Feature extraction and fusion using deep convolutional neural networks for Covid-19 detection using CT and X-RAY images
World Journal Of Advanced Research and Reviews(2023)
摘要
COVID-19 represents a novel variant of the coronavirus disease, having rapidly disseminated across the globe. In recent studies pertaining to computer vision, image processing, and classification techniques, numerous methodologies have been introduced employing chest X-ray images and computerized tomography (CT) images. This investigation introduces novel fused deep features founded on CT and X-ray image classification, with the aim of detecting COVID-19 disease. The proposed approach encompasses three key phases: deep feature generation, iterative feature selection, and classification. During the feature generation phase, well-known pre-trained deep convolutional neural networks, namely DenseNet201, MobileNetV2, ResNet18, ResNet50, ResNet101, VGG16, and VGG19, were leveraged. Each network model generated 1000 features, which were subsequently fused, culminating in the acquisition of a final feature vector comprising 7000 dimensions. In order to distill the most pertinent information, the ReliefF and iterative maximum relevance minimum redundancy (RFImRMR) feature selection techniques were employed in the generation of the ultimate feature vector. To evaluate the performance of the proposed method, publicly available datasets of CT images and X-ray images were employed. Notably, the suggested deep learning approach attained accuracy rates of 99.33% and 93.10% for COVID-19 detection using CT and X-ray images, respectively. These achieved results serve as compelling evidence substantiating the efficacy of the proposed fused deep features and RFImRMR-based COVID-19 detection.
更多查看译文
关键词
deep convolutional neural networks,detection,x-ray
AI 理解论文
溯源树
样例
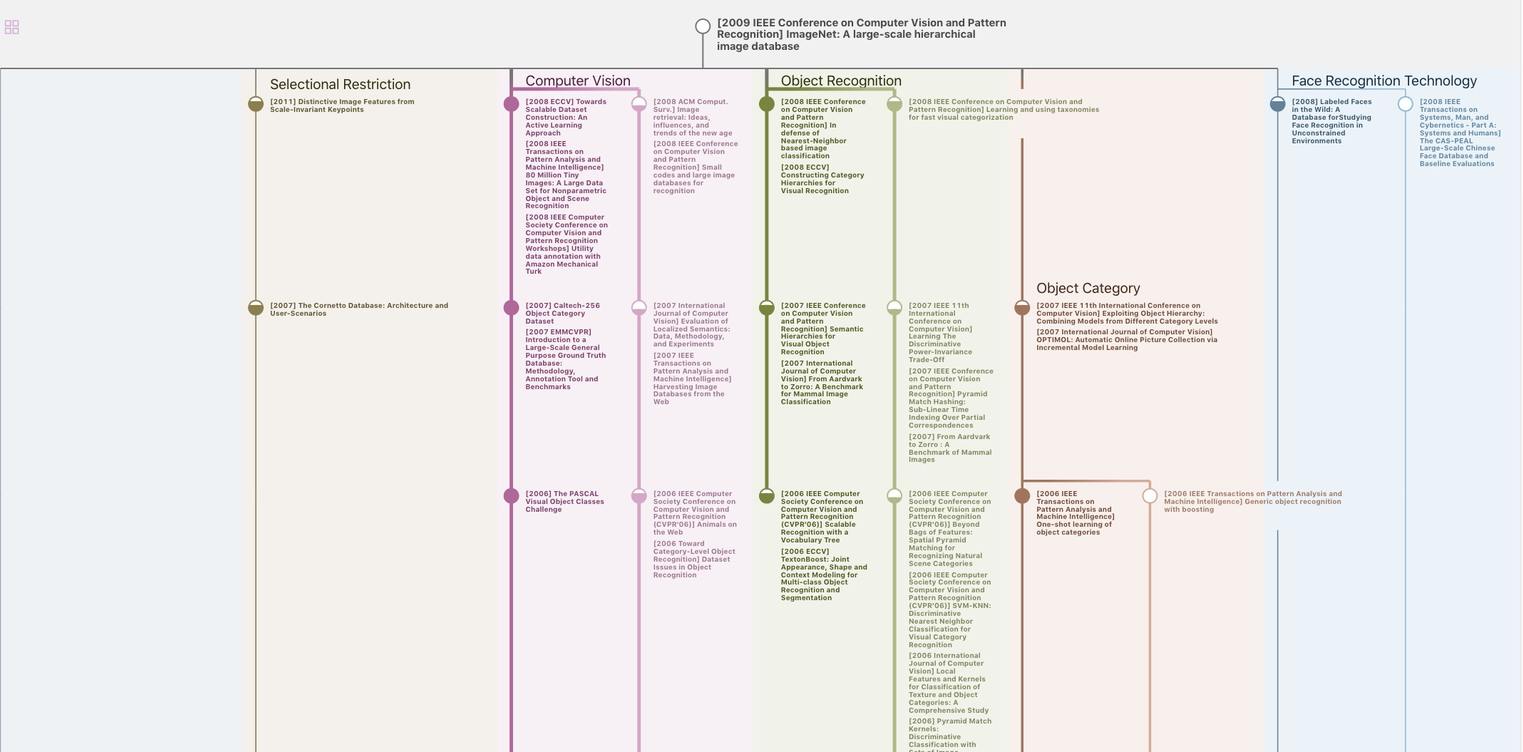
生成溯源树,研究论文发展脉络
Chat Paper
正在生成论文摘要