When ecological individual heterogeneity models and large data collide: an importance sampling approach
ANNALS OF APPLIED STATISTICS(2023)
摘要
We consider the challenges that arise when fitting ecological individual heterogeneity models to "large" data sets. In particular, we focus on dividual heterogeneity present in ecological populations within the context of capture-recapture data, although the approach is more widely applicable to more general latent variable models. Within such models the associated likelihood is expressible only as an analytically intractable integral. Common techniques for fitting such models to data include, for example, the use of numerical approximations for the integral or a Bayesian data augmentation approach. However, as the size of the data set increases (i.e., the number of individuals increases), these computational tools may become computationally infeasible. We present an efficient Bayesian model-fitting approach, whereby we initially sample from the posterior distribution of a smaller subsample of the data, before correcting this sample to obtain estimates of the posterior distribution of the full data set using an importance sampling approach. We consider several practical issues, including the subsampling mechanism, computational efficiencies (including the ability to parallelise the algorithm) and combining subsampling estimates using multiple subsampled data sets. We initially demonstrate the feasibility (and accuracy) of the approach via simulated data before considering a challenging real data set of approximately 30,000 guillemots and, using the proposed algorithm, obtain posterior estimates of the model parameters in substantially reduced computational time, compared to the standard Bayesian model-fitting approach.
更多查看译文
关键词
Capture-recapture,Cormack-Jolly-Seber model,importance sampling,individual heterogeneity,intractable likelihood,random effects
AI 理解论文
溯源树
样例
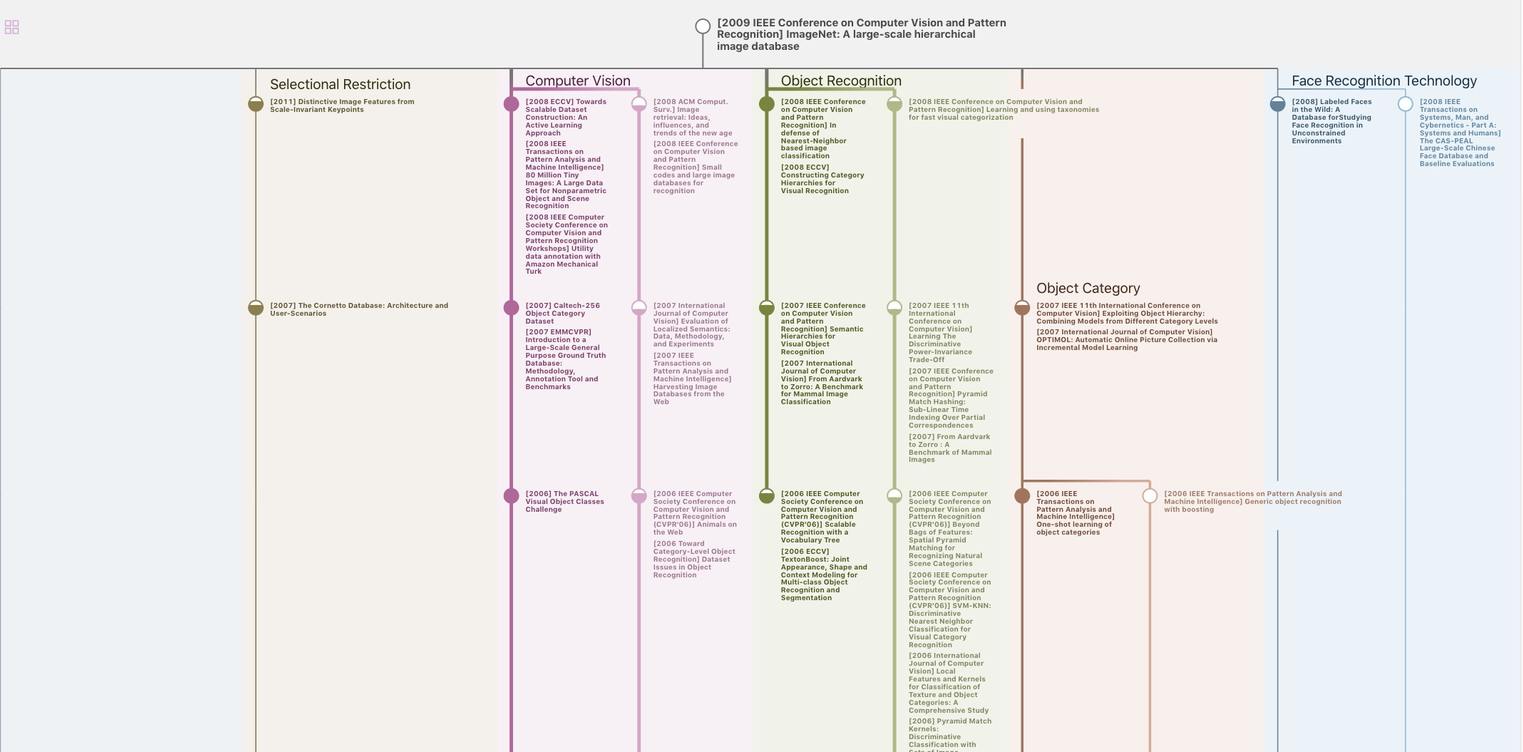
生成溯源树,研究论文发展脉络
Chat Paper
正在生成论文摘要