Selective Intervention Planning using RMABs: Increasing Program Engagement to Improve Maternal and Child Health Outcomes
arXiv (Cornell University)(2021)
摘要
India has a maternal mortality ratio of 113 and child mortality ratio of 2830 per 100,000 live births. Lack of access to preventive care information is a major contributing factor for these deaths, especially in low-income households. We work with ARMMAN, a non-profit based in India, to further the use of call-based information programs by early-on identifying women who might not engage with these programs that are proven to affect health parameters positively. We analyzed anonymized call-records of over 300,000 women registered in an awareness program created by ARMMAN that uses cellphone calls to regularly disseminate health related information. We built machine learning based models to predict the long term engagement pattern from call logs and beneficiaries' demographic information, and discuss the applicability of this method in the real world through a pilot validation. Through a randomized controlled trial, we show that using our model's predictions to make interventions boosts engagement metrics by 14.3%. We then formulate the intervention planning problem as restless multi-armed bandits (RMABs), and present preliminary results using this approach.
更多查看译文
关键词
child health outcomes,rmabs,program engagement,planning,maternal
AI 理解论文
溯源树
样例
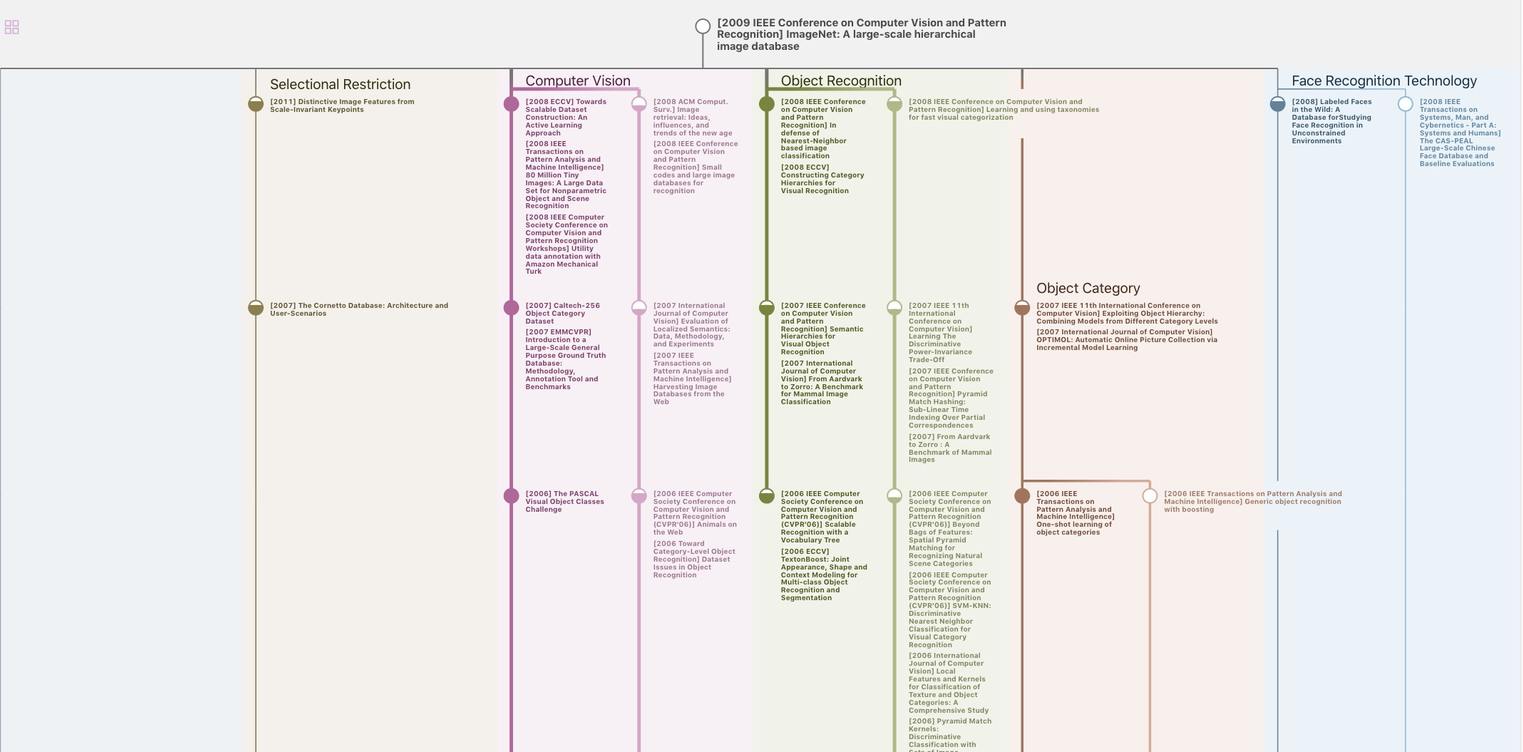
生成溯源树,研究论文发展脉络
Chat Paper
正在生成论文摘要