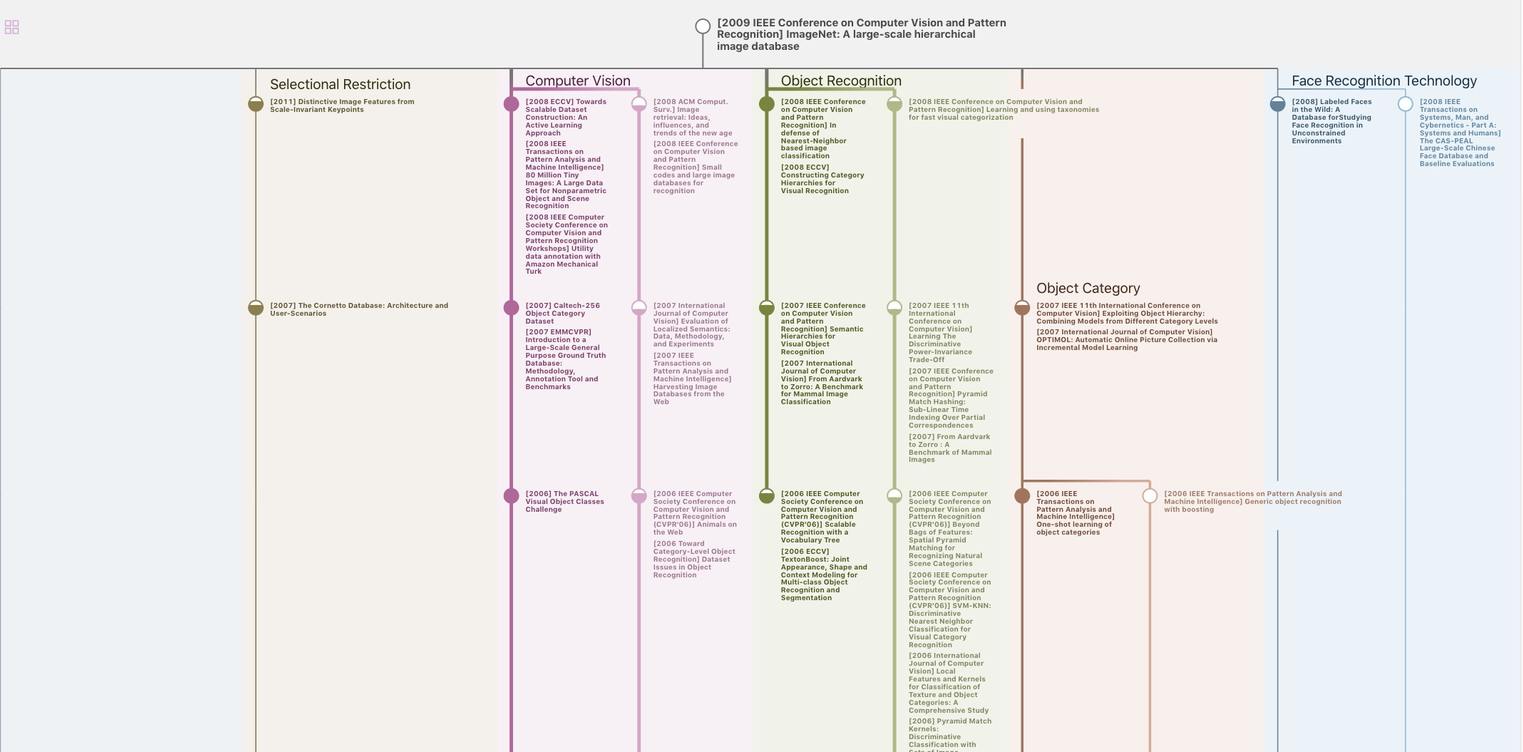
生成溯源树,研究论文发展脉络
Chat Paper
正在生成论文摘要