Evaluation of multiple imputation to address intended and unintended missing data in case-cohort studies with a binary endpoint
Research Square (Research Square)(2023)
摘要
Abstract Background: Case-cohort studies are conducted within cohort studies, with the defining feature that collection of exposure data is limited to a subset of the cohort, leading to a large proportion of missing data by design. Standard analysis uses inverse probability weighting (IPW) to address this intended missing data, but little research has been conducted into how best to perform analysis when there is also unintended missingness. Multiple imputation (MI) has become a default standard for handling unintended missingness and is typically used in combination with IPW to handle the intended missingness due to the case-control sampling. Alternatively, MI could be used to handle both the intended and unintended missingness. While the performance of an MI-only approach has been investigated in the context of a case-cohort study with a time-to-event outcome, it is unclear how this approach performs with a binary outcome. Methods: We conducted a simulation study to assess and compare the performance of approaches using only MI, only IPW, and a combination of MI and IPW, for handling intended and unintended missingness in the case-cohort setting. We also applied the approaches to a case study. Results: Our results show that the combined approach is approximately unbiased for estimation of the exposure effect when the sample size is large, and was the least biased with small sample sizes, while MI-only and IPW-only exhibited larger biases in both sample size settings. Conclusions: These findings suggest that a combined MI/IPW approach should be preferred to handle intended and unintended missing data in case-cohort studies with binary outcomes.
更多查看译文
关键词
multiple imputation,case-cohort
AI 理解论文
溯源树
样例
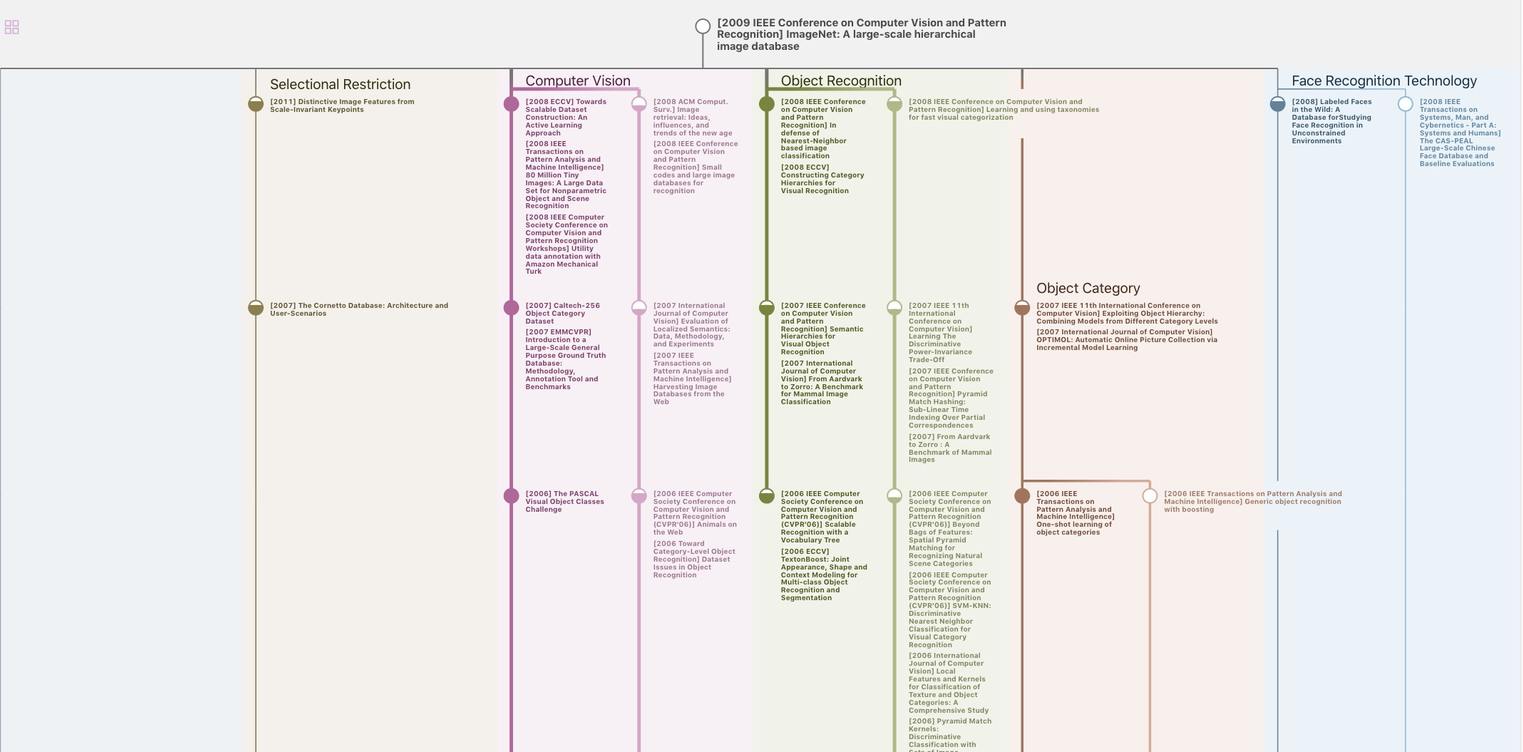
生成溯源树,研究论文发展脉络
Chat Paper
正在生成论文摘要