Running minimum in the best-choice problem
arXiv (Cornell University)(2021)
摘要
We consider the best-choice problem for independent (not necessarily iid) observations $X_1, \cdots, X_n$ with the aim of selecting the sample minimum. We show that in this full generality the monotone case of optimal stopping holds and the stopping domain may be defined by the sequence of monotone thresholds. In the iid case we get the universal lower bounds for the success probability. We cast the general problem with independent observations as a variational first-passage problem for the running minimum process which simplifies obtaining the formula for success probability. We illustrate this approach by revisiting the full-information game (where $X_j$'s are iid uniform-$[0,1]$), in particular deriving new representations for the success probability and its limit by $n \rightarrow \infty$. Two explicitly solvable models with discrete $X_j$'s are presented: in the first the distribution is uniform on $\{j,\cdots,n\}$, and in the second the distribution is uniform on $\{1,\cdots, n\}$. These examples are chosen to contrast two situations where the ties vanish or persist in the large-$n$ Poisson limit.
更多查看译文
关键词
minimum,best-choice
AI 理解论文
溯源树
样例
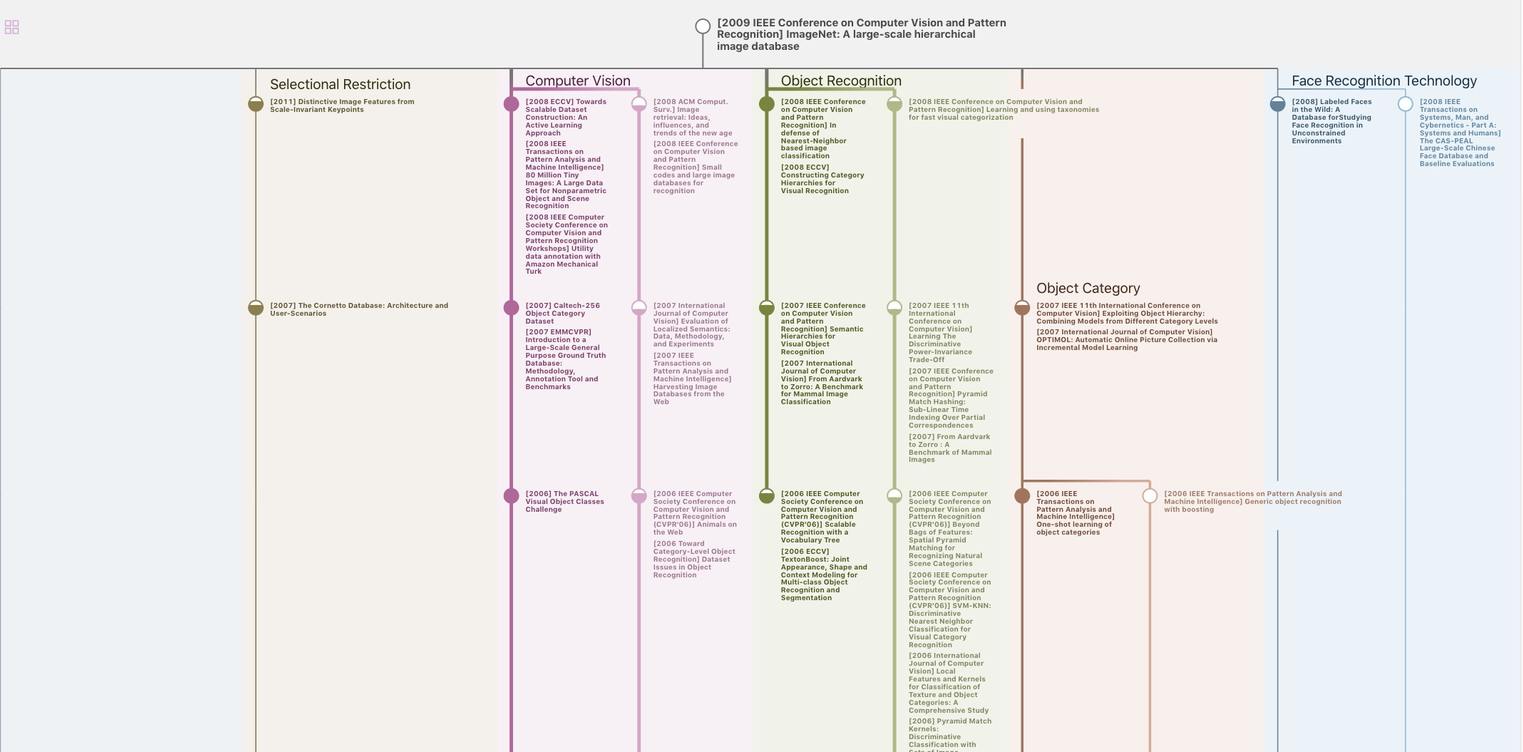
生成溯源树,研究论文发展脉络
Chat Paper
正在生成论文摘要