Generalization by Adaptation: Diffusion-Based Domain Extension for Domain-Generalized Semantic Segmentation
2024 IEEE/CVF Winter Conference on Applications of Computer Vision (WACV)(2023)
Abstract
When models, e.g., for semantic segmentation, are applied to images that are vastly different from training data, the performance will drop significantly. Domain adaptation methods try to overcome this issue, but need samples from the target domain. However, this might not always be feasible for various reasons and therefore domain generalization methods are useful as they do not require any target data. We present a new diffusion-based domain extension (DIDEX) method and employ a diffusion model to generate a pseudo-target domain with diverse text prompts. In contrast to existing methods, this allows to control the style and content of the generated images and to introduce a high diversity. In a second step, we train a generalizing model by adapting towards this pseudo-target domain. We outperform previous approaches by a large margin across various datasets and architectures without using any real data. For the generalization from GTA5, we improve state-of-the-art mIoU performance by 3.8% absolute on average and for SYNTHIA by 11.8% absolute, marking a big step for the generalization performance on these benchmarks. Code is available at https://github.com/JNiemeijer/DIDEX
MoreTranslated text
Key words
Algorithms,Machine learning architectures,formulations,and algorithms,Algorithms,Image recognition and understanding
AI Read Science
Must-Reading Tree
Example
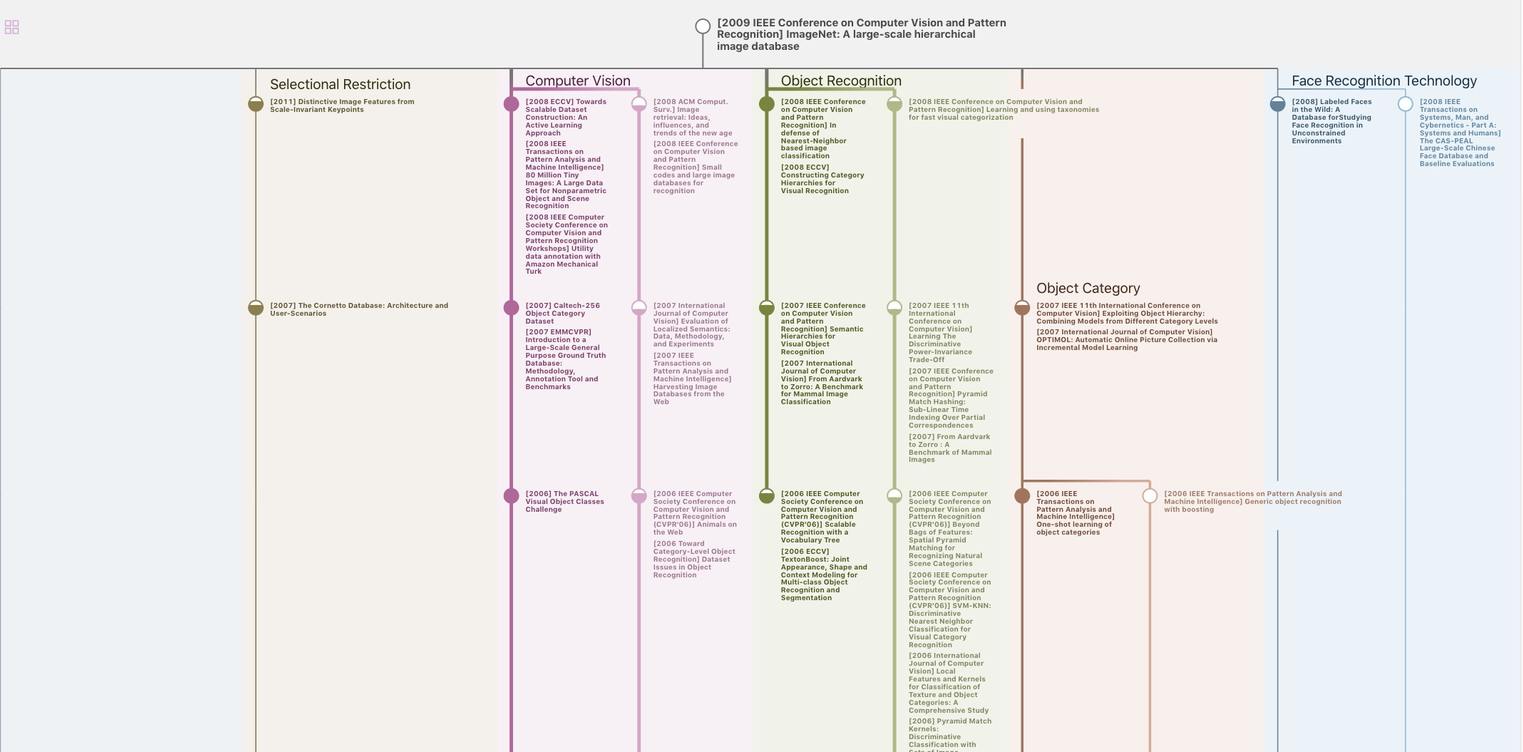
Generate MRT to find the research sequence of this paper
Chat Paper
Summary is being generated by the instructions you defined