Accelerating design of glass substrates by machine learning using small-to-medium datasets
CERAMICS INTERNATIONAL(2024)
摘要
The demand for high-performance displays is driving the development of glass substrates with extremely low strain variation during manufacturing. To achieve the necessary balance of physical properties to resist strain, the traditional compositional design methods for glass substrates like trial-and-error and classical computational techniques should be optimized. As an alternative approach, machine learning (ML) algorithms have emerged for designing new glass compositions. In this work, we conduct ML research focused on the compositional design for high-performance glass substrates. We employ three ML algorithms i.e., Random Forest (RF), Classification And Regression Tree (CART) and k-Nearest Neighbors (k-NN) to predict five physical properties that are key to the performance of alkaline-free aluminosilicate glass substrates. By using only small-to-medium dataset sizes, our model reaches a high coefficient of determination of 0.9879. Furthermore, our model achieves an accurate generalized prediction of 50 experimental data and enables the prediction and design of glass substrate compositions with advanced comprehensive properties.
更多查看译文
关键词
Glass substrates,Machine Learning (ML),Composition design,Property prediction
AI 理解论文
溯源树
样例
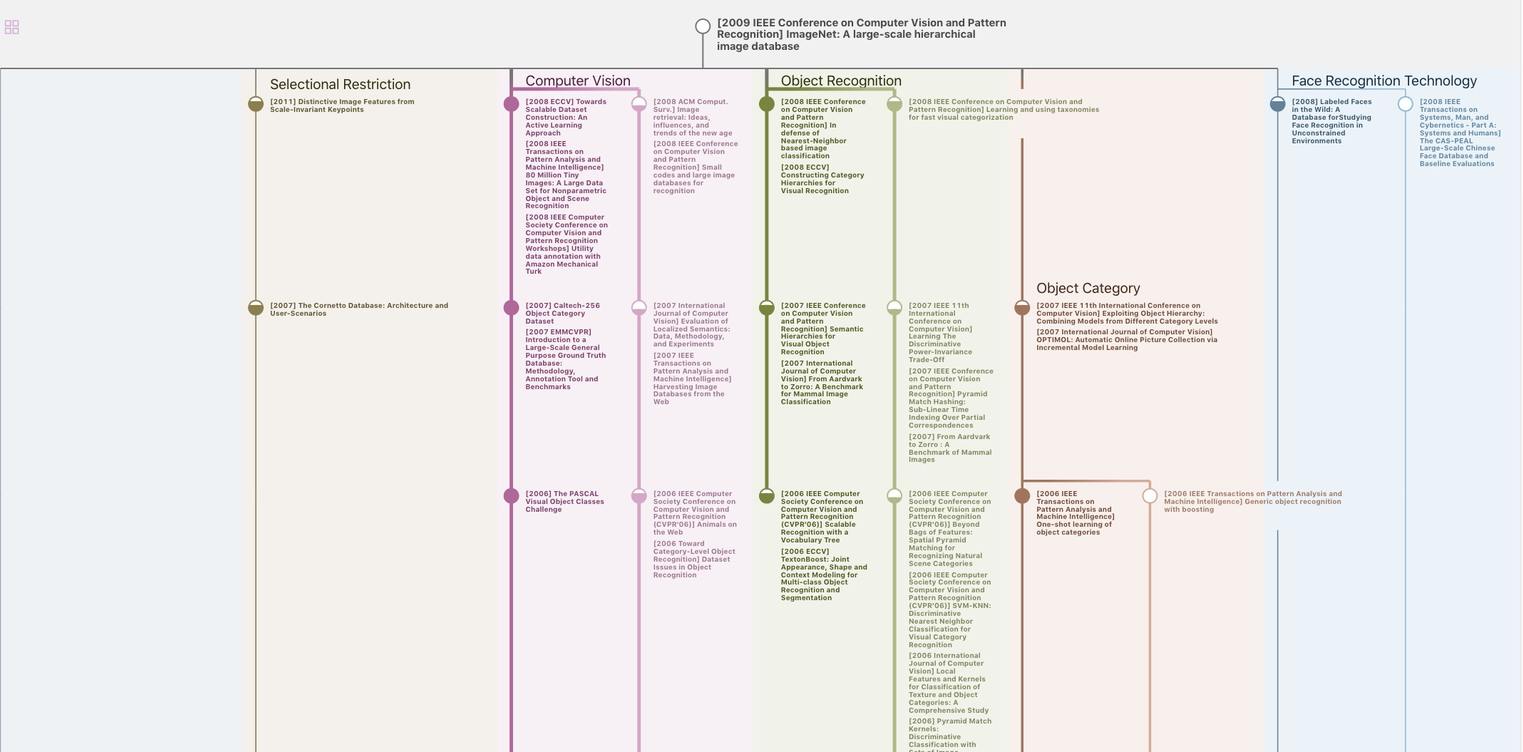
生成溯源树,研究论文发展脉络
Chat Paper
正在生成论文摘要