Regret Minimization Experience Replay.
arXiv (Cornell University)(2021)
摘要
In reinforcement learning, experience replay stores past samples for further reuse. Prioritized sampling is a promising technique to better utilize these samples. Previous criteria of prioritization include TD error, recentness and corrective feedback, which are mostly heuristically designed. In this work, we start from the regret minimization objective, and obtain an optimal prioritization strategy for Bellman update that can directly maximize the return of the policy. The theory suggests that data with higher hindsight TD error, better on-policiness and more accurate Q value should be assigned with higher weights during sampling. Thus most previous criteria only consider this strategy partially. We not only provide theoretical justifications for previous criteria, but also propose two new methods to compute the prioritization weight, namely ReMERN and ReMERT. ReMERN learns an error network, while ReMERT exploits the temporal ordering of states. Both methods outperform previous prioritized sampling algorithms in challenging RL benchmarks, including MuJoCo, Atari and Meta-World.
更多查看译文
关键词
minimization,experience
AI 理解论文
溯源树
样例
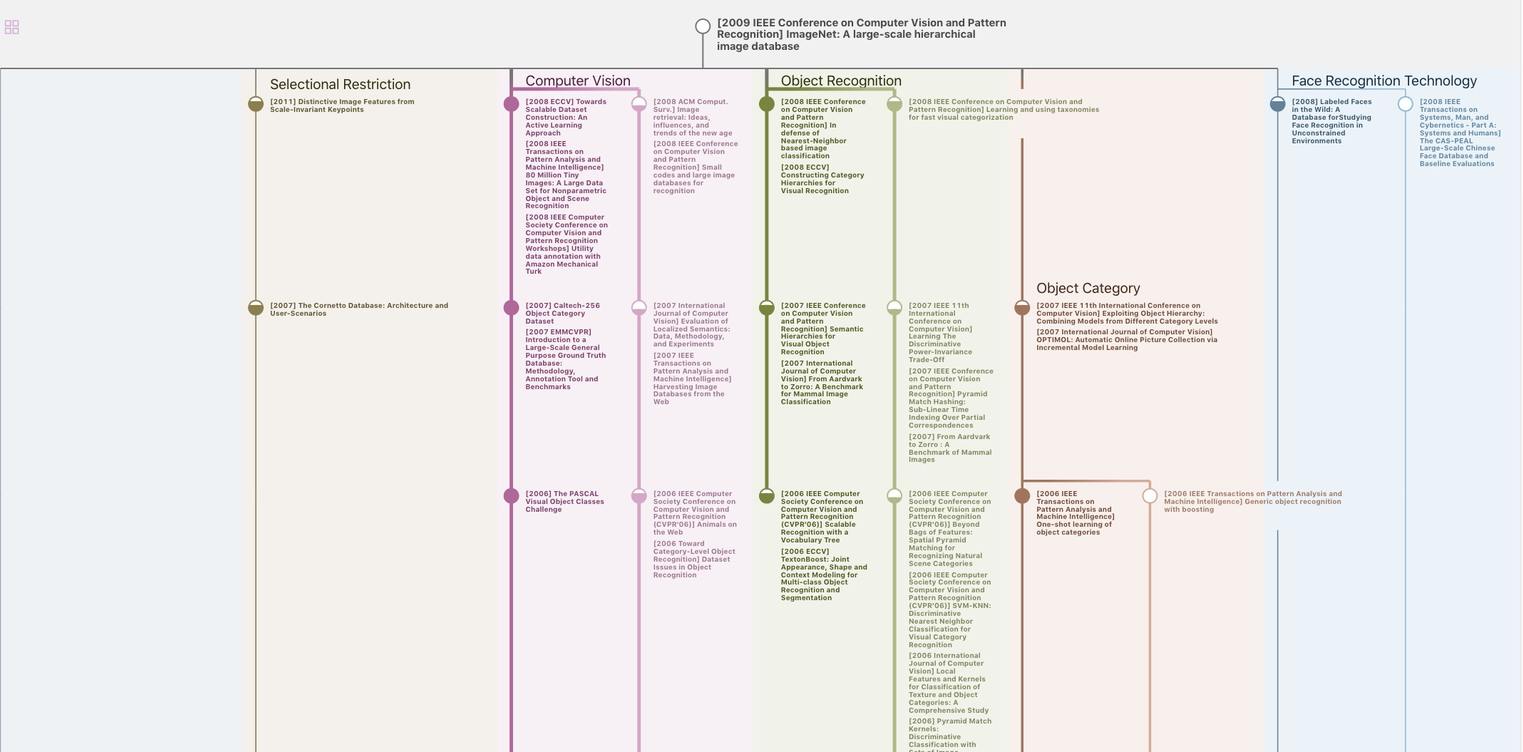
生成溯源树,研究论文发展脉络
Chat Paper
正在生成论文摘要