Joint modelling of task requirements and worker preferences based on heterogeneous features and multiple interactions for knowledge-intensive crowdsourcing recommendation
INTERNATIONAL JOURNAL OF BIO-INSPIRED COMPUTATION(2023)
摘要
Automatic worker recommendation has become a key technology in knowledge-intensive crowdsourcing (KIC). However, KIC recommendation encounters the task cold-start problem in nature as only newly posted tasks need to be matched with workers. Current studies fail to accurately model both tasks and workers in the task cold-start scenario, and ignore the problem of task clarity in task requirements understanding and treat task features linearly in worker preferences estimation. Therefore, this paper proposed a heterogeneous features and multiple interactions-based deep neural framework (called HFMIRec) to assist new task completion more smartly in KIC. Specifically, different types of task features can be flexibly incorporated to tackle the cold-start problem. To accurately model both tasks and workers, multiple interactions between tasks and workers are identified and learned by attentive neural networks in the framework. Extensive experiments on a real-world dataset demonstrate the effectiveness of the proposed model.
更多查看译文
关键词
crowdsourcing,task cold-start,worker recommendation,supply-demand matching,recommender system
AI 理解论文
溯源树
样例
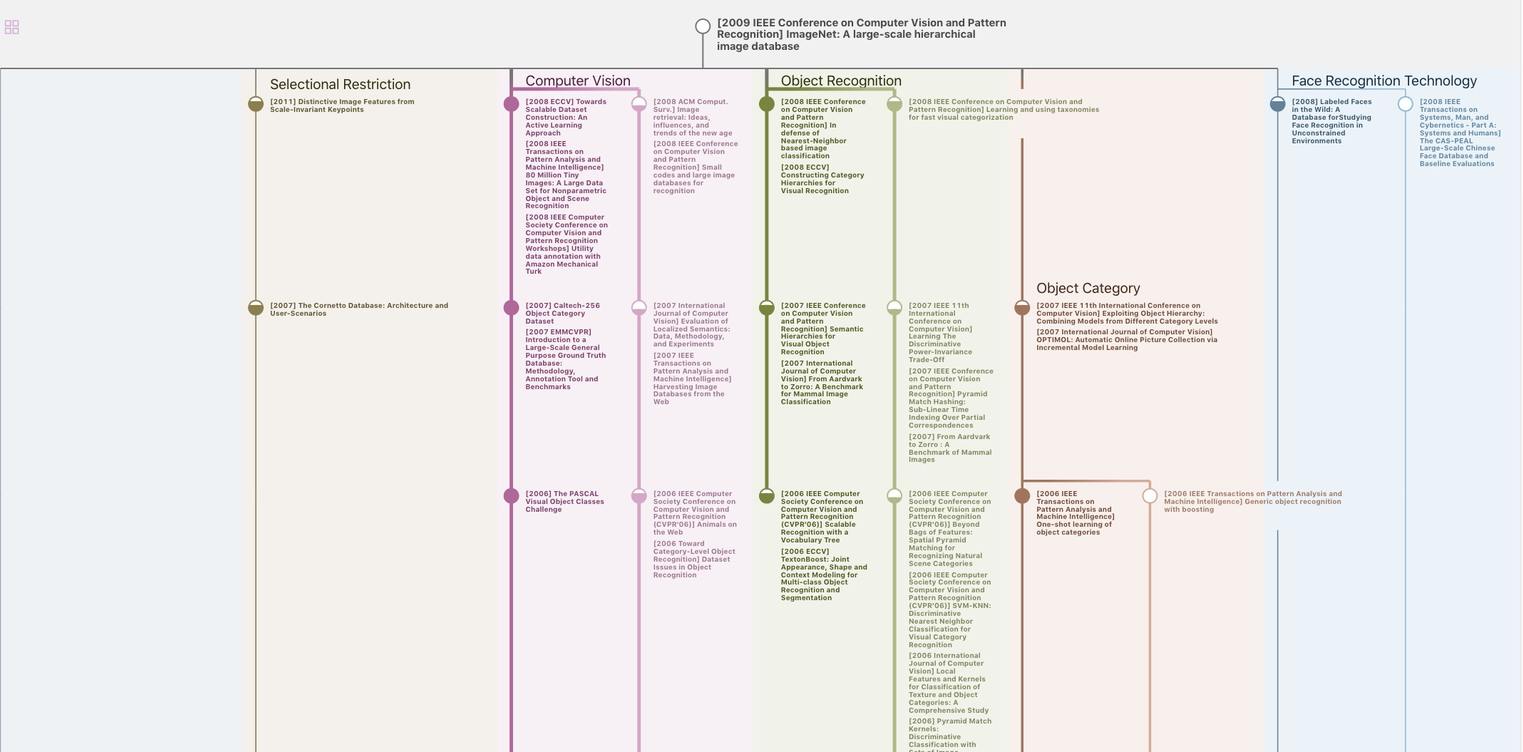
生成溯源树,研究论文发展脉络
Chat Paper
正在生成论文摘要