Conditional generative adversarial network based data augmentation for fault diagnosis of diesel engines applied with infrared thermography and deep convolutional neural network
EKSPLOATACJA I NIEZAWODNOSC-MAINTENANCE AND RELIABILITY(2024)
Abstract
This paper tries to introduce a new intelligent method for the early fault diagnosis of diesel engines. Firstly, infrared thermography (IRT) is introduced into diesel engine condition monitoring, then infrared images of diesel engines in four health states, such as normal condition, singlecylinder misfire, multi -cylinder misfire and air filter blockage, are collected and the region of interest (ROI) of infrared images are extracted. Next, conditional generative adversarial network (CGAN) is deployed to perform data augmentation on infrared image datasets. Then, deep convolutional neural network (DCNN) and Softmax regression (SR) classifier are used for automatically extracting infrared image fault features and pattern recognition, respectively. Finally, a comparison with three deep learning (DL) models is performed. The validation results show that the data augmentation method proposed in the paper can significantly improve the early fault diagnosis accuracy, and DCNN has the best fault diagnosis effect and resistance to temperature fluctuation interference among the four DL models.
MoreTranslated text
Key words
diesel engines,data augmentation,conditional generative adversarial network,infrared thermography,deep convolutional neural network,fault diagnosis
AI Read Science
Must-Reading Tree
Example
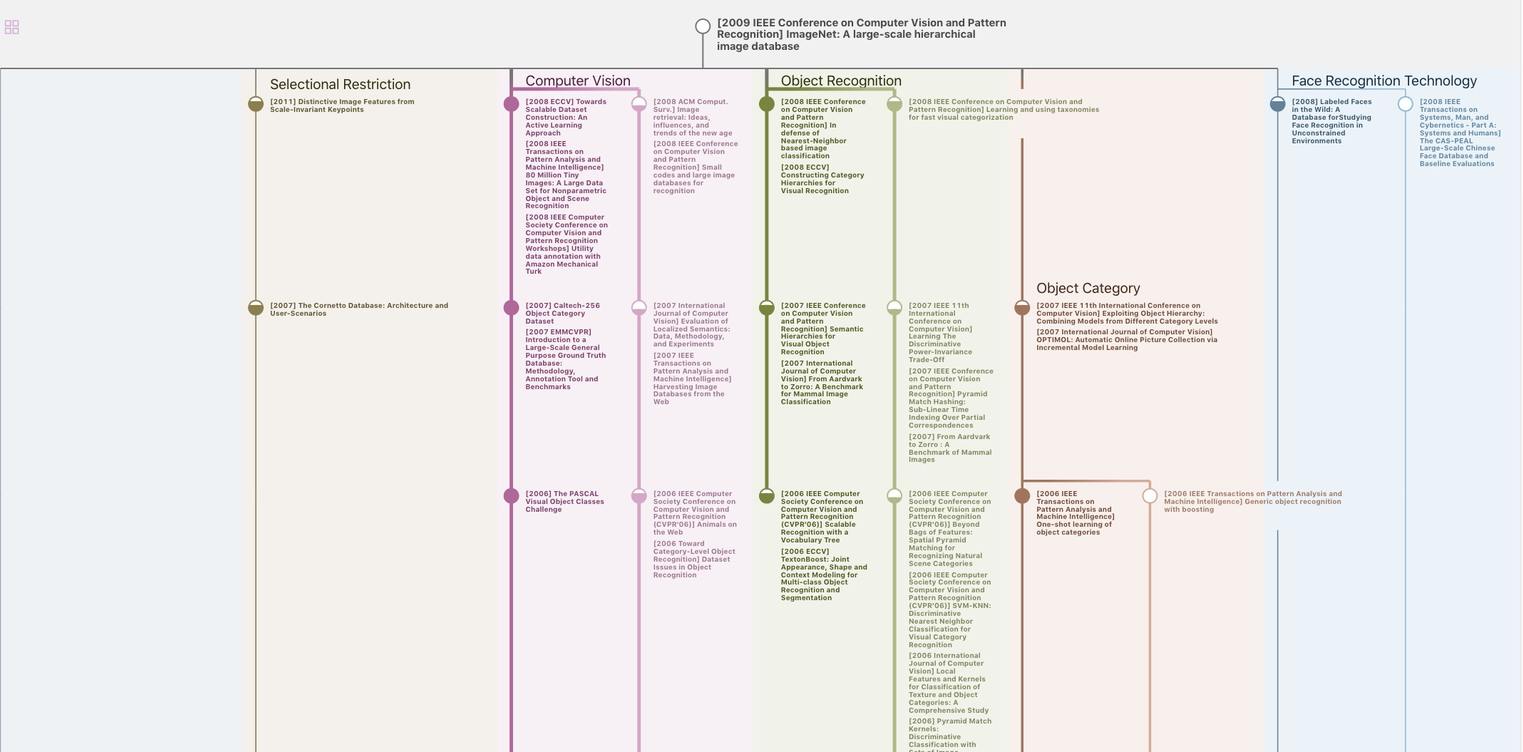
Generate MRT to find the research sequence of this paper
Chat Paper
Summary is being generated by the instructions you defined