Self-Supervised Approaches to the Classification of Spectra: Application to Phase Transitions in X-ray Diffraction Data
Research Square (Research Square)(2023)
Abstract
Abstract The ability to detect interesting events is instrumental to effectively steer experiments and maximize their scientific efficiency. To address this, here we introduce and validate three frameworks based on self-supervised learning which are capable of classifying 1D spectral data using a limited amount of labeled data. In particular, in this work we focus on the identification of phase transitions in samples investigated by x-ray diffraction. We demonstrate that the three frameworks, based either on relational reasoning, contrastive learning, or a combination of the two, are capable of accurately identifying phase transitions. Furthermore, we discuss in detail the selection of data augmentations, crucial to ensure that scientifically meaningful information is retained.
MoreTranslated text
Key words
phase transitions,spectra,diffraction,classification,self-supervised,x-ray
AI Read Science
Must-Reading Tree
Example
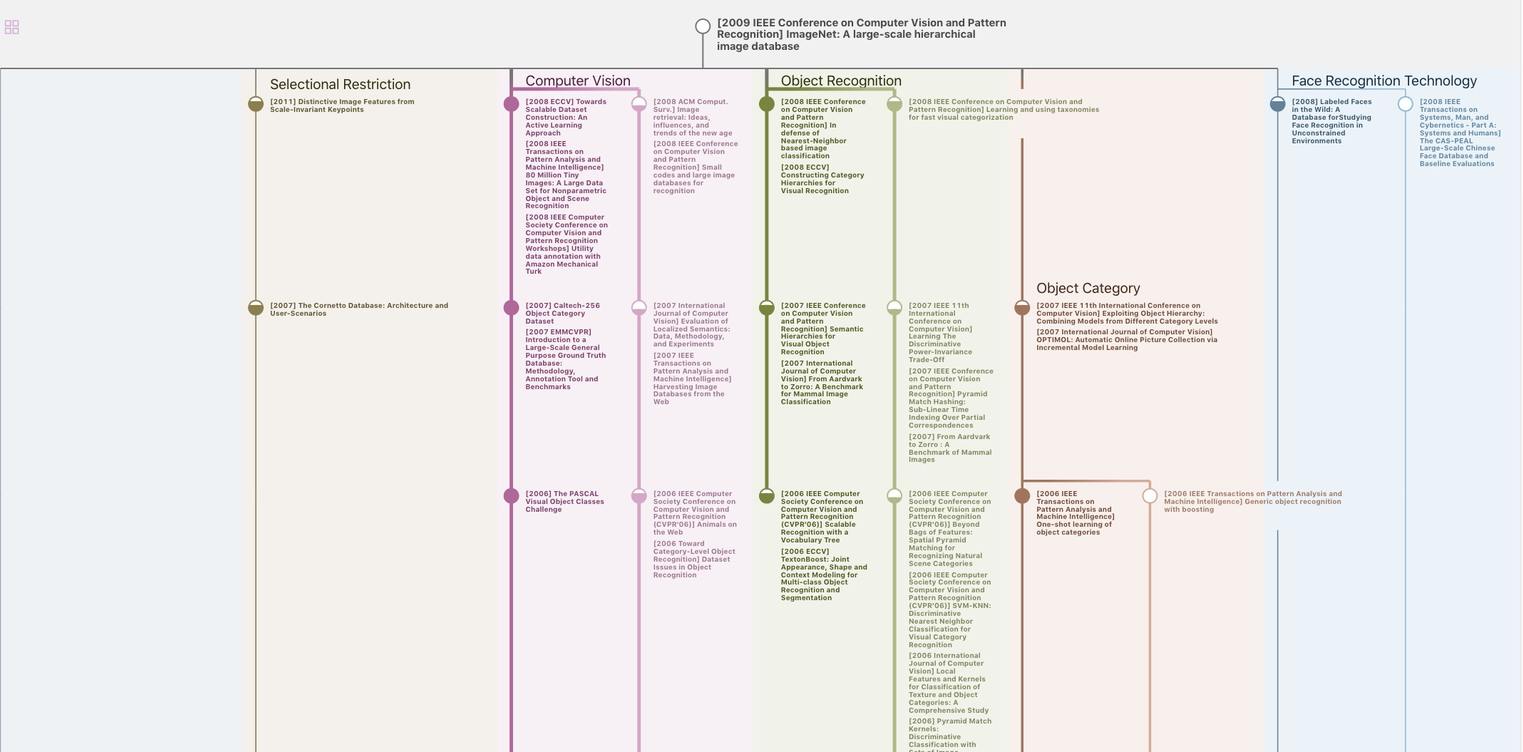
Generate MRT to find the research sequence of this paper
Chat Paper
Summary is being generated by the instructions you defined