Semi-Supervised Single-Image Dehazing Network via Disentangled Meta-Knowledge
IEEE TRANSACTIONS ON MULTIMEDIA(2024)
Abstract
Captured outdoor scene images are easily affected by haze. Most image dehazing methods have limited generalization capabilities for real-world hazy images owing to the complexities of real-world environments and domain gaps in the training datasets. This article proposes a semi-supervised single-image dehazing network based on disentangled meta-knowledge. The symmetric and heterogeneous design of the disentangled network is conducive to the separation of the content and mask features of hazy images and these features are used as meta-knowledge to guide feature fusion in the dehazing network. Moreover, functions describing constant-color and disentangled-reconstruction-checking losses are designed to ensure the subjective qualities of the generated dehazed images. The results of extensive experiments conducted on synthetic datasets and real-world images indicate that the proposed algorithm outperforms state-of-the-art single-image dehazing algorithms. In addition, the algorithm effectively improves the performance of object-detection tasks.
MoreTranslated text
Key words
Atmospheric modeling,Training,Metalearning,Image reconstruction,Task analysis,Prediction algorithms,Synthetic data,Disentangled representations,meta-learning,semi-supervised learning,single-image dehazing
AI Read Science
Must-Reading Tree
Example
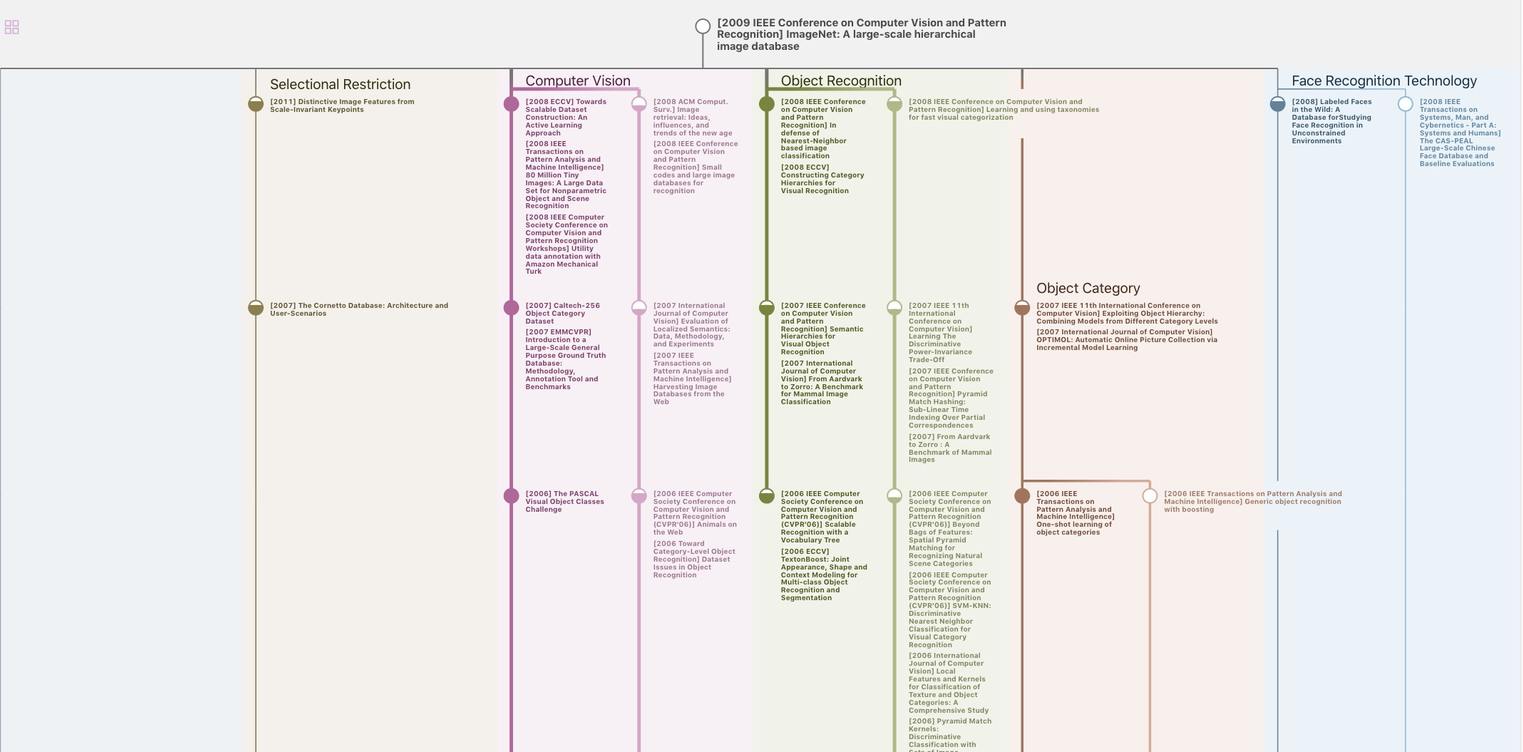
Generate MRT to find the research sequence of this paper
Chat Paper
Summary is being generated by the instructions you defined