Visualization of Leaf Botanical Features Extracted from AlexNet Convolutional Layers
HAL (Le Centre pour la Communication Scientifique Directe)(2018)
摘要
To recognize tree species from pictures of their leaves, one way is to automatically extract the features botanists look at for identification and use them in a classification system. This is the approach developed in the Folia application. Another way that has emerged in the past years is to train Convolutional Neural Networks (CNN) to recognize plants directly from pictures, as done by many researchers in the PlantCLEF challenge. CNN-based methods have spread into many fields as they generally give the best results, but can arguably be considered a black box. The aim of our work is to try to understand their core and establish a link between the features extracted through such networks and botanical characteristics of the species, in order to improve expressiveness and propose alternative recognition algorithms. For our study, we worked with the widely used AlexNet network. Its performances are inferior to those of the most recent CNN (GoogLeNet, ResNet, VGGNet) but its linear architecture allows to visualize more easily the different convolutional layers. Thus, we chose to train AlexNet on an image database of 72 simple-leaved species developed by the PlantCLEF challenge, where leaves are shot on a plain color sheet (no complex background). We trained AlexNet from scratch to construct its convolutional filters directly on plant organ elements. As the entry of AlexNet is a 256x256 image, the images have been resized and filled with random values on the borders to preserve the aspect ratio of the leaves. Then, we have visualized the obtained filters and analysed them.
更多查看译文
关键词
leaf botanical features,visualization,layers
AI 理解论文
溯源树
样例
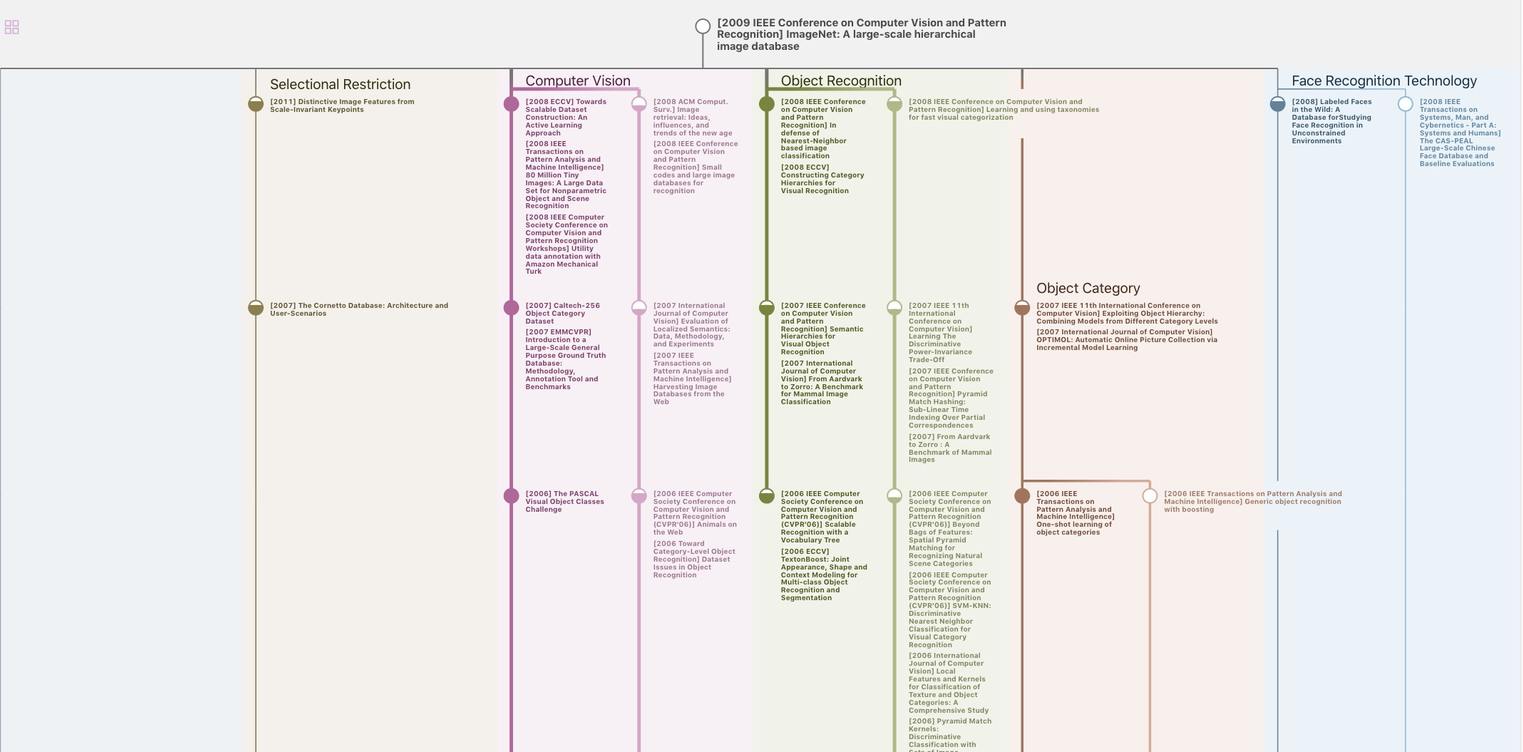
生成溯源树,研究论文发展脉络
Chat Paper
正在生成论文摘要