SWiMM DEEPeR: A Simulated Underwater Environment for Tracking Marine Mammals Using Deep Reinforcement Learning and BlueROV2.
2023 IEEE Conference on Games (CoG)(2023)
摘要
This paper offers a feasibility study on using simulated environments for training autonomous underwater vehicles (AUVs). With the goal of monitoring marine megafauna, we propose a Unity-hosted simulation of a realistic open ocean environment, with a focus on simulating Blue Robotics’ BlueROV2. The result is SWiMM DEEPeR
1
, coupling the former simulation with a reinforcement learning (RL) pipeline. Animated marine mammal models emulate the target objects of the real-world deployment scenario, offering a solution in a new application space (conservation) as well as a new problem space (visual active tracking). We provide experiments with respect to each stage of the proposed pipeline: i) image similarity experiments provide evidence for decisions around image rendering and data transfer, ii) autoencoder training demonstrates the feasibility of mapping raw images to low-dimensional feature representations, iii) agent training demonstrates successful self-learnt vehicle control.
更多查看译文
关键词
Unity,active tracking,marine mammals,simulation environment,reinforcement learning,autoencoders
AI 理解论文
溯源树
样例
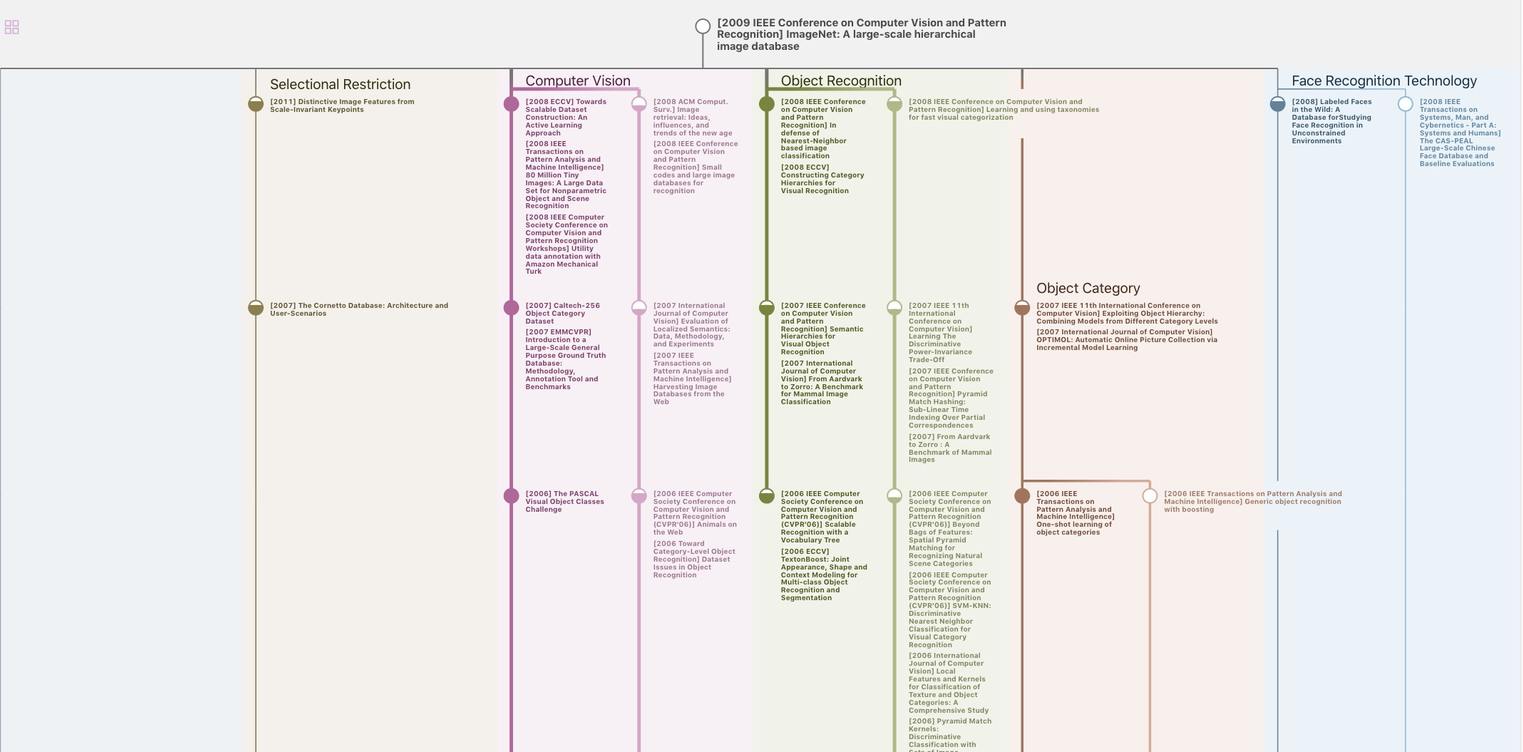
生成溯源树,研究论文发展脉络
Chat Paper
正在生成论文摘要