Enhanced Quantified Local Implicit Neural Representation for Image Compression
IEEE SIGNAL PROCESSING LETTERS(2023)
摘要
Recently, implicit neural representation (INR) has been applied to image compression. However, the rate-distortion performance of most existing INR-based image compression methods is still obviously inferior to the state-of-the-art image compression methods. In this letter, we propose an Enhanced Quantified Local Implicit Neural Representation (EQLINR) for image compression by enhancing the utilization of local relationships of INR and narrow the quantization gap between training and encoding to further improve the performance of INR-based image compression. Our framework consists of latent representation and the corresponding implicit neural network consisting of MLP and CNN, which can transform the latent representation into the image space. To enhance local relationships utilization, we design a local enhancement module (LEM) consisted of CNN to capture the neighborhood relationships of the reconstructed image from MLP. Furthermore, to mitigate the performance loss caused by quantization of latent representation, we employ an enhanced quantization scheme (EQS) in our training process. We use uniform noise for network initialization and then use Stochastic Gumbel Annealing (SGA) with dynamic temperature regulation as a proxy function for quantization during training. Extensive experimental results demonstrate that our approach significantly the compression performance of INR-based image compression, and even better than BPG.
更多查看译文
关键词
Image coding,implicit neual representation,deep learning,enhanced quantization scheme
AI 理解论文
溯源树
样例
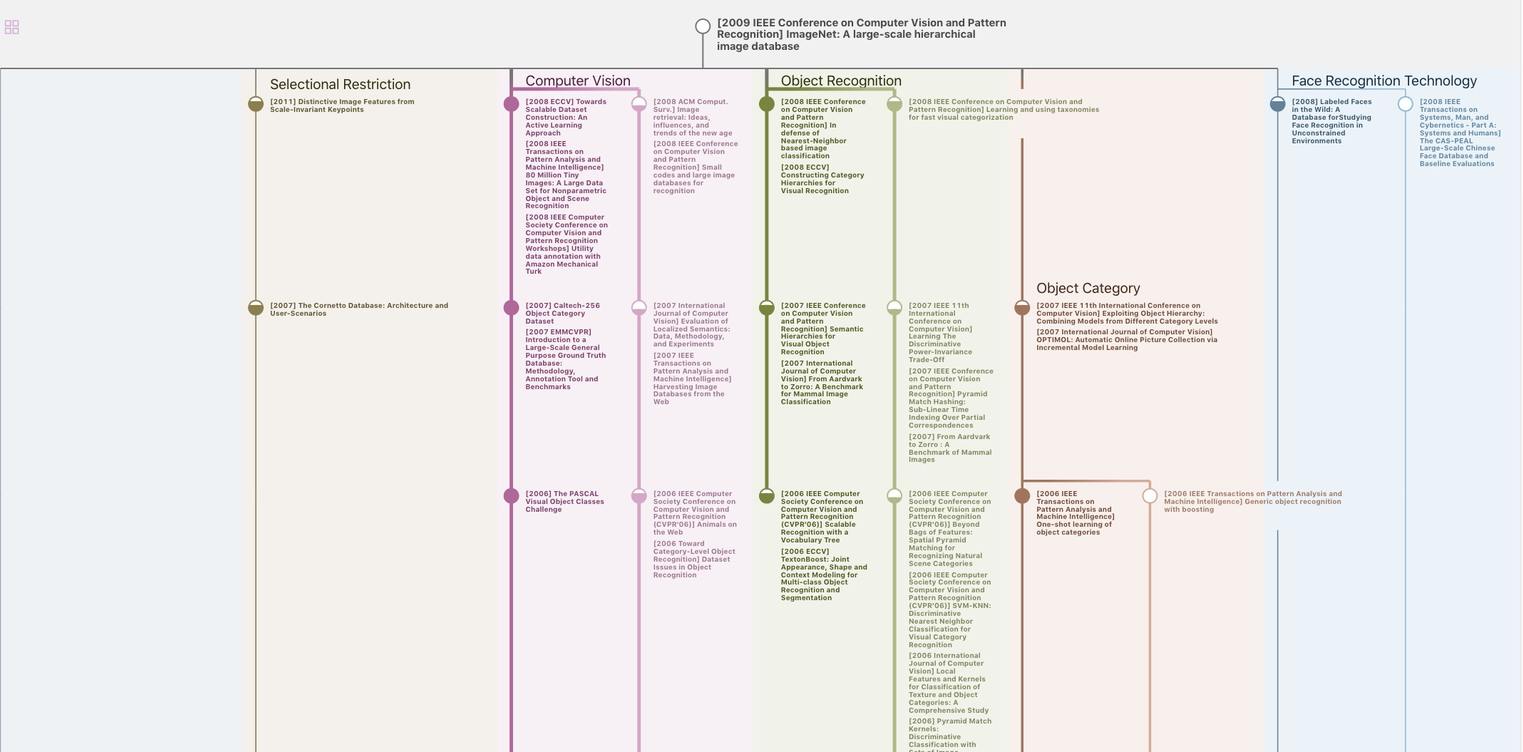
生成溯源树,研究论文发展脉络
Chat Paper
正在生成论文摘要