Unsupervised Sparse-View Backprojection via Convolutional and Spatial Transformer Networks
Lecture Notes in Computer Science(2023)
摘要
Imaging technologies heavily rely on tomographic reconstruction, which involves solving a multidimensional inverse problem given a limited number of projections. Building upon our prior research [14], we have ascertained that the integration of the predicted source space derived from electroencephalography (EEG) and functional magnetic resonance imaging (fMRI) can be effectively approached as a backprojection problem involving sensor non-uniformity. Although backprojection is a commonly used algorithm for tomographic reconstruction, it often produces subpar image reconstructions when the projection angles are sparse or the sensor characteristics are non-uniform. To address this issue, various deep learning-based algorithms have been developed to solve the inverse problem and reconstruct images using a reduced number of projections. However, these algorithms typically require ground-truth examples, i.e., reconstructed images, to achieve satisfactory performance. In this paper, we present an unsupervised sparse-view backprojection algorithm that does not rely on ground-truth examples. Our algorithm comprises two modules within a generator-projector framework: a convolutional neural network and a spatial transformer network. We evaluate the effectiveness of our algorithm using computed tomography (CT) images of the human chest. The results demonstrate that our algorithm outperforms filtered backprojection significantly in scenarios with very sparse projection angles or varying sensor characteristics for different angles. Our proposed approach holds practical implications for medical imaging and other imaging modalities (e.g., radar) where sparse and/or non-uniform projections may arise due to time or sampling constraints.
更多查看译文
关键词
convolutional,spatial,sparse-view
AI 理解论文
溯源树
样例
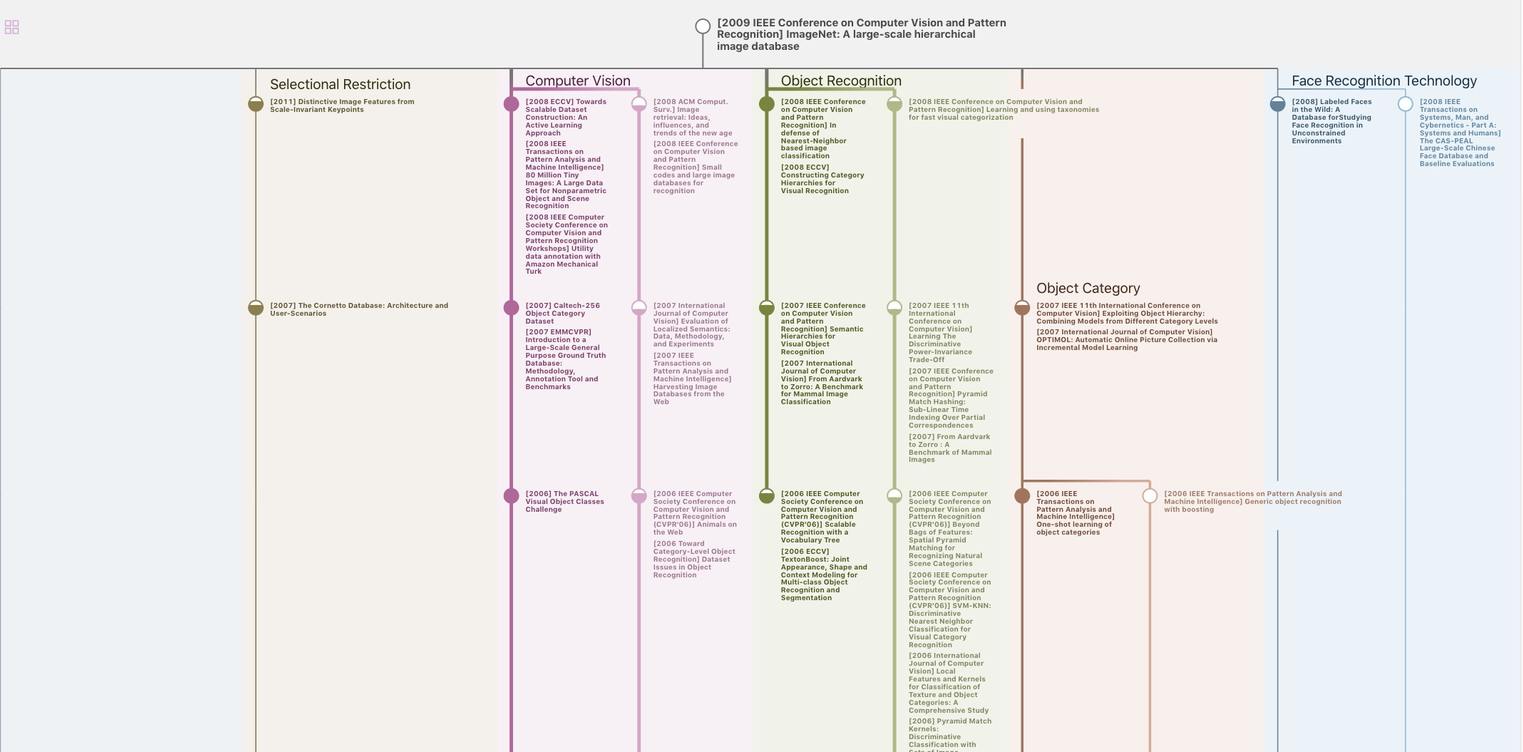
生成溯源树,研究论文发展脉络
Chat Paper
正在生成论文摘要