Principal Component Analysis Based Dominant Features Selection Method for Speaker Identification
Current trends in signal processing(2021)
摘要
Cepstrum based features are mostly used in speaker identification. Mel-frequency cepstrum coefficients (MFCCs) and their statistical properties (skewness, kurtosis and standard deviation) are used in this paper for text-dependent speaker identification. Principal component analysis (PCA) is employed to select the dominant feature vector representing the speaker characteristics. Multi-layer neural network is used as the classification engine. There occurs the inter-speaker variation of speech length uttering the same word. The feature speaker specific vector of defined dimension is derived with PCA. The statistical properties of the selected features and the effects of the first MFCC are studied in terms of speaker identification performance and convergence speed of neural network as illustrated by the experimental results.
更多查看译文
关键词
selection method,features,identification
AI 理解论文
溯源树
样例
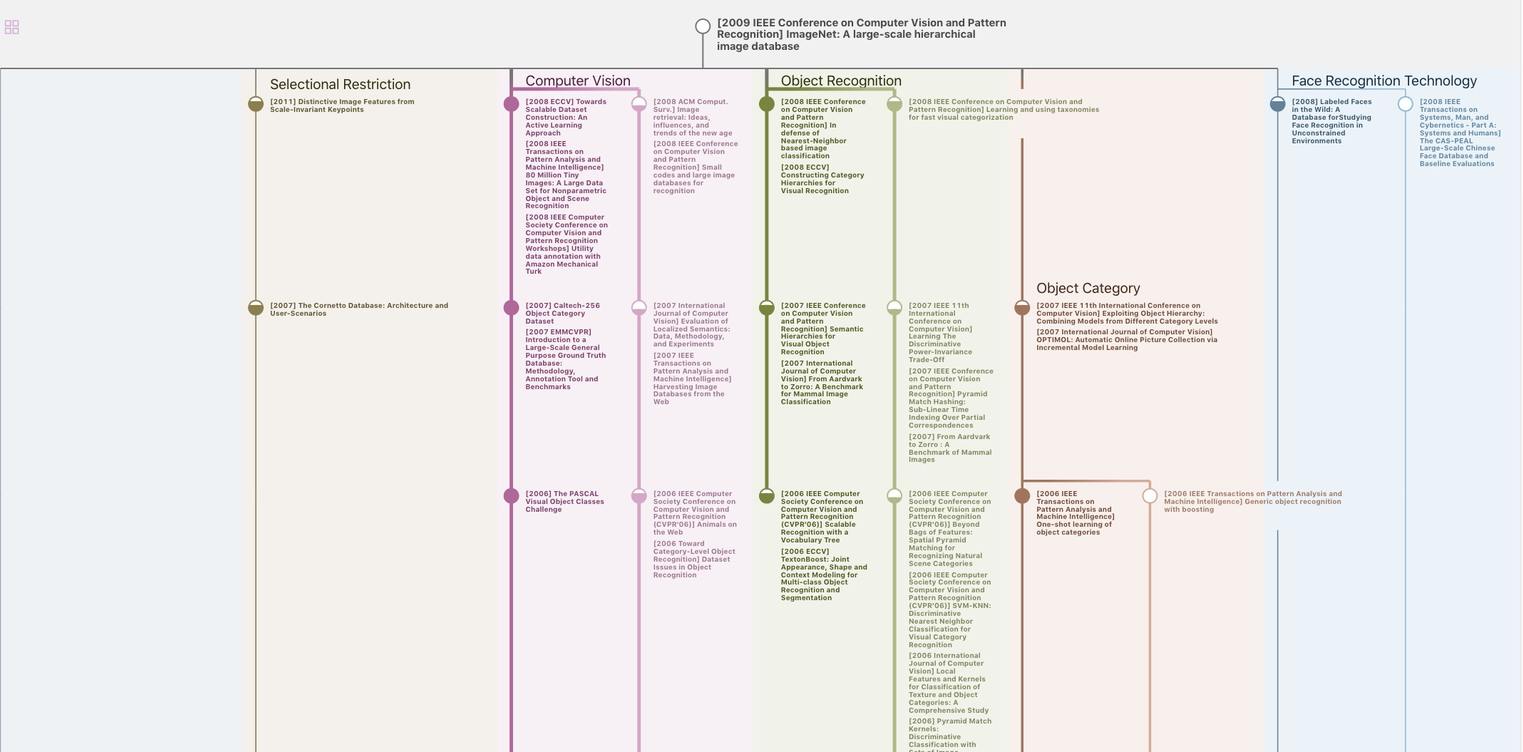
生成溯源树,研究论文发展脉络
Chat Paper
正在生成论文摘要