Certifiably Robust Reinforcement Learning through Model-Based Abstract Interpretation
arXiv (Cornell University)(2023)
摘要
We present a reinforcement learning (RL) framework in which the learned policy comes with a machine-checkable certificate of provable adversarial robustness. Our approach, called CAROL, learns a model of the environment. In each learning iteration, it uses the current version of this model and an external abstract interpreter to construct a differentiable signal for provable robustness. This signal is used to guide learning, and the abstract interpretation used to construct it directly leads to the robustness certificate returned at convergence. We give a theoretical analysis that bounds the worst-case accumulative reward of CAROL. We also experimentally evaluate CAROL on four MuJoCo environments with continuous state and action spaces. On these tasks, CAROL learns policies that, when contrasted with policies from the state-of-the-art robust RL algorithms, exhibit: (i) markedly enhanced certified performance lower bounds; and (ii) comparable performance under empirical adversarial attacks.
更多查看译文
关键词
certifiably robust reinforcement learning,reinforcement learning,model-based
AI 理解论文
溯源树
样例
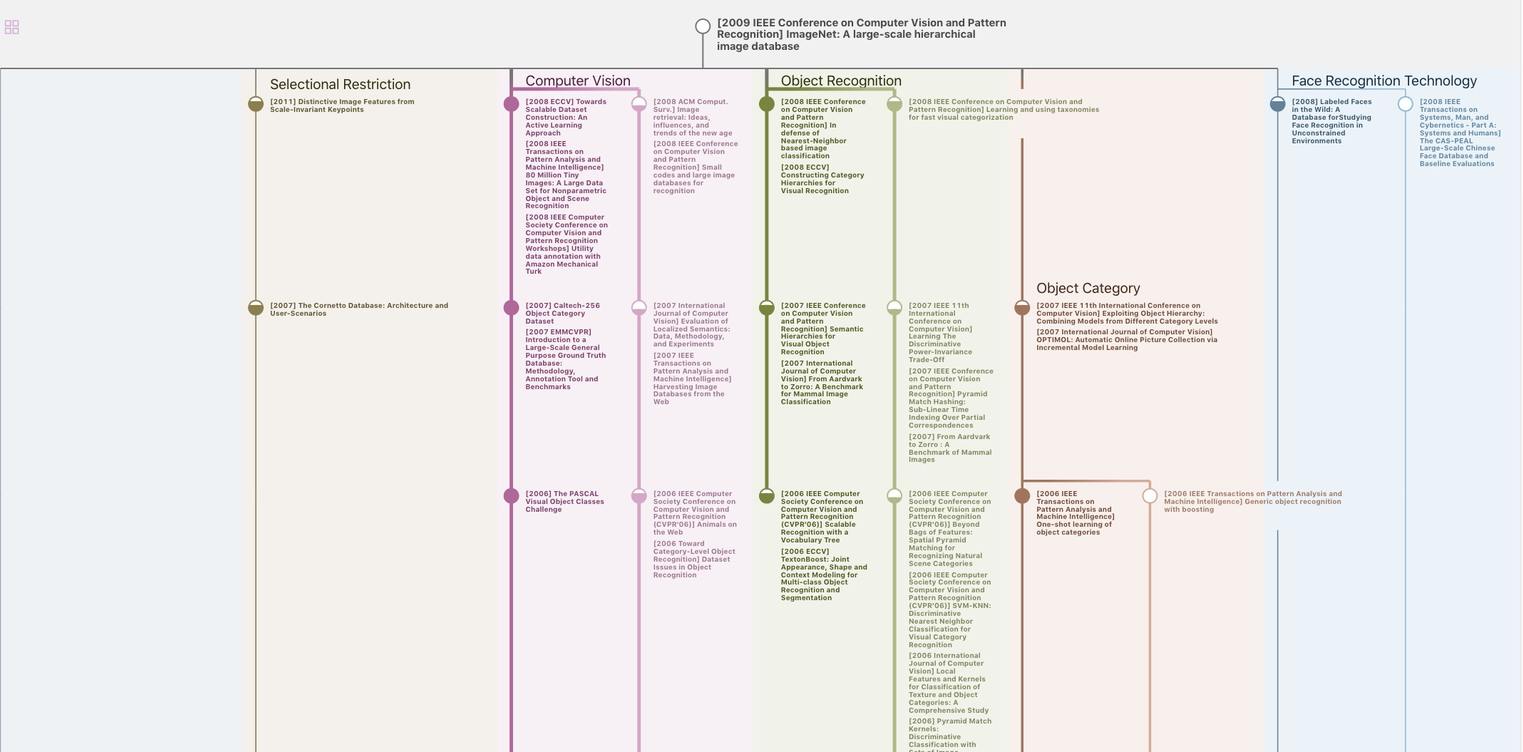
生成溯源树,研究论文发展脉络
Chat Paper
正在生成论文摘要