Synthesis and evaluation of hybrid sulfonamide-chalcones with potential antileishmanial activity
ARCHIV DER PHARMAZIE(2024)
Abstract
Leishmaniasis is an emerging tropical infectious disease caused by a protozoan parasite of the genus Leishmania. In this work, the molecular hybridization between a trimethoxy chalcone and a sulfonamide group was used to generate a series of sulfonamide-chalcones. A series of eight sulfonamide-chalcone hybrids were made with good yields (up to 95%). These sulfonamide-chalcones were tested against promastigotes of Leishmania amazonensis and cytotoxicity against mouse macrophages, which showed good antileishmanial activity with IC50 = 1.72-3.19 mu M. Three of them (10c, 10g, and 10h) were also highly active against intracellular amastigotes and had a good selectivity index (SI > 9). Thus, those three compounds were docked in the cytosolic tryparedoxin peroxidase (cTXNPx) enzyme of the parasite, and molecular dynamics simulations were carried out. This enzyme was selected as a target protein for the sulfonamide-chalcones due to the fact of the anterior report, which identified a strong and stable interaction between the chalcone NAT22 (6) and the cTXNPx. In addition, a prediction of the drug-likeness, and the pharmacokinetic profile of all compounds were made, demonstrating a good profile of those chalcones.
MoreTranslated text
Key words
amastigote,Leishmania amazonensis,molecular docking,molecular dynamics,promastigote
AI Read Science
Must-Reading Tree
Example
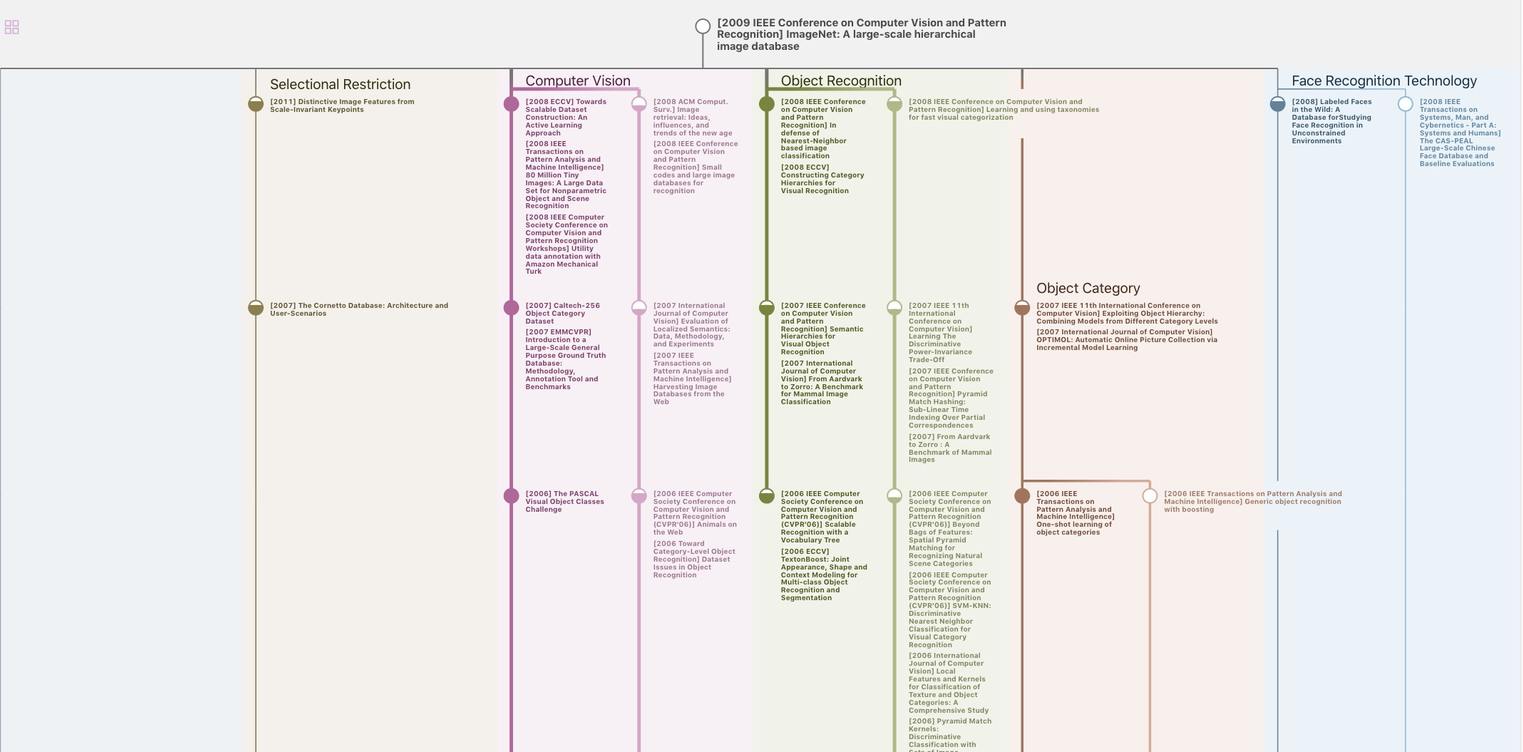
Generate MRT to find the research sequence of this paper
Chat Paper
Summary is being generated by the instructions you defined