DynamicAug: Enhancing Transfer Learning Through Dynamic Data Augmentation Strategies Based on Model State
Neural Processing Letters(2024)
摘要
Transfer learning has made significant advancements, however, the issue of overfitting continues to pose a major challenge. Data augmentation has emerged as a highly promising technique to counteract this challenge. Current data augmentation methods are fixed in nature, requiring manual determination of the appropriate intensity prior to the training process. However, this entails substantial computational costs. Additionally, as the model approaches convergence, static data augmentation strategies can become suboptimal. In this paper, we introduce the concept of Dynamic Data Augmentation (DynamicAug), a method that autonomously adjusts the intensity of data augmentation, taking into account the convergence state of the model. During each iteration of the model’s forward pass, we utilize a Gaussian distribution based sampler to stochastically sample the current intensity of data augmentation. To ensure that the sampled intensity is aligned with the convergence state of the model, we introduce a learnable expectation to the sampler and update the expectation iteratively. In order to assess the convergence status of the model, we introduce a novel loss function called the convergence loss. Through extensive experiments conducted over 27 vision datasets, we have demonstrated that DynamicAug can significantly enhance the performance of existing transfer learning methods.
更多查看译文
关键词
Deep learning,Transfer learning,Data augmentation,Vision transformer
AI 理解论文
溯源树
样例
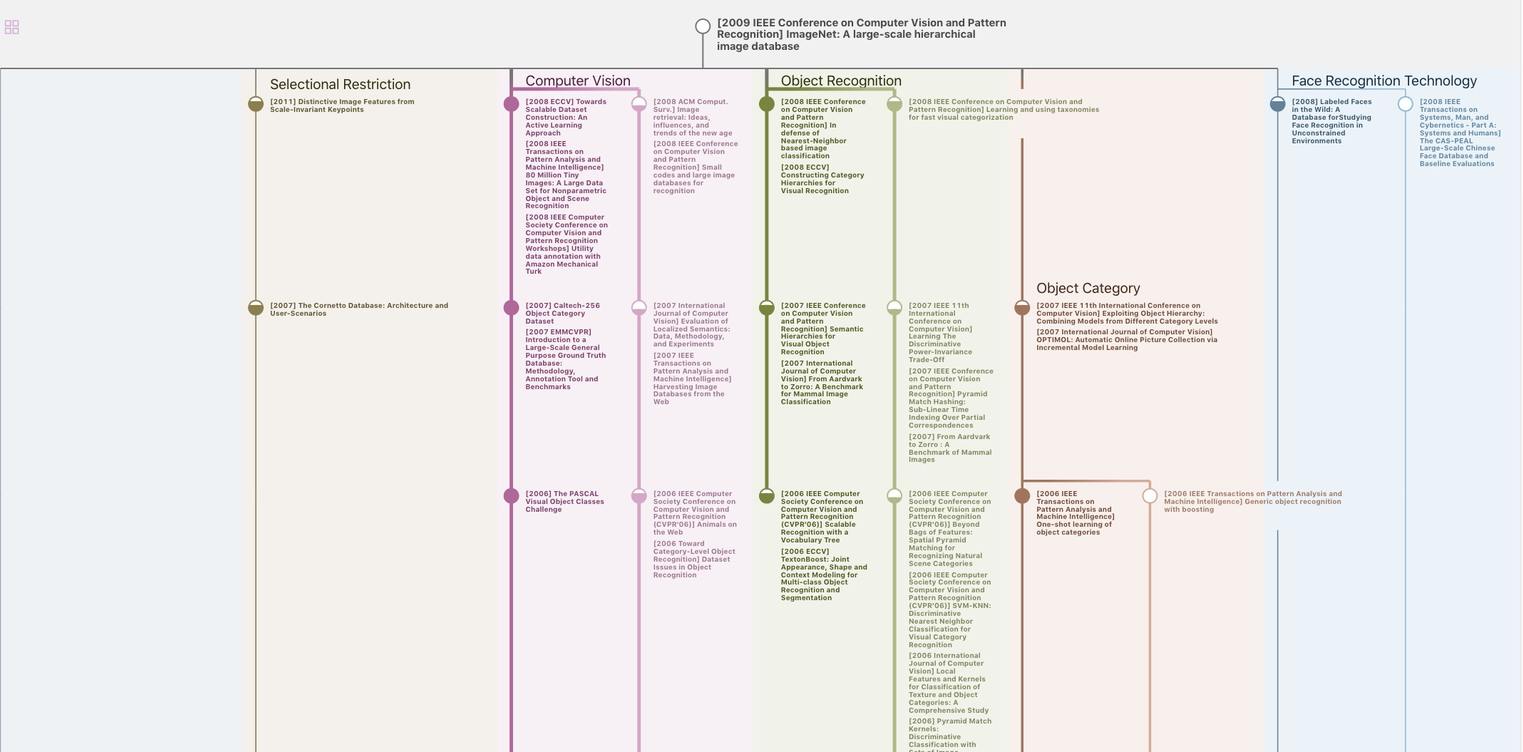
生成溯源树,研究论文发展脉络
Chat Paper
正在生成论文摘要