A Hybrid Deep Learning Model for Rapid Probabilistic Earthquake Source Parameter Estimation With Displacement Waveforms From a Flexible Set of Seismic or HR-GNSS Stations
IEEE TRANSACTIONS ON GEOSCIENCE AND REMOTE SENSING(2023)
摘要
The prompt and reliable determination of seismic source parameters, which provide information on earthquake location (LOC), magnitude (MAG), and focal mechanism (FM), is an imperative undertaking after a destructive earthquake. Deep learning (DL) techniques, owing to their excellent ability to extract pertinent features from waveforms, have gained growing popularity in addressing the challenge of rapid seismic source characterization. However, most existing DL models, particularly those for FM estimation, rely on a fixed configuration of stations. In this work, we propose a hybrid DL model tailored to probabilistic seismic source characterization using displacement waveforms from a flexible set of seismic or high rate Global Navigation Satellite System (HR-GNSS) stations. The proposed model encompasses a convolutional neural network (CNN) module, a graph module, and a mixture density network (MDN) module. Preliminary features are first extracted by the CNN module for each station. The graph module, where a graph attention network (GAT) branch and a maximum aggregation branch are included, then aggregates these features, enabling the adaptive learning of edge information among stations. The MDN module finally calculates the posterior probability distribution of each source parameter. The proposed model performed best compared to benchmark models within synthetic test datasets. The established model was then applied to estimate the source parameters of five events with $M > $ 5 in the 2019 Ridgecrest earthquake sequence and three other representative earthquakes worldwide. Probabilistic estimates that are consistent with known published ground-truth values could be obtained within seconds, verifying the effectiveness and efficiency of the proposed hybrid model in real-world scenarios.
更多查看译文
关键词
Earthquakes,Feature extraction,Frequency modulation,Estimation,Probabilistic logic,Data models,Convolutional neural networks,Deep learning (DL),earthquake source observations,high-rate Global Navigation Satellite System (HR-GNSS),probabilistic inversion,seismology
AI 理解论文
溯源树
样例
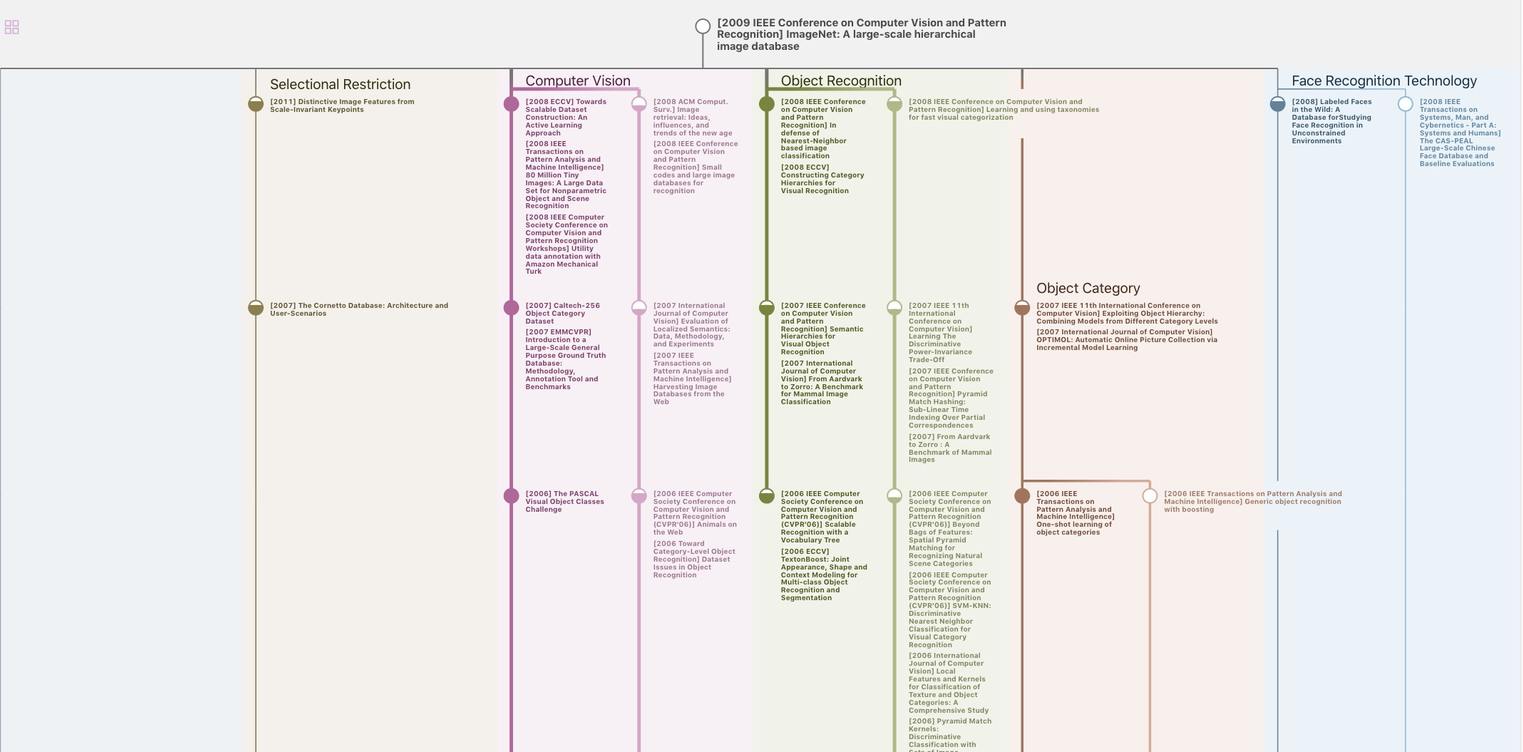
生成溯源树,研究论文发展脉络
Chat Paper
正在生成论文摘要