Representing Source Movement in Sequences of Telescopic Images Based on Contrastive Learning for Asteroid Detection
2023 27th International Computer Science and Engineering Conference (ICSEC)(2023)
摘要
The study of asteroids, the moving rocky objects, not only makes feasible prevention of hazardous collisions, but also provides better understanding of the solar system in it's early stage. However, existing software for asteroid detection requires manual parameter setup, which is a sensitive task requiring an experienced person. Moreover, the sequence of images contains only the brightness of each image while the key feature of asteroid detection is its movement. In this research, we propose a contrastive deep learning model to learn the motion representation of asteroids in a sequence of images. The representation is used to classify a sequence of images by investigation of distance calculation using Euclidean distance and cosine similarity. Moreover, simple classifiers including k-nearest neighbors (KNN) and logistic regression (LR) are implemented to evaluate their ability to classify the motion representation. The representation generation model is trained on sky images from the Gravitational-wave Optical Transient Observer (GOTO) survey. For motion representation, the classification results show that the best classifier achieves F1 score of 88.32% on the validation set and 86.60% on the test set. Moreover, k-nearest neighbors model underperforms the best model by -3.62% of F1 score in the test set. As a result, our approach replaces the hand-engineering process and produces more promising performance.
更多查看译文
关键词
Asteroid,Contrastive learning model,Machine Learning
AI 理解论文
溯源树
样例
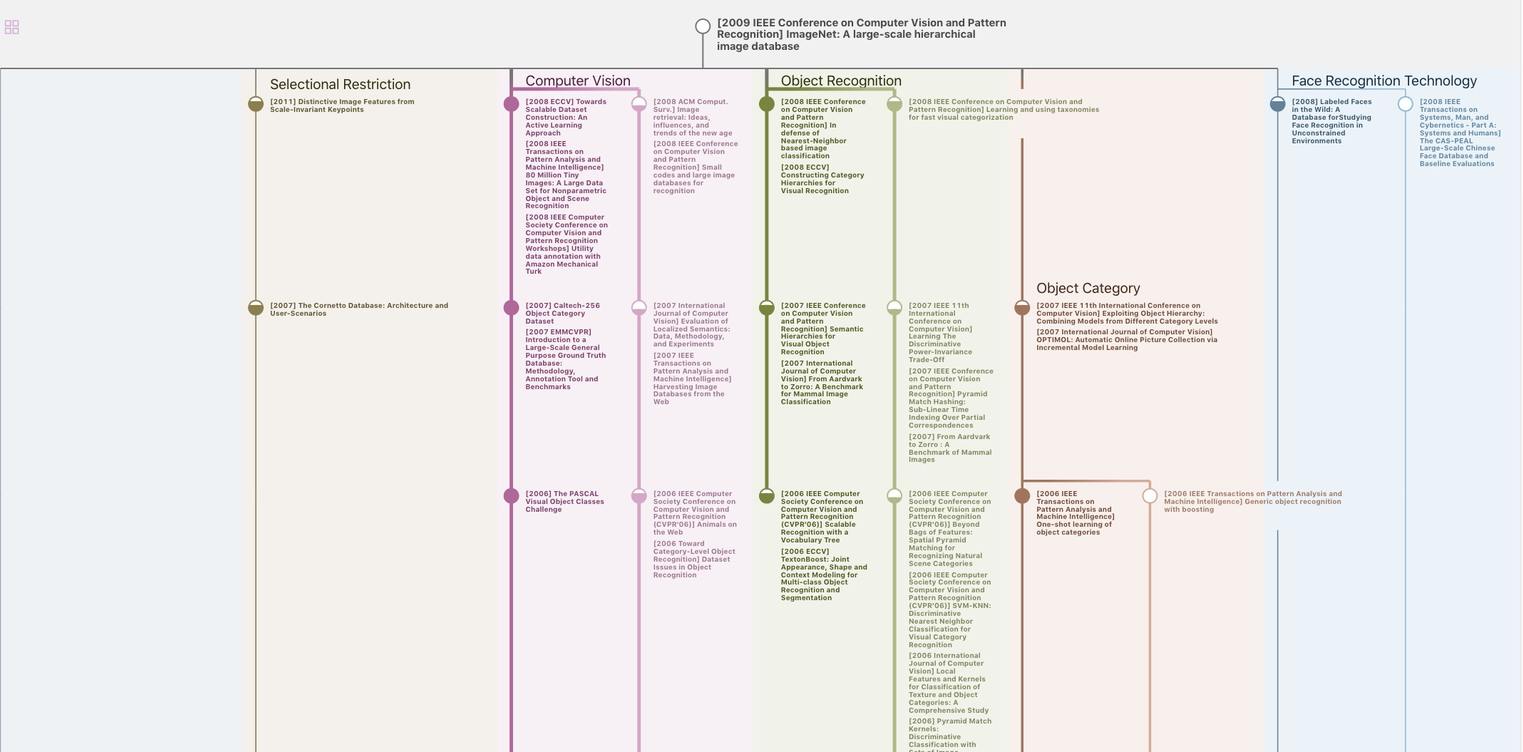
生成溯源树,研究论文发展脉络
Chat Paper
正在生成论文摘要