Graph-Based Information Separator and Area Convolutional Network for EEG-Based Intention Decoding
IEEE TRANSACTIONS ON COGNITIVE AND DEVELOPMENTAL SYSTEMS(2024)
摘要
In the current research on brain-computer interface (BCI), the electroencephalography (EEG) signal is usually only represented by a 2-D matrix, and the installation position of the EEG electrodes and the correlation between them are not considered. Actually, the cerebral cortex is a continuous potential surface and the information collected directly from each electrode is influenced by the other electrodes, so direct use of the raw data results in information redundancy. This article converts the EEG signal into a graph, and then creates an information separator (IS) based on the Laplace matrix of the graph to obtain the independent source information of electrode nodes, and propose an IS-based area convolutional network (IS-ACN). Integrating the proposed IS with some advanced methods, the experimental results show that the incorporation of IS can enhance the performance of these methods. By observing and tracking samples with abnormal noise in the BCI competition IV data set 2a, it is demonstrated that the proposed method can greatly reduce the influence of noise and effectively obtain the source features of EEG signals with low signal-to-noise ratio, and the average accuracy and kappa coefficient of the proposed IS-ACN on this data set are 80.59% and 74.1%, respectively.
更多查看译文
关键词
Electroencephalography,Electrodes,Feature extraction,Convolutional neural networks,Convolution,Correlation,Symmetric matrices,Area convolutional network (ACN),electroencephalography (EEG),graph,information separator (IS),intention decoding
AI 理解论文
溯源树
样例
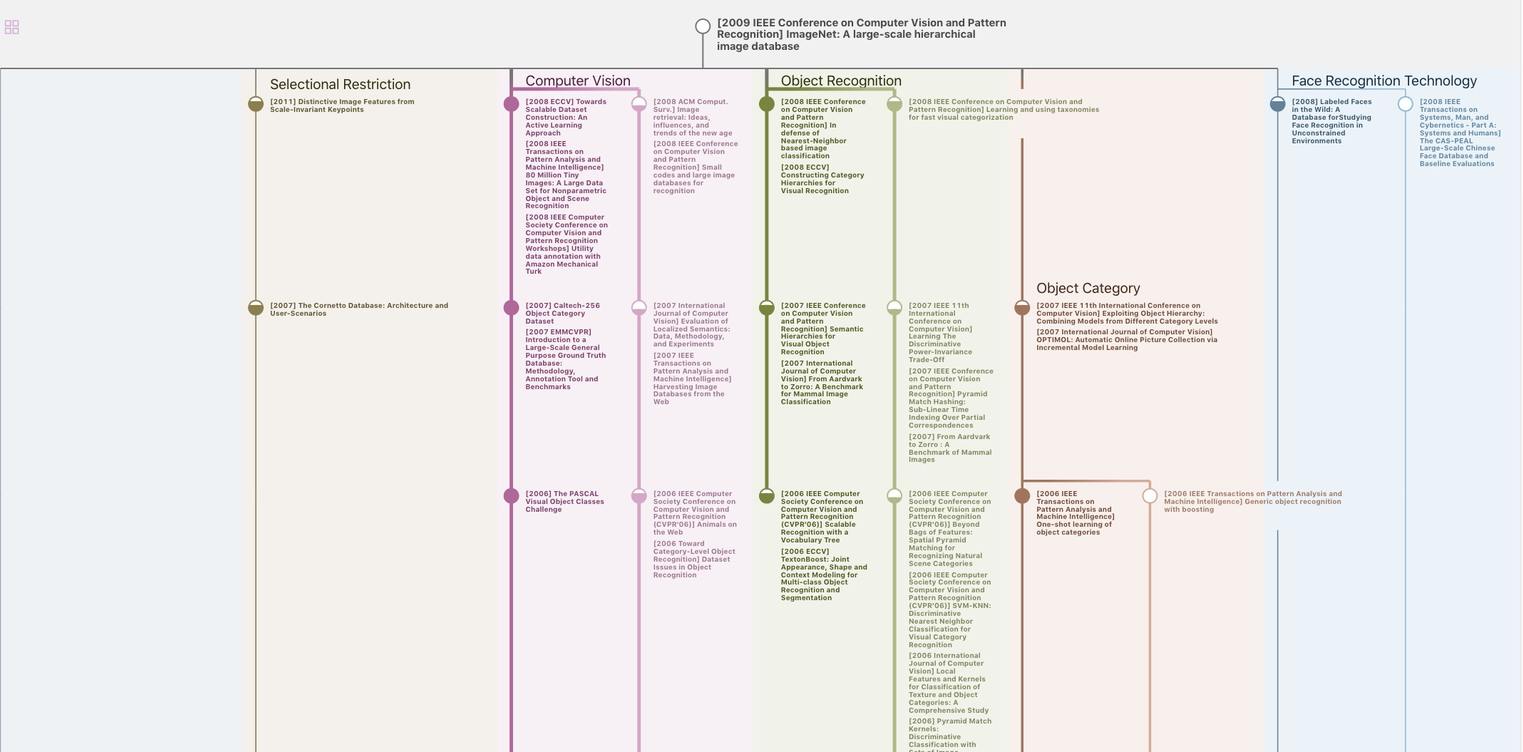
生成溯源树,研究论文发展脉络
Chat Paper
正在生成论文摘要