Machine Learning Based Prediction of Postoperative Pancreatic Fistula Following Pancreaticoduodenectomy
Annals of Surgery(2023)
摘要
To develop a novel machine learning (ML) model to predict clinically relevant postoperative pancreatic fistula (CR-POPF) following pancreaticoduodenectomy (PD).Accurate prognostication of CR-POPF may allow for risk stratification and adaptive treatment strategies for potential PD candidates. However, antecedent models, such as the modified Fistula Risk Score (mFRS), are limited by poor discrimination and calibration.All records entailing PD within the 2014-2018 ACS NSQIP were identified. Additionally, patients undergoing PD at our institution between 2013 and 2021 were queried from our local data repository. An eXtreme Gradient Boosting (XGBoost) model was developed to estimate the risk of CR-POPF using data from the ACS NSQIP and evaluated using institutional data. Model discrimination was estimated using the area under the receiver operating characteristic (AUROC) and precision recall curve (AUPRC).Overall, 12,281 and 445 patients undergoing PD were identified within the 2014-2018 ACS NSQIP and our institutional registry, respectively. Application of the XGBoost and mFRS scores to the internal validation dataset revealed that the former model had significantly greater AUROC (0.72 vs. 0.68, P<0.001) and AUPRC (0.22 vs. 0.18, P<0.001). Within the external validation dataset, the XGBoost model remained superior to the mFRS with an AUROC of 0.79 (95% CI 0.74-0.84) versus 0.75 (95% CI 0.70-0.80, P<0.001). In addition, AUPRC was higher for the XGBoost model, compared to the mFRS.Our novel ML model consistently outperformed the previously validated mFRS within internal and external validation cohorts, thereby demonstrating its generalizability and utility for enhancing prediction of CR-POPF.
更多查看译文
关键词
postoperative pancreatic fistula,machine learning,prediction
AI 理解论文
溯源树
样例
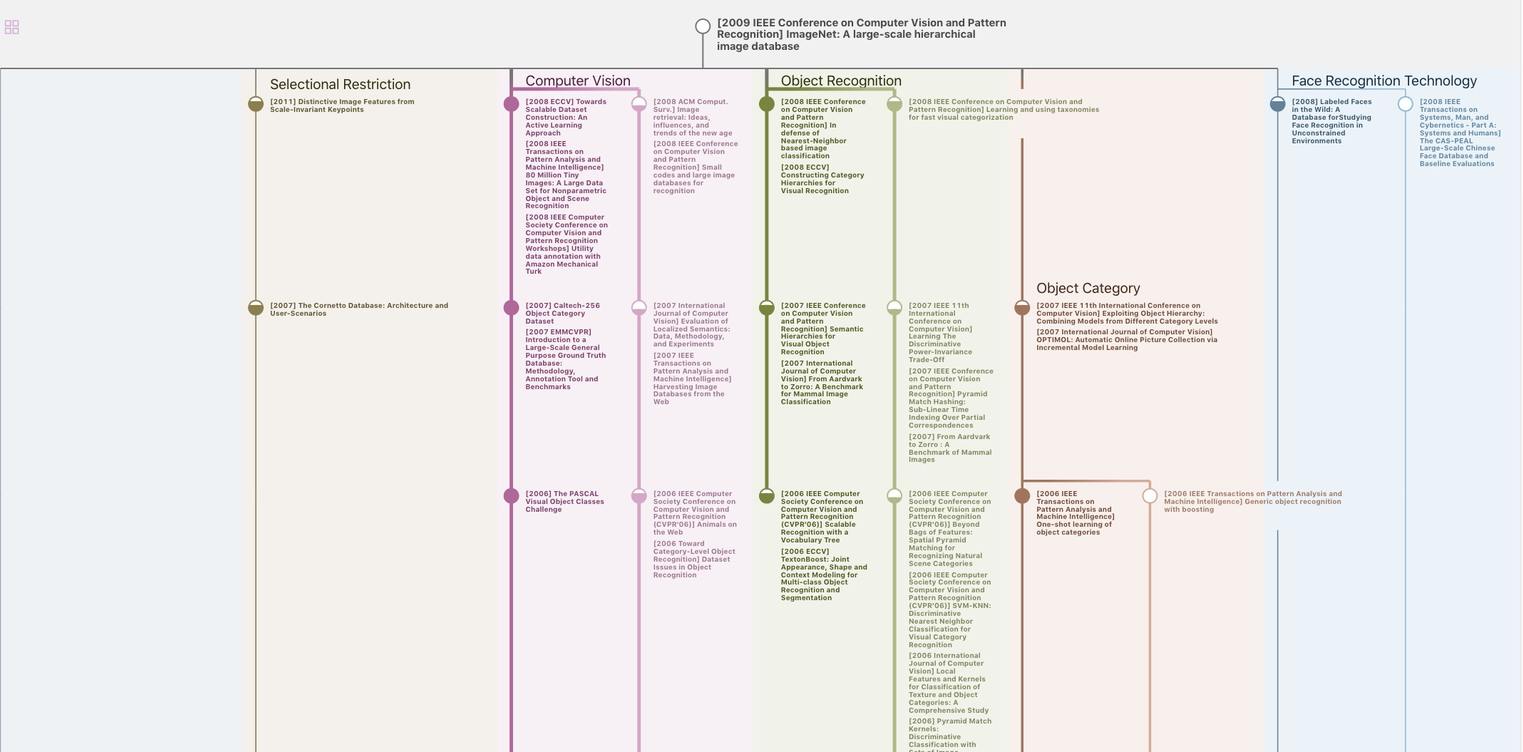
生成溯源树,研究论文发展脉络
Chat Paper
正在生成论文摘要