Physics-enhanced neural networks for half-space seismic wave modeling
Computers & Geosciences(2023)
摘要
Forward modelling of seismic waves using physics-informed neural networks (PINNs) has attracted much attention. A critical challenge is that these wave propagation problems are typically defined in large domains (i.e., half-space). However, a notable challenge arises when modeling seismic wave propagation in large domains (i.e., half-space), as PINNs may encounter the issue of "soft constraint failure". To address this problem, we propose a novel framework called physics-enhanced neural networks (PENNs) specifically designed for modeling seismic wave propagation in half-space. The method of images is incorporated to effectively implement the free stress boundary conditions of the Earth's surface, leading to successful propagation of plane waves and cylindrical waves in a half-space. We analyze the training dynamics of neural networks when solving two-dimensional (2D) wave equations from the neural tangent kernel (NTK) perspective. An adaptive training algorithm is introduced to mitigate the unbalanced gradient flow dynamics of the different components of the loss function of PINNs/PENNs. Furthermore, to tackle the complex behavior of seismic waves in layered media, a sequential training strategy is considered to enhance network scalability and solution accuracy. The results of numerical experiments demonstrate the accuracy and effectiveness of our approach.
更多查看译文
关键词
seismic wave modeling,neural networks,physics-enhanced,half-space
AI 理解论文
溯源树
样例
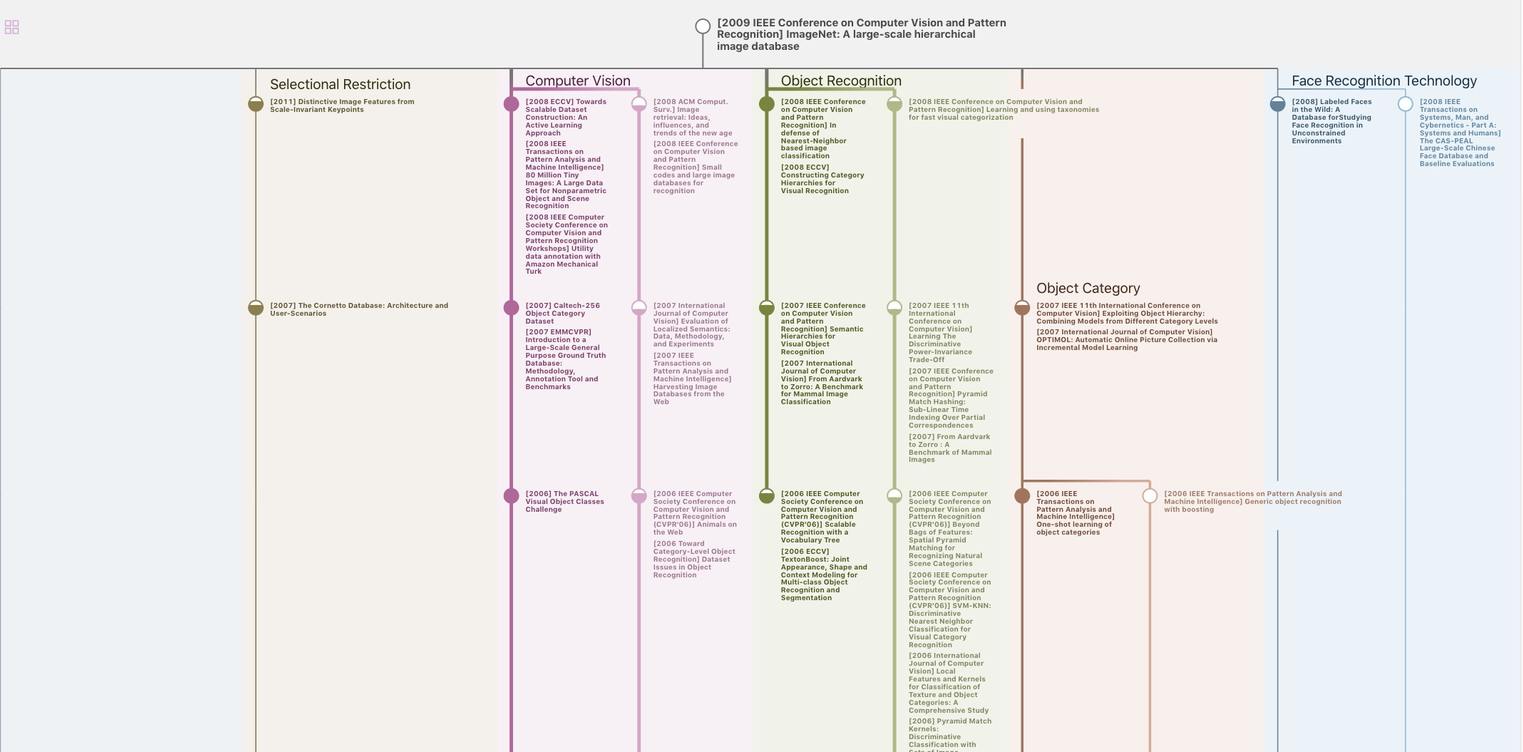
生成溯源树,研究论文发展脉络
Chat Paper
正在生成论文摘要