KGRACDA: A Model Based on Knowledge Graph from Recursion and Attention Aggregation for CircRNA-disease Association Prediction
biorxiv(2023)
摘要
CircRNA has been found to exhibit with human diseases, rendering the investigation of the circRNA-disease association (CDA) of significant importance. Deep learning provides a novel avenue for predicting CDA, however, due to the limited availability of comprehensive CDA datasets, most existing methods operate by directly reasoning over triplets of embedded entities and relations, neglecting the explicit capture local depth information. In this study, we propose a novel approach called Knowledge Graph-Enhanced Recursive Aggregation for CDA (KGRACDA), which enables the querying of explicit local structures surrounding triplets, thereby serving as crucial evidence for predicting CDA. First, we construct a comprehensive CDA knowledge graph utilizing a large-scale, multi-source heterogeneous dataset. Subsequently, we develop a graph neural network using recursive techniques and employ attention aggregation to generate CDA prediction scores. Experimental results demonstrate that KGRACDA surpasses other state-of-the-art methods by explicitly capturing local depth information related to CDA. To improve the convenience of CDA prediction, we have updated an interactive web platform named HNRBase v2.0, which provides rich visualization of circRNA data, and allows users to easily download data and predict CDA using KGRACDA. The code and datasets are publicly available at .
### Competing Interest Statement
The authors have declared no competing interest.
更多查看译文
AI 理解论文
溯源树
样例
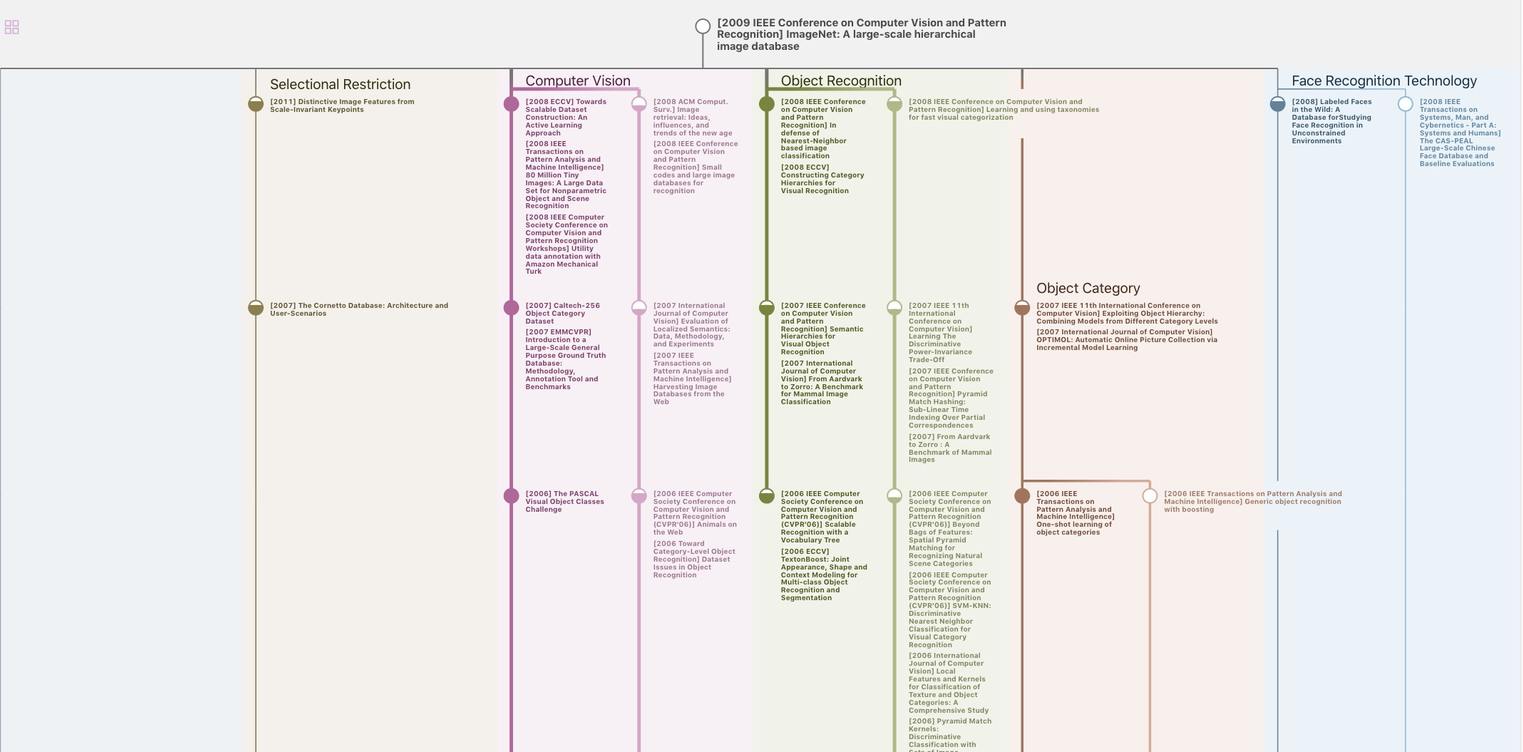
生成溯源树,研究论文发展脉络
Chat Paper
正在生成论文摘要