Transfer Learning-based State of Charge and State of Health Estimation for Li-ion Batteries: A Review
IEEE Transactions on Transportation Electrification(2023)
摘要
State of charge (SOC) and state of health (SOH) estimation play a vital role in battery management systems (BMS). Accurate and robust state estimation can prevent Li-ion batteries (LIBs) from overcharging and undercharging. In recent years, many methods have been utilized for accurate state estimation. Among these methods, data-driven methods show overwhelming effectiveness. However, data-driven methods are also facing several severe challenges such as data distribution discrepancy and data insufficiency. To tackle these problems, transfer learning (TL) has been leveraged in the community recently. TL-based methods are promising since they can be generalized to various working conditions and are able to alleviate the data distribution discrepancy issue. However, transfer learning was studied in the machine learning community and it is not familiar to the power electronics researchers. In this paper, we provide a brief review to mitigate the domain gap. Specifically, recent TL-based state estimation methods are reviewed and divided into three categories: fine-tuning methods, metric-based methods and adversarial adaptation methods. The model structures, theories and key techniques of these methods are discussed in detail. Moreover, it is noticed that there are no uniform evaluation criteria in previous literature. By analyzing the required properties of estimation models, new standards for performance evaluation criteria, test datasets and experiment settings are discussed. Extensive experiments are performed according to the newly proposed standards. At last, we propose several possible directions for future works.
更多查看译文
关键词
Li-ion batteries,state of charge estimation,state of health estimation,domain adaptation,unsupervised learning,transfer learning
AI 理解论文
溯源树
样例
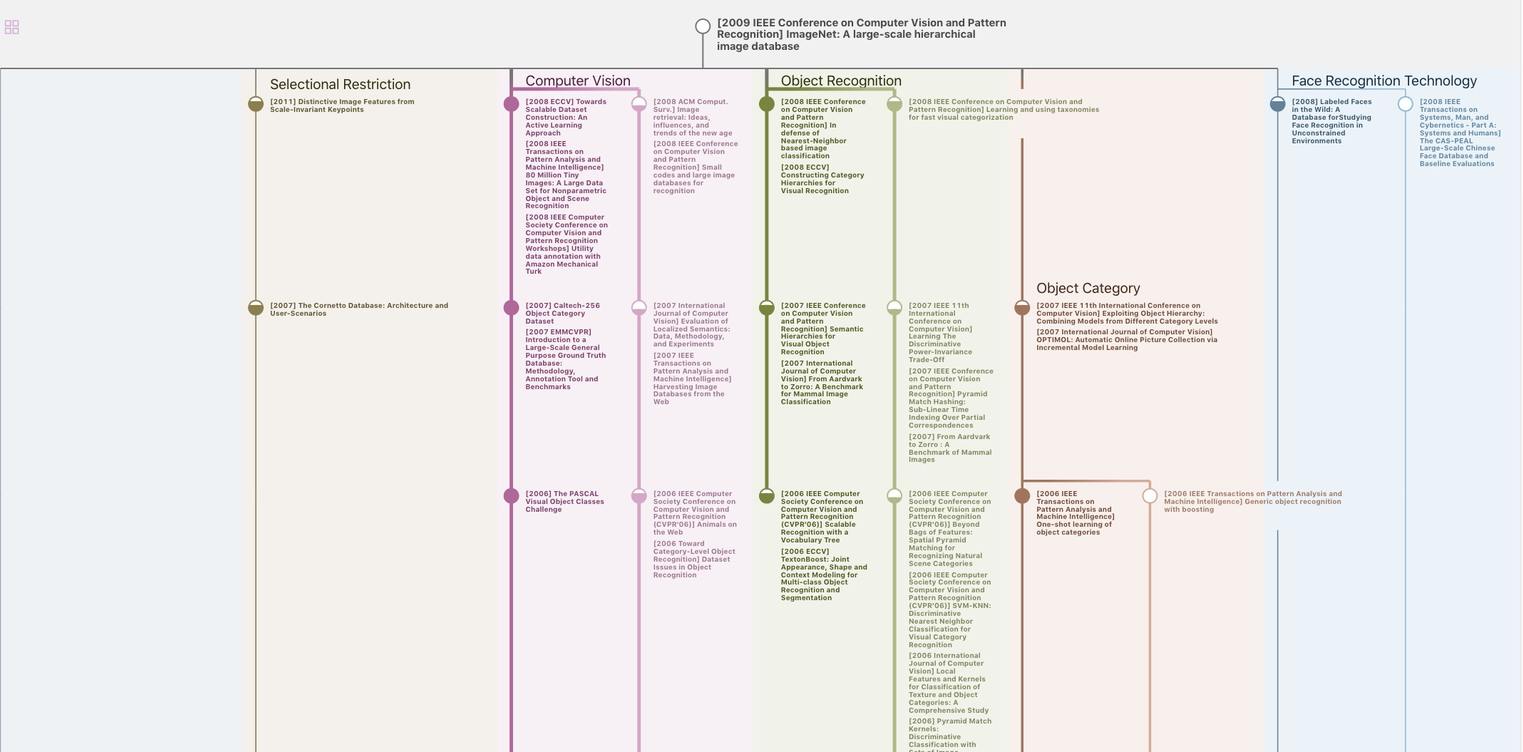
生成溯源树,研究论文发展脉络
Chat Paper
正在生成论文摘要