Deep Autoencoders with Value-at-Risk Thresholding for Unsupervised Anomaly Detection
arXiv (Cornell University)(2019)
摘要
Many real-world monitoring and surveillance applications require non-trivial anomaly detection to be run in the streaming model. We consider an incremental-learning approach, wherein a deep-autoencoding (DAE) model of what is normal is trained and used to detect anomalies at the same time. In the detection of anomalies, we utilise a novel thresholding mechanism, based on value at risk (VaR). We compare the resulting convolutional neural network (CNN) against a number of subspace methods, and present results on changedetection net.
更多查看译文
关键词
anomaly,unsupervised
AI 理解论文
溯源树
样例
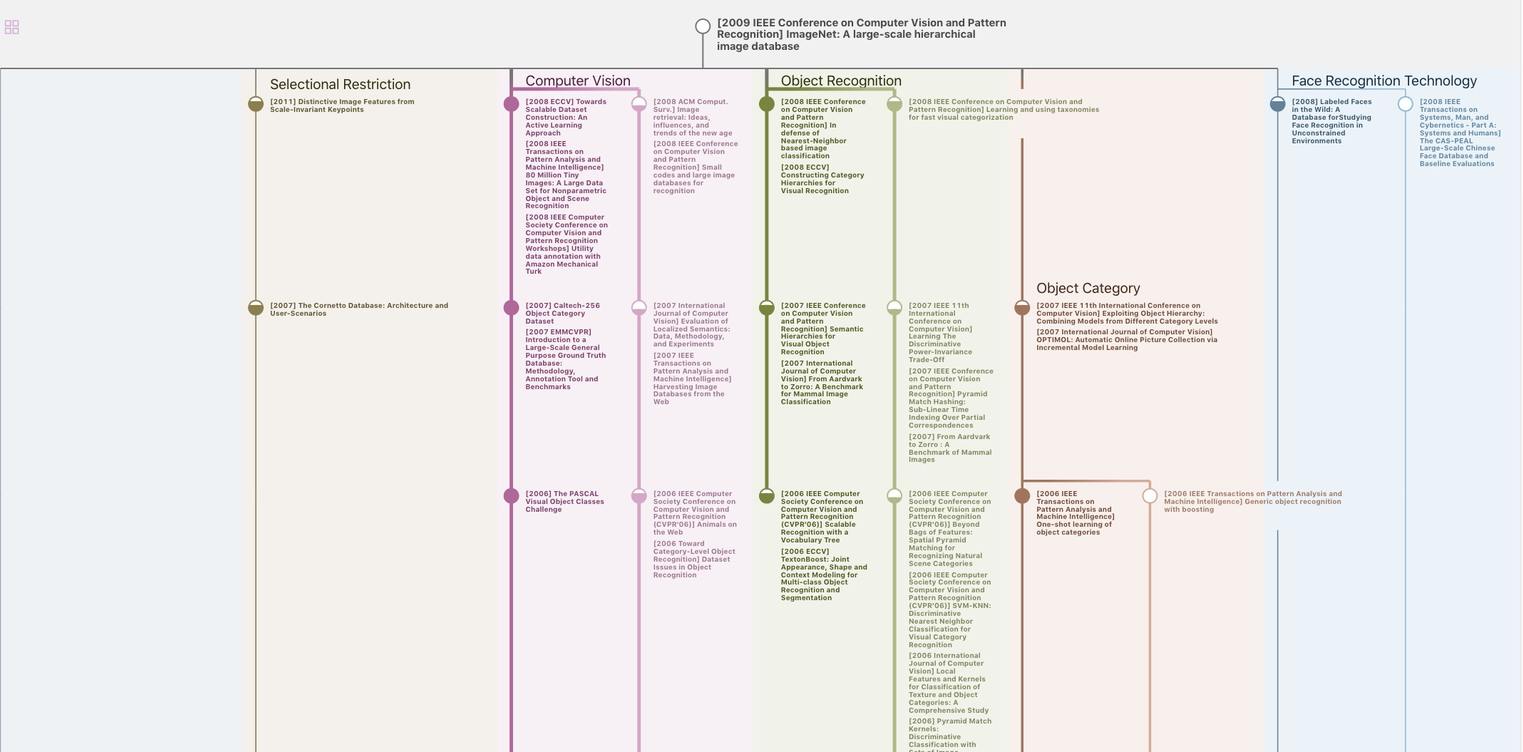
生成溯源树,研究论文发展脉络
Chat Paper
正在生成论文摘要