HEBO: Heteroscedastic Evolutionary Bayesian Optimisation.
arXiv (Cornell University)(2020)
摘要
Inspired by the increasing desire to efficiently tune machine learning hyper-parameters, in this work we rigorously analyse conventional and non-conventional assumptions inherent to Bayesian optimisation. Across an extensive set of experiments we conclude that: 1) the majority of hyper-parameter tuning tasks exhibit heteroscedasticity and non-stationarity, 2) multi-objective acquisition ensembles with Pareto-front solutions significantly improve queried configurations, and 3) robust acquisition maximisation affords empirical advantages relative to its non-robust counterparts. We hope these findings may serve as guiding principles, both for practitioners and for further research in the field.
更多查看译文
关键词
heteroscedastic evolutionary bayesian optimisation,hebo
AI 理解论文
溯源树
样例
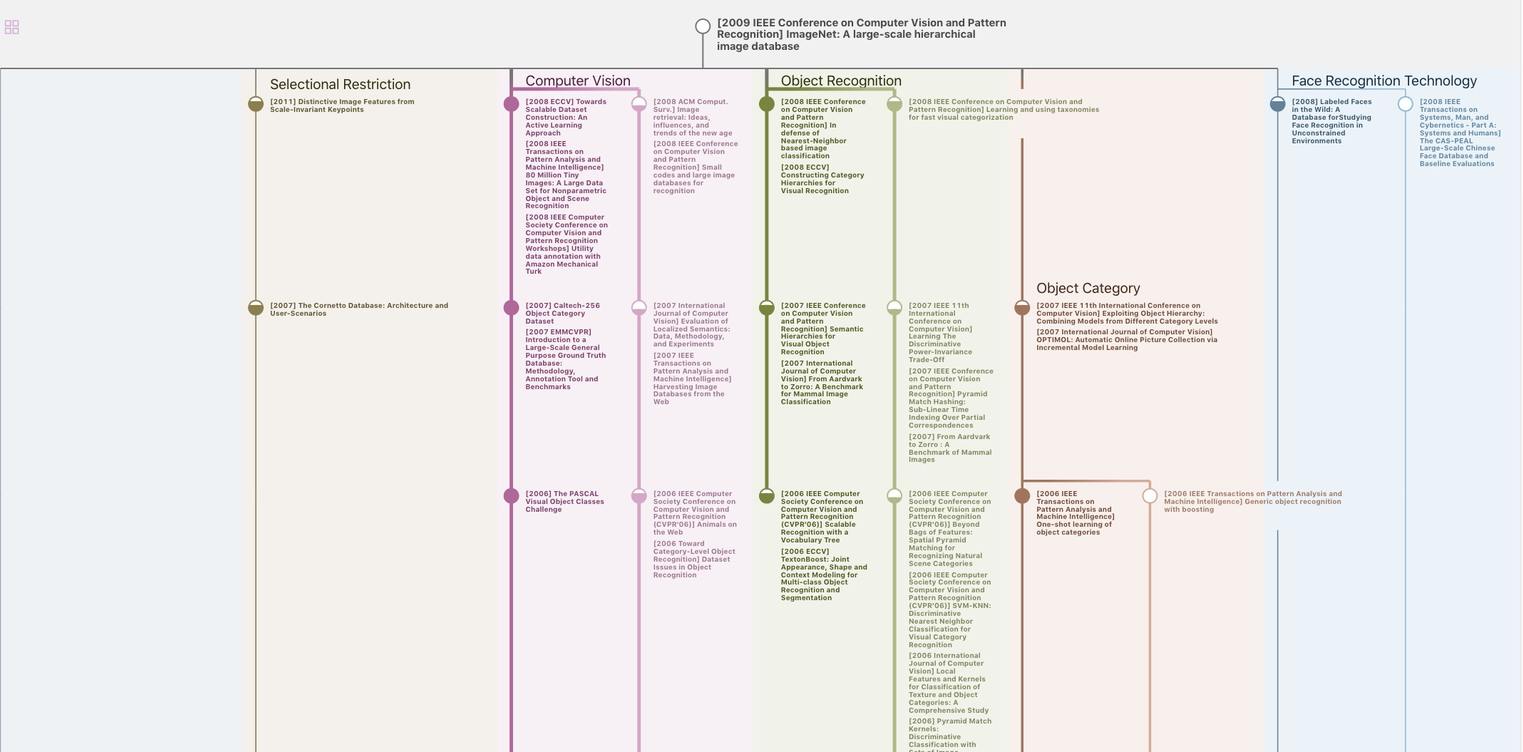
生成溯源树,研究论文发展脉络
Chat Paper
正在生成论文摘要