Detecting the invasive Lespedeza cuneata in grasslands using commercial small satellite imagery
INTERNATIONAL JOURNAL OF REMOTE SENSING(2023)
摘要
Lespedeza cuneata (L. cuneata) is an invasive legume threatening grassland ecosystems of several states in the U.S. Mapping the occurrence of invasive species is the necessary first step to control their spread. Imaging spectroscopy or hyperspectral remote sensing imagery has shown its effectiveness in detecting invasive plants. However, challenges such as limited access to hyperspectral imagery, coarse temporal resolution, high costs, and lack of standardized analysis approaches have limited their application in monitoring biological invasions. Here, we aimed to take advantage of fine spatial and temporal resolution of PlanetScope multispectral satellite data and develop an effective approach to detect L. cuneata in a naturally assembled grassland ecosystem. To achieve this objective, we first sampled a suite of foliar functional traits in the field and identified key traits that distinguish L. cuneata from native plants. We then used our airborne hyperspectral data to select vegetation indices that are associated with the key traits while focusing on those indices that are applicable to satellite-derived multispectral data. We developed seven machine learning models and a decision fusion approach using layers of vegetation indices to map L. cuneata during the growing season. Using our approach, we were able to map L. cuneata with high level of accuracy using PlanetScope multispectral imagery. Our results suggested that the optimal period for detecting L. cuneata in our site was mid-to-late growing season, with an overall classification accuracy of 80.49 +/- 5.69% (mid-August) and 82.02 +/- 4.66% (end of September), respectively. Our study contributes to developing a cost-effective solution for mapping and monitoring invasive plants, which is essential for effective control of biological invasions.
更多查看译文
关键词
Invasive plants,Lespedeza cuneata,PlanetScope imagery,machine learning,vegetation indices
AI 理解论文
溯源树
样例
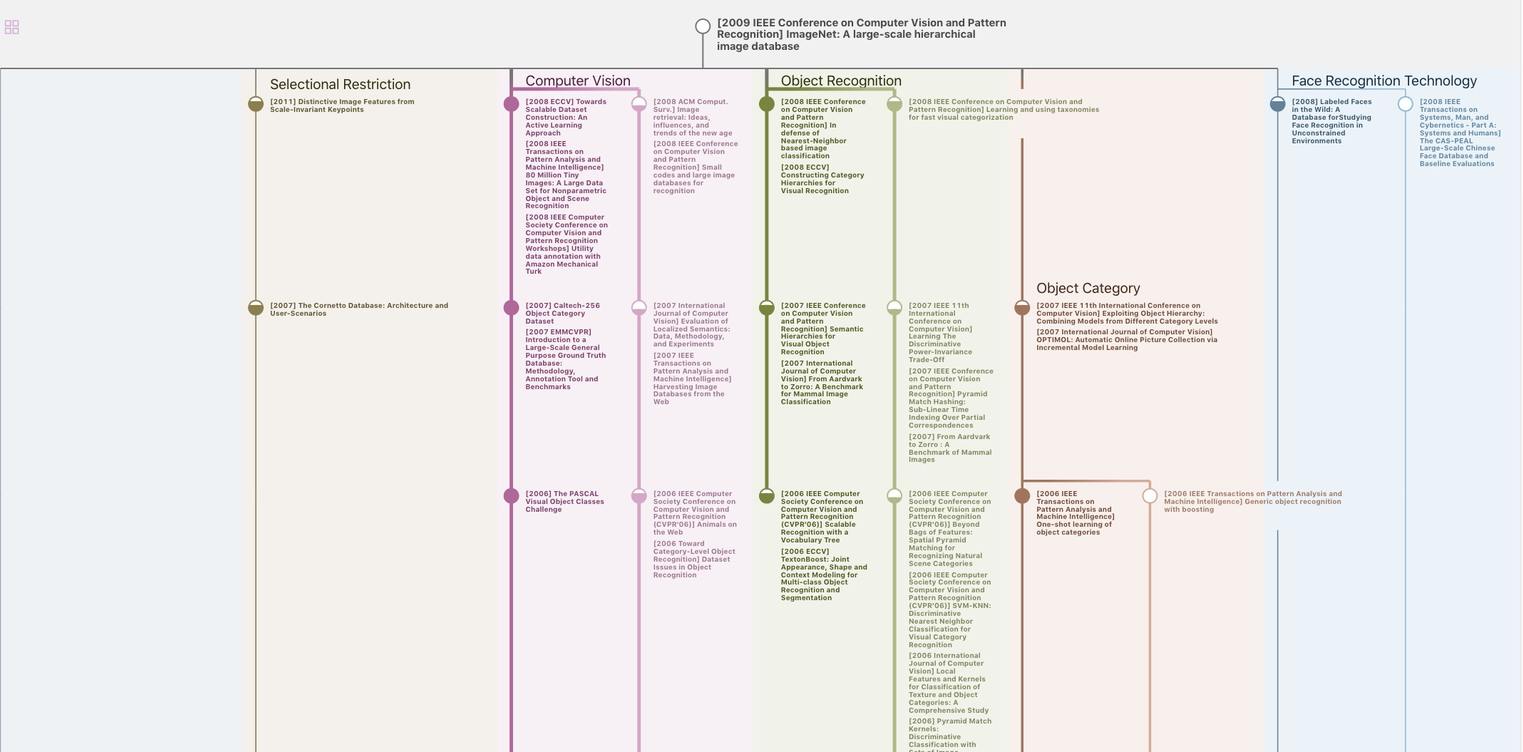
生成溯源树,研究论文发展脉络
Chat Paper
正在生成论文摘要