Deep Image Prior-Based PET Reconstruction From Partial Data
IEEE TRANSACTIONS ON RADIATION AND PLASMA MEDICAL SCIENCES(2024)
Abstract
In this article, we propose an unsupervised deep learning method for positron emission tomography (PET) reconstruction from incomplete data. This method utilizes the so-called deep image prior (DIP) as an untrained deep convolutional neural network (CNN) to generate object reconstructions. The main idea is to reparameterize the image reconstruction problem as a neural network optimization problem. We show that the proposed method effectively addresses the incomplete data reconstruction problem, which otherwise degrades the image resolution and quality of standard reconstruction algorithms. Meanwhile, the proposed method does not require any pretraining procedures, i.e., it is not biased toward any particular dataset. Hence, it has the potential to be used in clinical situations, where training data would be infeasible or prohibitively expensive. The performance of the proposed approach is evaluated with noisy synthetic data based on the Shepp-Logan and brainweb phantoms, and clinical naive rat data. In addition, robustness studies of the approach with respect to regularization parameters are also carried out. We showcase that the proposed method considerably outperforms the state-of-the-art methods, leading to flexible reconstruction from incomplete PET data.
MoreTranslated text
Key words
Contourlet transform,deep image prior (DIP),incomplete data,positron emission tomography (PET),unsupervised learning
AI Read Science
Must-Reading Tree
Example
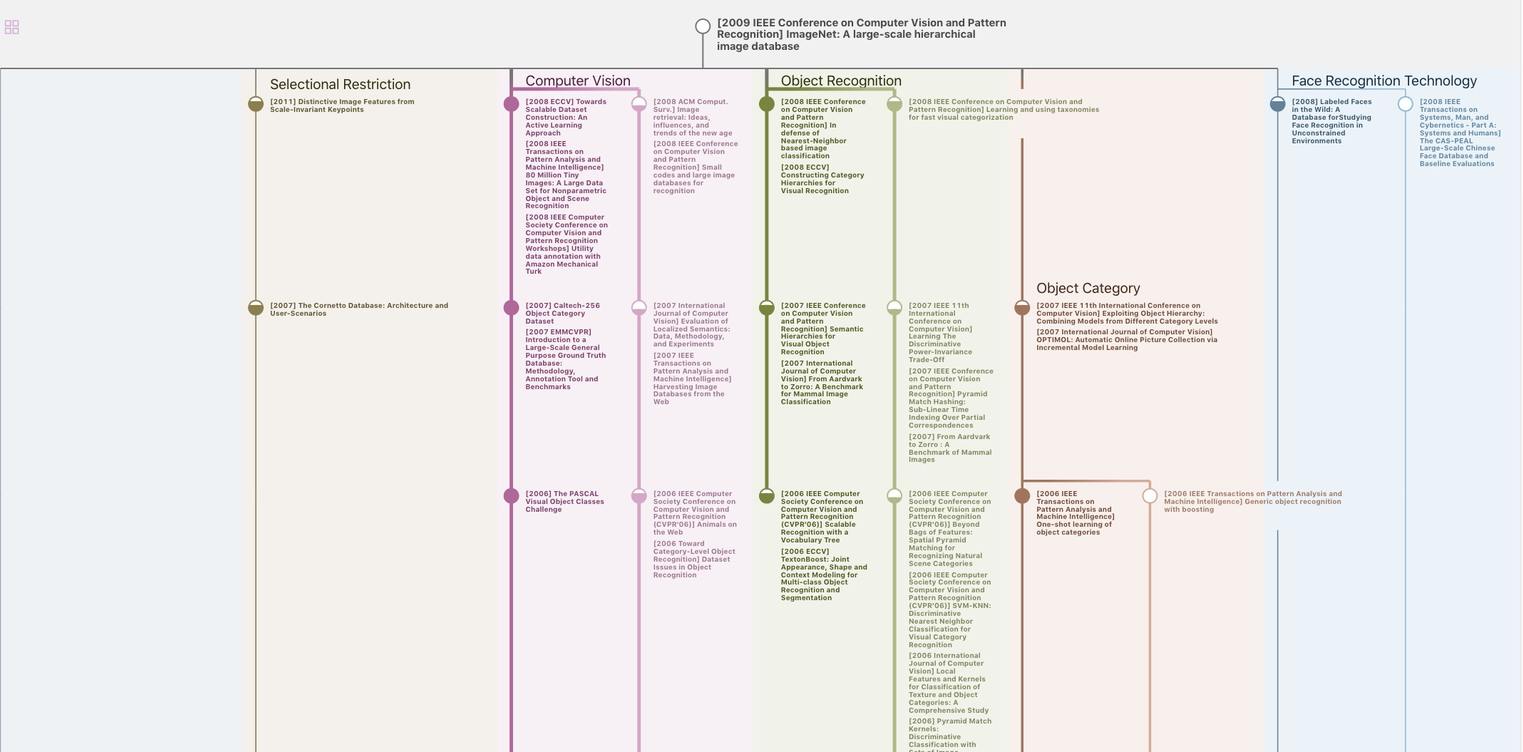
Generate MRT to find the research sequence of this paper
Chat Paper
Summary is being generated by the instructions you defined