Open-set Face Recognition with Neural Ensemble, Maximal Entropy Loss and Feature Augmentation
arXiv (Cornell University)(2023)
摘要
Open-set face recognition refers to a scenario in which biometric systems have incomplete knowledge of all existing subjects. Therefore, they are expected to prevent face samples of unregistered subjects from being identified as previously enrolled identities. This watchlist context adds an arduous requirement that calls for the dismissal of irrelevant faces by focusing mainly on subjects of interest. As a response, this work introduces a novel method that associates an ensemble of compact neural networks with a margin-based cost function that explores additional samples. Supplementary negative samples can be obtained from external databases or synthetically built at the representation level in training time with a new mix-up feature augmentation approach. Deep neural networks pre-trained on large face datasets serve as the preliminary feature extraction module. We carry out experiments on well-known LFW and IJB-C datasets where results show that the approach is able to boost closed and open-set identification rates.
更多查看译文
关键词
face recognition,neural ensemble,maximal entropy loss,open-set
AI 理解论文
溯源树
样例
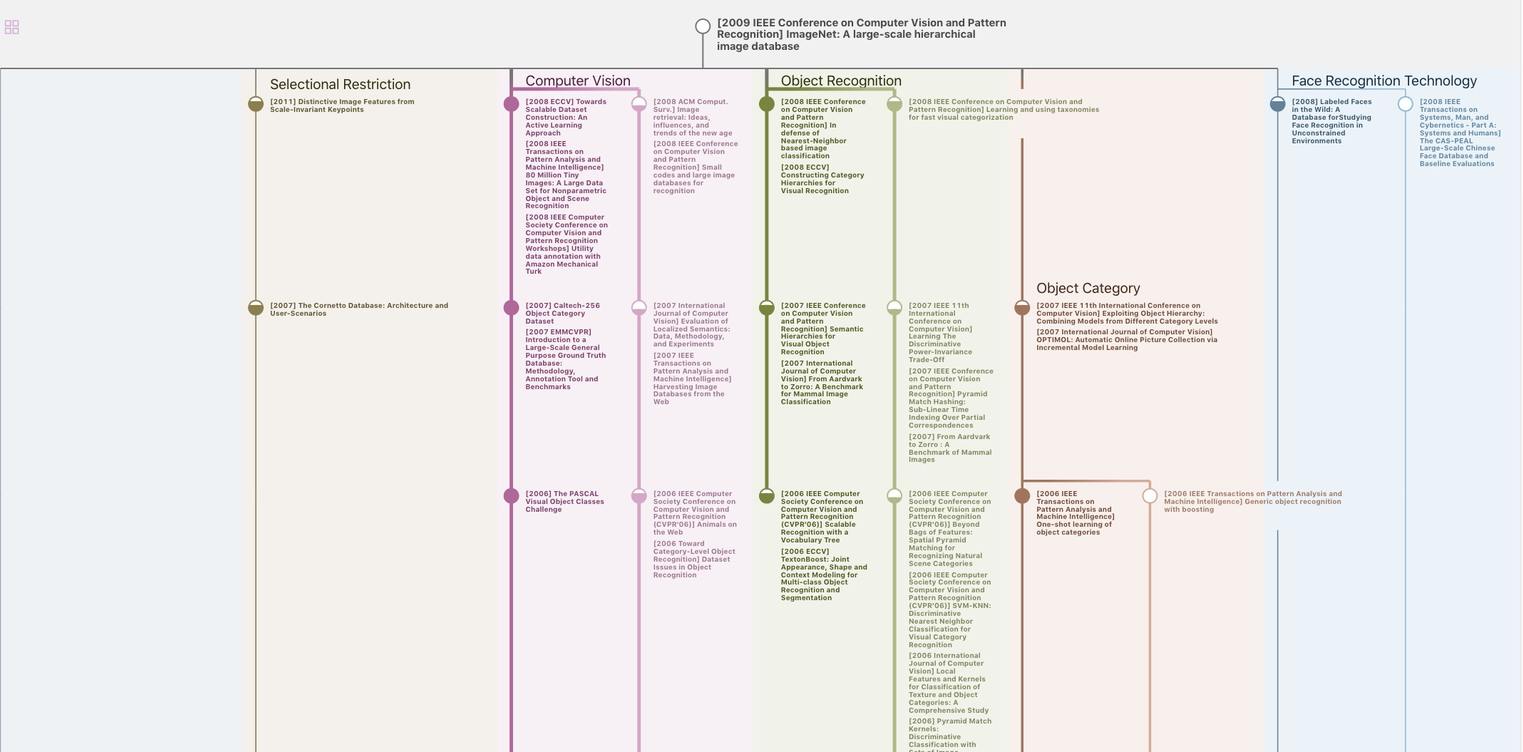
生成溯源树,研究论文发展脉络
Chat Paper
正在生成论文摘要