Generalizing Projected Gradient Descent for Deep-Learning-Aided Massive MIMO Detection.
IEEE Trans. Wirel. Commun.(2024)
摘要
In this paper, the projected-gradient-descent (PGD) -based detector for massive MIMO system, which consists of two basic operations — projection and gradient descent (GD), is studied to achieve the performance improvement. Since the projection and GD step have different loss functions, necessary compromise has to be made to balance them during iterations. For this reason, the generalized PGD (GPGD) method is proposed with flexible choices of projection and GD. Different from performing projection and GD alternatively, we show that implementing projection after every multiple GD steps is a better solution. Meanwhile, the step-size of GD is also investigated for convergence efficiency. After that, by unfolding this proposed GPGD method with deep neural networks (DNN), the self-corrected auto-detector (SAD) is established to achieve better decoding performance, where enhancement by attention mechanism and extension by another iterative method are also given for performance improvement and efficiency.
更多查看译文
关键词
Massive MIMO detection,projected gradient descent,denoising auto-encoder,attention mechanism
AI 理解论文
溯源树
样例
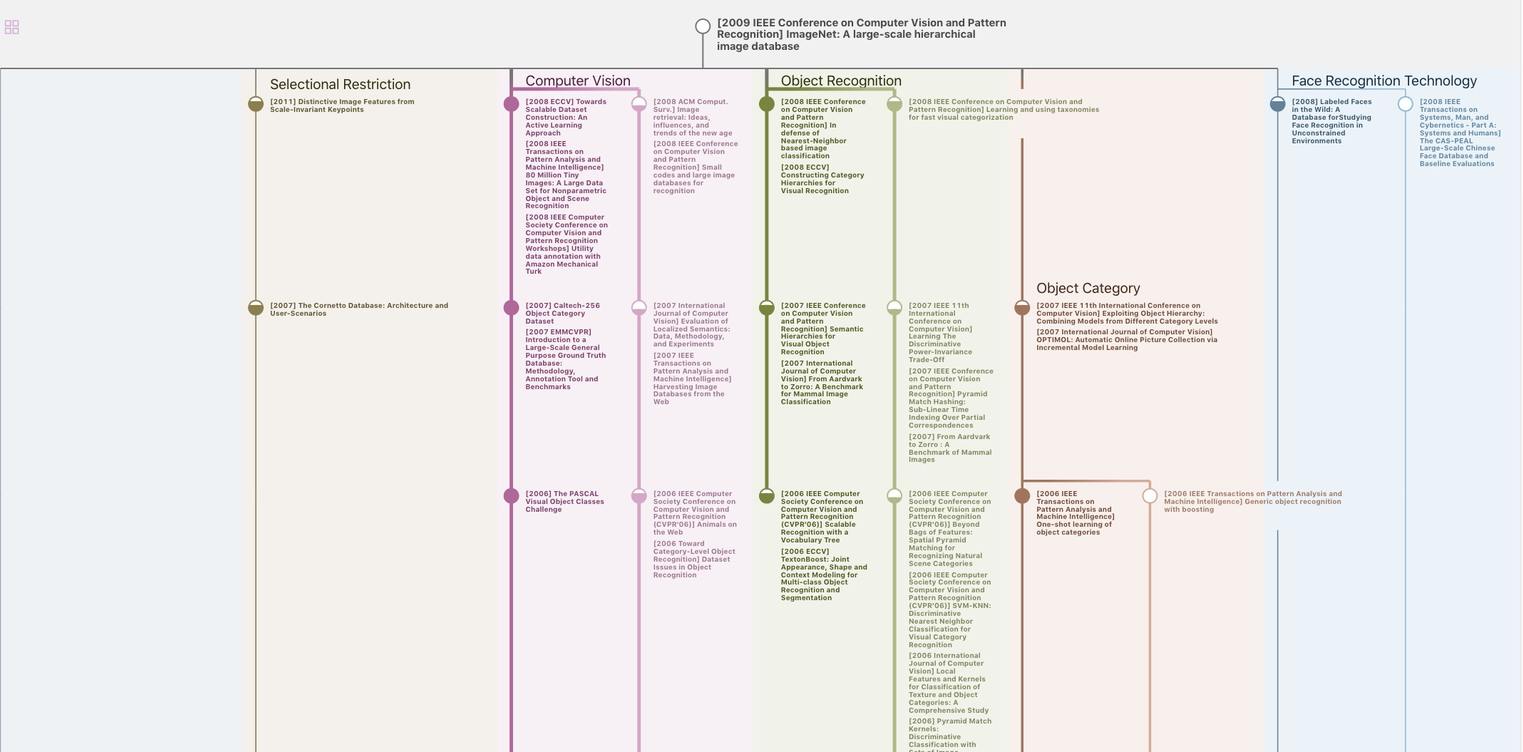
生成溯源树,研究论文发展脉络
Chat Paper
正在生成论文摘要