Overcoming catastrophic forgetting in neural networks
Proceedings of the National Academy of Sciences of the United States of America(2017)
摘要
Significance Deep neural networks are currently the most successful machine-learning technique for solving a variety of tasks, including language translation, image classification, and image generation. One weakness of such models is that, unlike humans, they are unable to learn multiple tasks sequentially. In this work we propose a practical solution to train such models sequentially by protecting the weights important for previous tasks. This approach, inspired by synaptic consolidation in neuroscience, enables state of the art results on multiple reinforcement learning problems experienced sequentially.
更多查看译文
关键词
catastrophic forgetting,neural networks
AI 理解论文
溯源树
样例
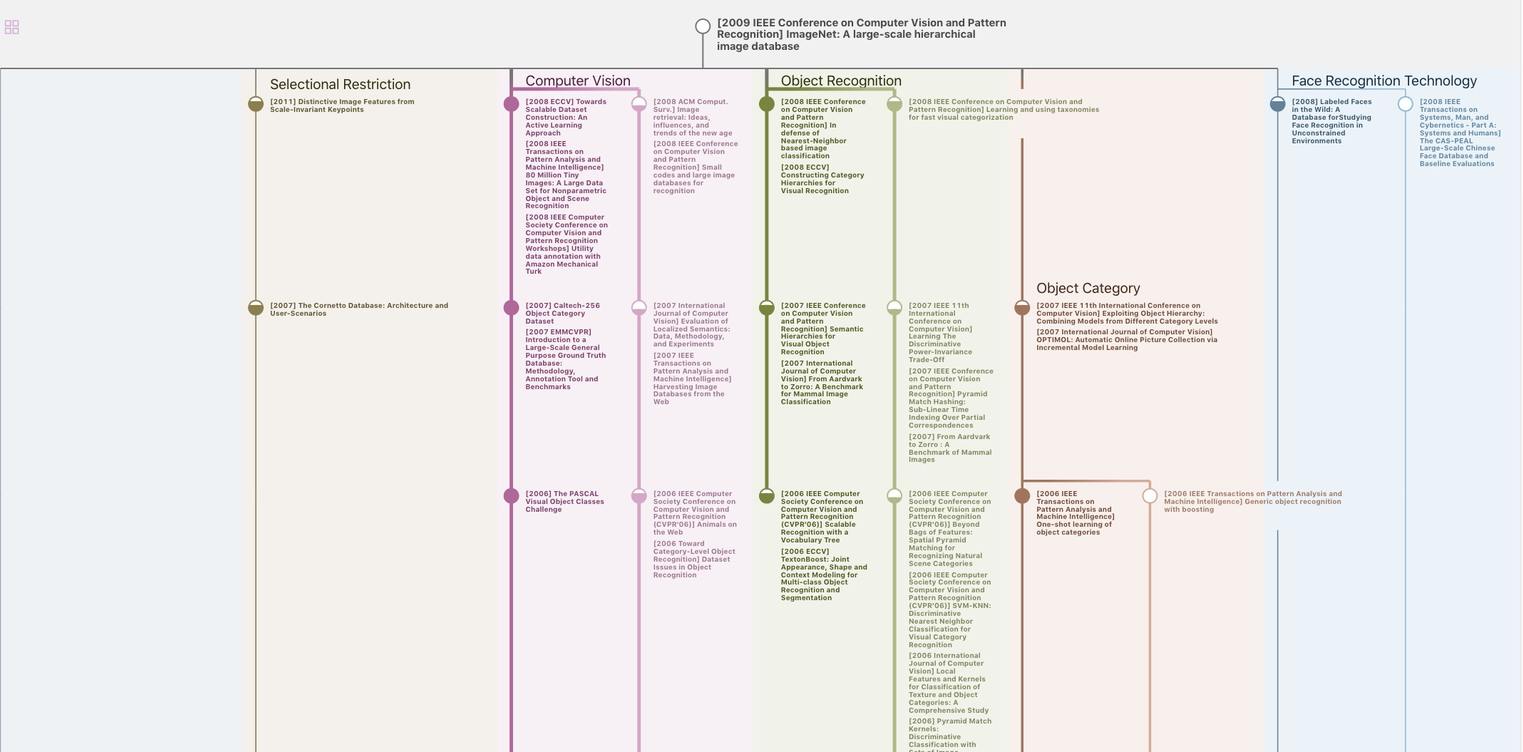
生成溯源树,研究论文发展脉络
Chat Paper
正在生成论文摘要