Robust Autoencoders for Collective Corruption Removal
arXiv (Cornell University)(2023)
摘要
Robust PCA is a standard tool for learning a linear subspace in the presence of sparse corruption or rare outliers. What about robustly learning manifolds that are more realistic models for natural data, such as images? There have been several recent attempts to generalize robust PCA to manifold settings. In this paper, we propose $\ell_1$- and scaling-invariant $\ell_1/\ell_2$-robust autoencoders based on a surprisingly compact formulation built on the intuition that deep autoencoders perform manifold learning. We demonstrate on several standard image datasets that the proposed formulation significantly outperforms all previous methods in collectively removing sparse corruption, without clean images for training. Moreover, we also show that the learned manifold structures can be generalized to unseen data samples effectively.
更多查看译文
关键词
robust autoencoders,collective corruption removal
AI 理解论文
溯源树
样例
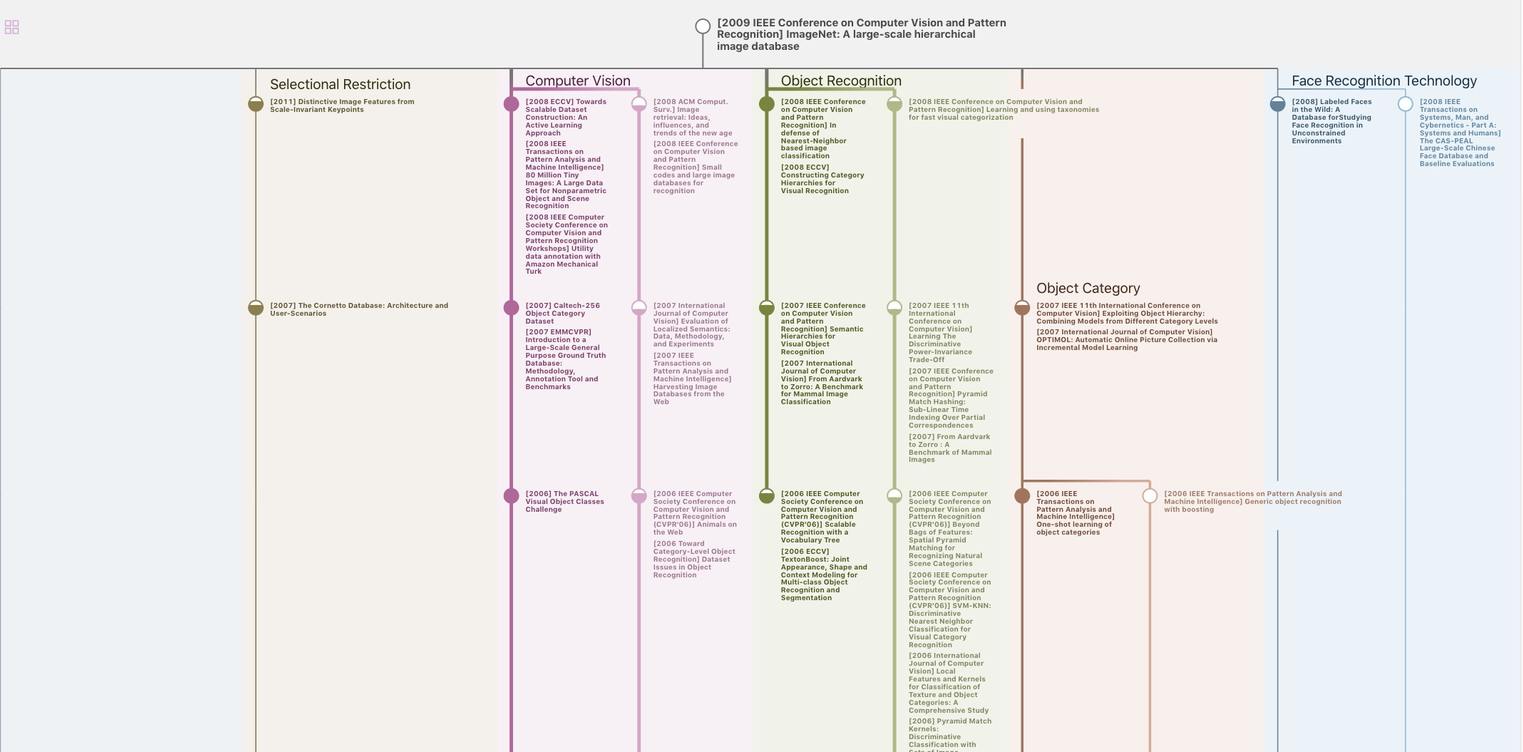
生成溯源树,研究论文发展脉络
Chat Paper
正在生成论文摘要