3D cooperative inversion of airborne magnetic and gravity gradient data using deep learning techniques
Geophysics(2024)
摘要
Using multiple geophysical methods has become a prevailing approach in numerous geophysical applications to investigate subsurface structures and parameters. These multimethod-based exploration strategies have the potential to greatly diminish uncertainties and ambiguities encountered during geophysical data analysis and interpretation. One of the applications is the cooperative inversion of airborne magnetic and gravity gradient data for the interpretation of data obtained in mineral, oil and gas, and geothermal explorations. In this paper, a unified cooperative inversion framework is designed by combining the standard separate inversions with a deep neural network (DNN), which serves as the link between different types of data. A well-trained DNN takes the separately inverted susceptibility and density models as the inputs and provides improved models that will be used as the initial models of deterministic inversions. A two-round iteration strategy is adopted to guarantee the reasonability of the recovered models and overall efficiency of the inversion. In addition, this deep-learning (DL)-based framework demonstrates excellent generalization abilities when tested on models that are entirely distinct from the training data sets. The framework can easily incorporate multiphysics without necessitating any structural changes to the network. Synthetic experiments validate that our DL-based method outperforms conventional separate inversions and cross-gradient-based joint inversion in view of the accuracy of the recovered models and inversion efficiency. Successful application to field data further verifies the effectiveness of our DL-based method.
更多查看译文
关键词
3d cooperative inversion,gravity gradient data,deep learning techniques,deep learning
AI 理解论文
溯源树
样例
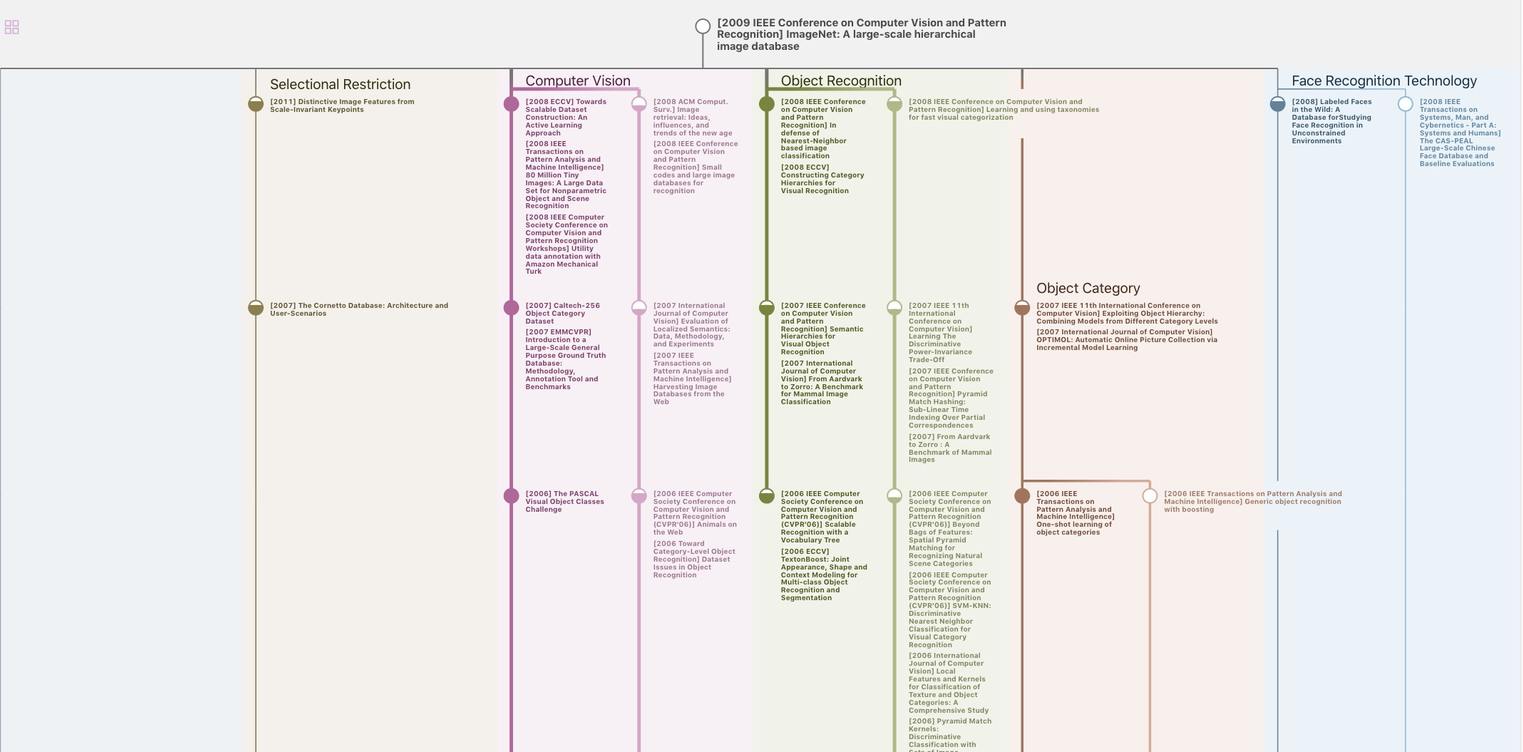
生成溯源树,研究论文发展脉络
Chat Paper
正在生成论文摘要