Enhancing Indoor Localization With Semi-Crowdsourced Fingerprinting and GAN-Based Data Augmentation
IEEE Internet of Things Journal(2023)
摘要
The popularity of radio frequency (RF)-based fingerprinting for indoor localization has grown owing to its relatively low cost of equipment deployment and satisfactory accuracy. However, generating a complete radio map by associating unlabeled RF signals with the corresponding location information remains challenging, especially in crowdsourcing-based fingerprinting. In this paper, we propose a semi-crowdsourced radio map construction method based on Bluetooth low energy (BLE) landmarks that harnesses reference points in the radio map for coarse localization and facilitates the labeling of location information to WiFi signals. Principally, we acquire RF-received signal strength (RSS) measurements annotating them with location coordinates recorded while a user is walking to provide an efficient method of data collection. Furthermore, we introduce a generative adversarial network (GAN)-based method to increase the amount of training data collected at each reference point and reduce human effort by augmenting the fingerprint database. Our proposed method demonstrates promising results, including improved localization accuracy and localization performance comparable to that of traditional site surveys while reducing measurement time and human effort.
更多查看译文
关键词
Mobile crowdsourcing,reinforcement learning,BLE landmark-based,WiFi fingerprint positioning,generative adversarial networks,Internet of Things
AI 理解论文
溯源树
样例
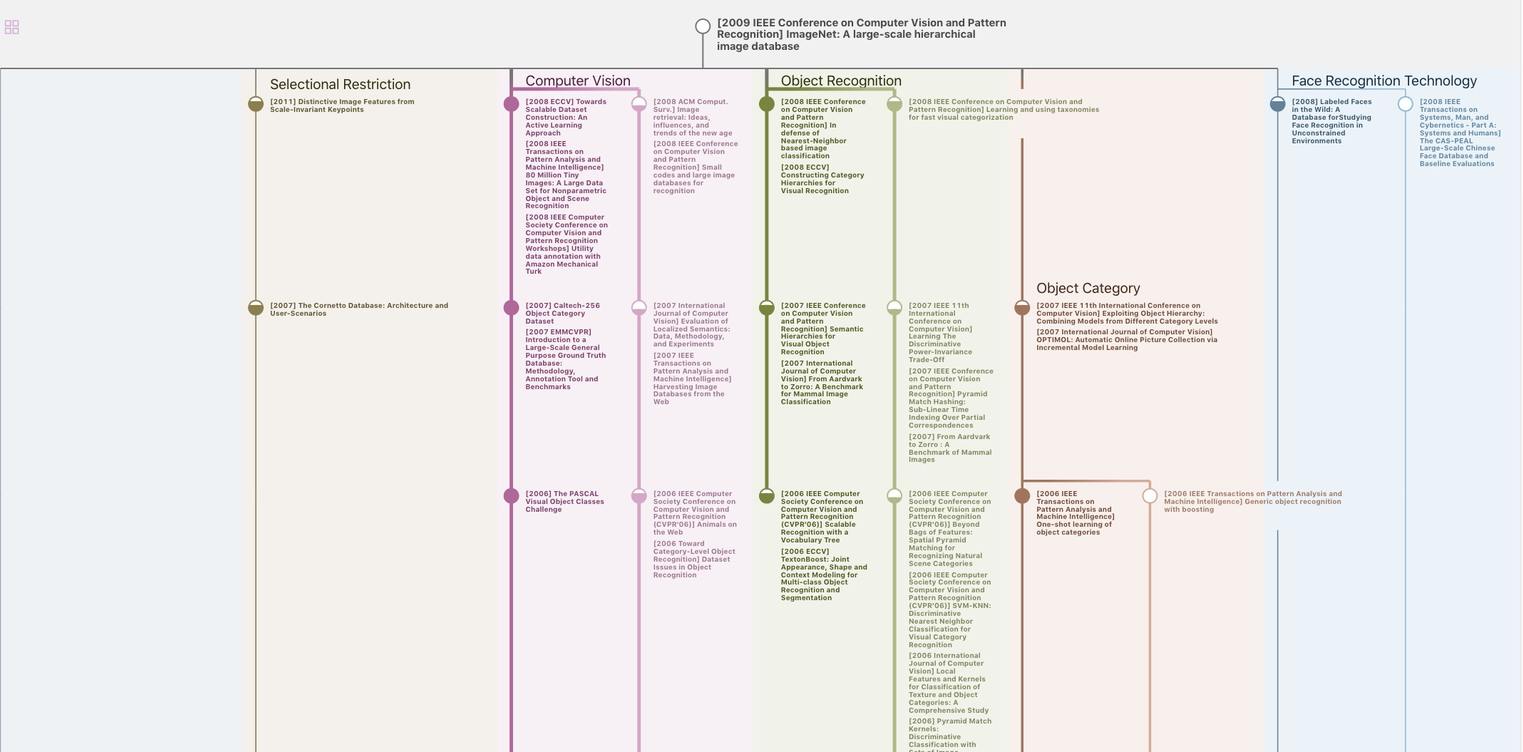
生成溯源树,研究论文发展脉络
Chat Paper
正在生成论文摘要