Learning to rank for uplift modeling
arXiv (Cornell University)(2020)
摘要
Uplift modeling has effectively been used in fields such as marketing and customer retention, to target those customers that are most likely to respond due to the campaign or treatment. Uplift models produce uplift scores which are then used to essentially create a ranking. We instead investigate to learn to rank directly by looking into the potential of learning-to-rank techniques in the context of uplift modeling. We propose a unified formalisation of different global uplift modeling measures in use today and explore how these can be integrated into the learning-to-rank framework. Additionally, we introduce a new metric for learning-to-rank that focusses on optimizing the area under the uplift curve called the promoted cumulative gain (PCG). We employ the learning-to-rank technique LambdaMART to optimize the ranking according to PCG and show improved results over standard learning-to-rank metrics and equal to improved results when compared with state-of-the-art uplift modeling. Finally, we show how learning-to-rank models can learn to optimize a certain targeting depth, however, these results do not generalize on the test set.
更多查看译文
关键词
uplift modeling,rank,learning
AI 理解论文
溯源树
样例
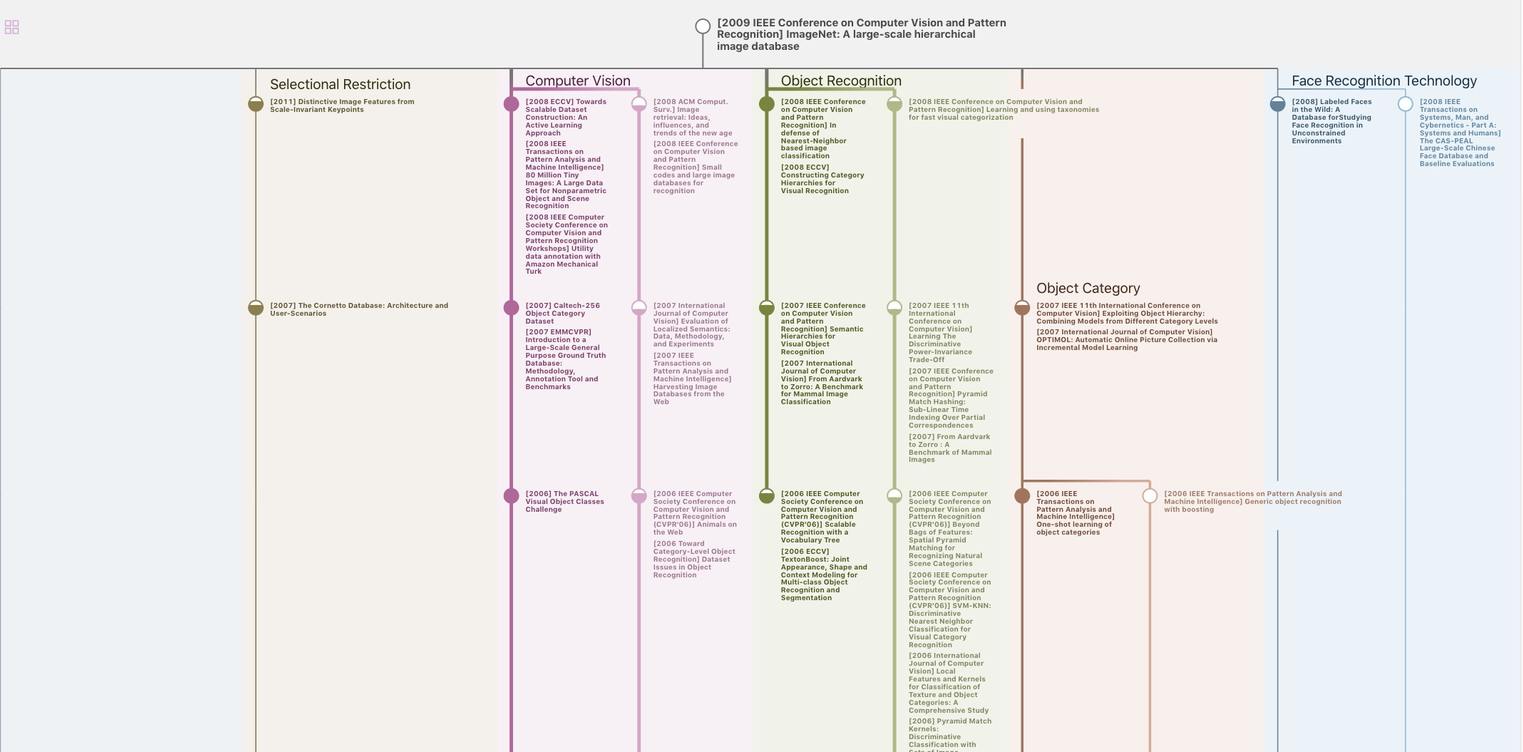
生成溯源树,研究论文发展脉络
Chat Paper
正在生成论文摘要