STELLAR: Siamese Multi-Headed Attention Neural Networks for Overcoming Temporal Variations and Device Heterogeneity with Indoor Localization
IEEE Journal of Indoor and Seamless Positioning and Navigation(2023)
摘要
Smartphone-based indoor localization has emerged as a cost-effective and
accurate solution to localize mobile and IoT devices indoors. However, the
challenges of device heterogeneity and temporal variations have hindered its
widespread adoption and accuracy. Towards jointly addressing these challenges
comprehensively, we propose STELLAR, a novel framework implementing a
contrastive learning approach that leverages a Siamese multi-headed attention
neural network. STELLAR is the first solution that simultaneously tackles
device heterogeneity and temporal variations in indoor localization, without
the need for retraining the model (re-calibration-free). Our evaluations across
diverse indoor environments show 8-75% improvements in accuracy compared to
state-of-the-art techniques, to effectively address the device heterogeneity
challenge. Moreover, STELLAR outperforms existing methods by 18-165% over 2
years of temporal variations, showcasing its robustness and adaptability.
更多查看译文
关键词
Device heterogeneity,indoor localization,machine learning (ML),neural networks,temporal variation,Wi-Fi fingerprinting
AI 理解论文
溯源树
样例
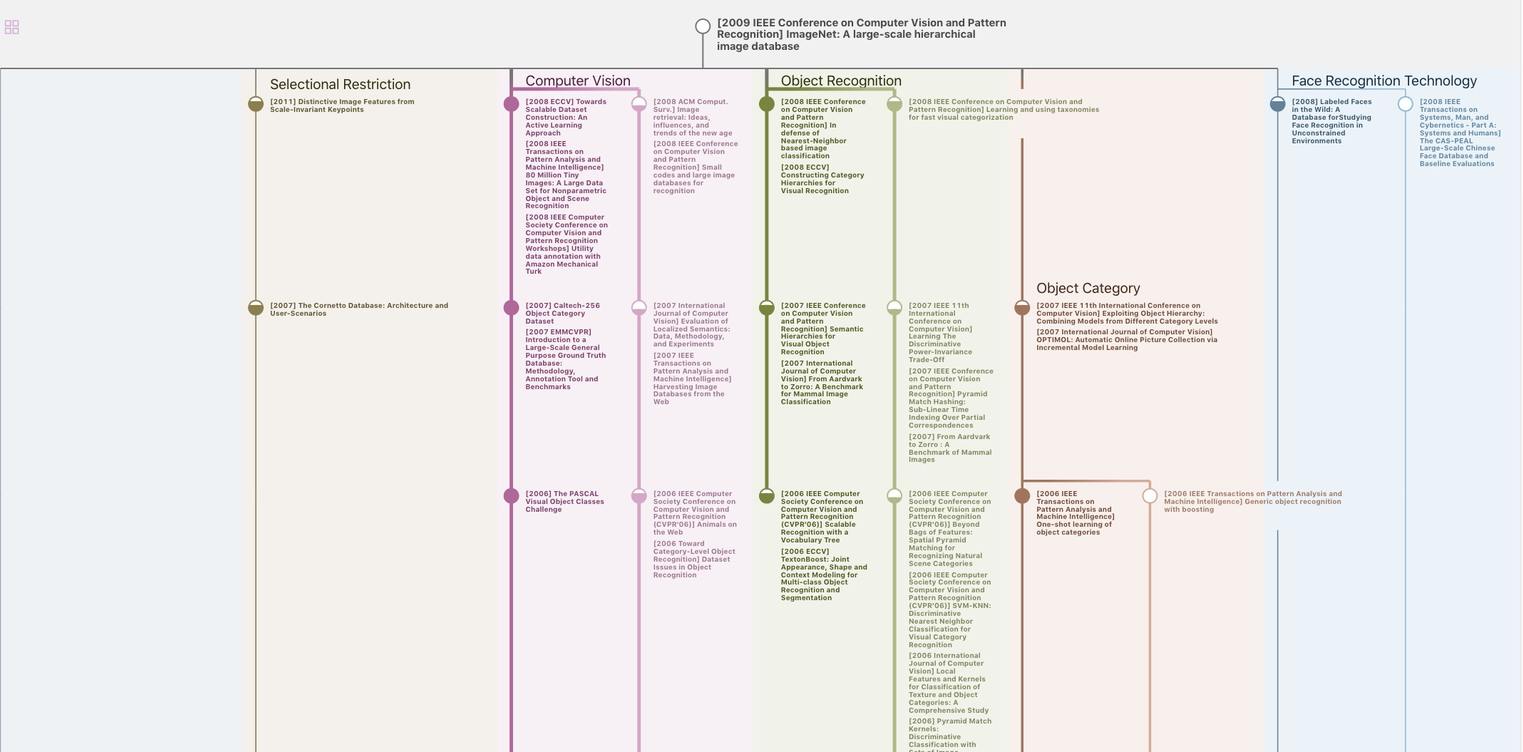
生成溯源树,研究论文发展脉络
Chat Paper
正在生成论文摘要