A Novel Loss for Change Point Detection Models With Time-Invariant Representations
IEEE SIGNAL PROCESSING LETTERS(2023)
摘要
Change point detection (CPD) refers to the problem of detecting changes in the statistics of pseudo-stationary signals or time series. A recent trend in CPD research is to replace the traditional statistical tests with distribution-free autoencoder-based algorithms, which can automatically learn complex patterns in time series data. In particular, the so-called time-invariant representation (TIRE) models have gained traction, as these separately encode time-variant and time-invariant subfeatures, as opposed to traditional autoencoders. However, optimizing the trade-off between two loss terms, i.e., the reconstruction loss and the time-invariant loss, is challenging. To address this issue, we propose a novel loss function that elegantly combines both losses without the need for manually tuning a trade-off hyperparameter. We demonstrate that this new hyperparameter-free loss, in combination with a relatively simple convolutional neural network (CNN), consistently achieves superior or comparable performance compared to the manually-tuned baseline TIRE models across diverse benchmark datasets, both simulated and real-life. In addition, we present a representation analysis, demonstrating that the distribution of the time-invariant features extracted by our model is more concentrated within the same segment (more so than with previous TIRE models), which implies that these features can potentially be used for other applications, such as classification and clustering.
更多查看译文
关键词
TV,Tires,Feature extraction,Diamonds,Time series analysis,Decoding,Windows,Autoencoder,change point detection,time-invariant representation (TIRE),unsupervised learning
AI 理解论文
溯源树
样例
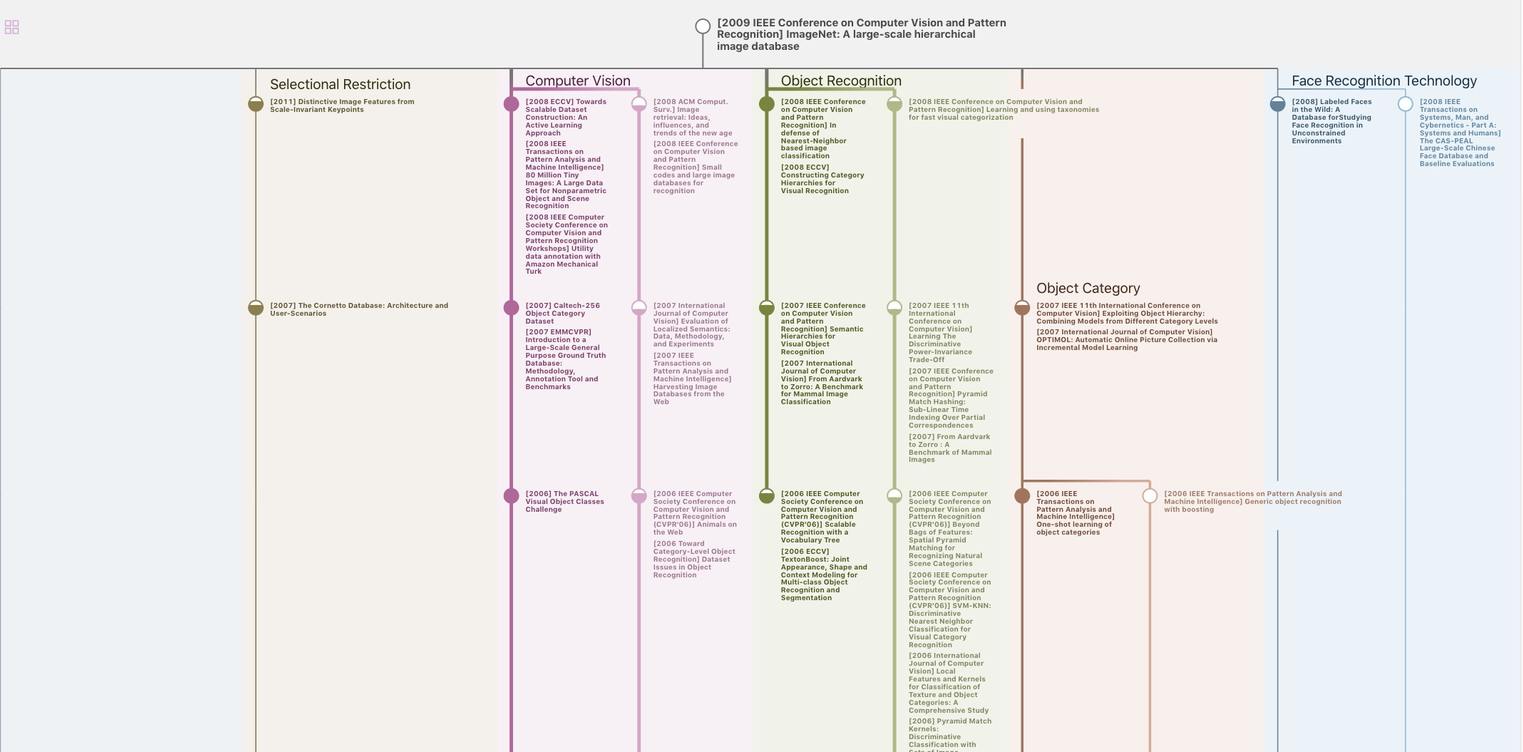
生成溯源树,研究论文发展脉络
Chat Paper
正在生成论文摘要