RJHMC-Tree for Exploration of the Bayesian Decision Tree Posterior
CoRR(2023)
Abstract
Decision trees have found widespread application within the machine learning community due to their flexibility and interpretability. This paper is directed towards learning decision trees from data using a Bayesian approach, which is challenging due to the potentially enormous parameter space required to span all tree models. Several approaches have been proposed to combat this challenge, with one of the more successful being Markov chain Monte Carlo (MCMC) methods. The efficacy and efficiency of MCMC methods fundamentally rely on the quality of the so-called proposals, which is the focus of this paper. In particular, this paper investigates using a Hamiltonian Monte Carlo (HMC) approach to explore the posterior of Bayesian decision trees more efficiently by exploiting the geometry of the likelihood within a global update scheme. Two implementations of the novel algorithm are developed and compared to existing methods by testing against standard datasets in the machine learning and Bayesian decision tree literature. HMC-based methods are shown to perform favourably with respect to predictive test accuracy, acceptance rate, and tree complexity.
MoreTranslated text
AI Read Science
Must-Reading Tree
Example
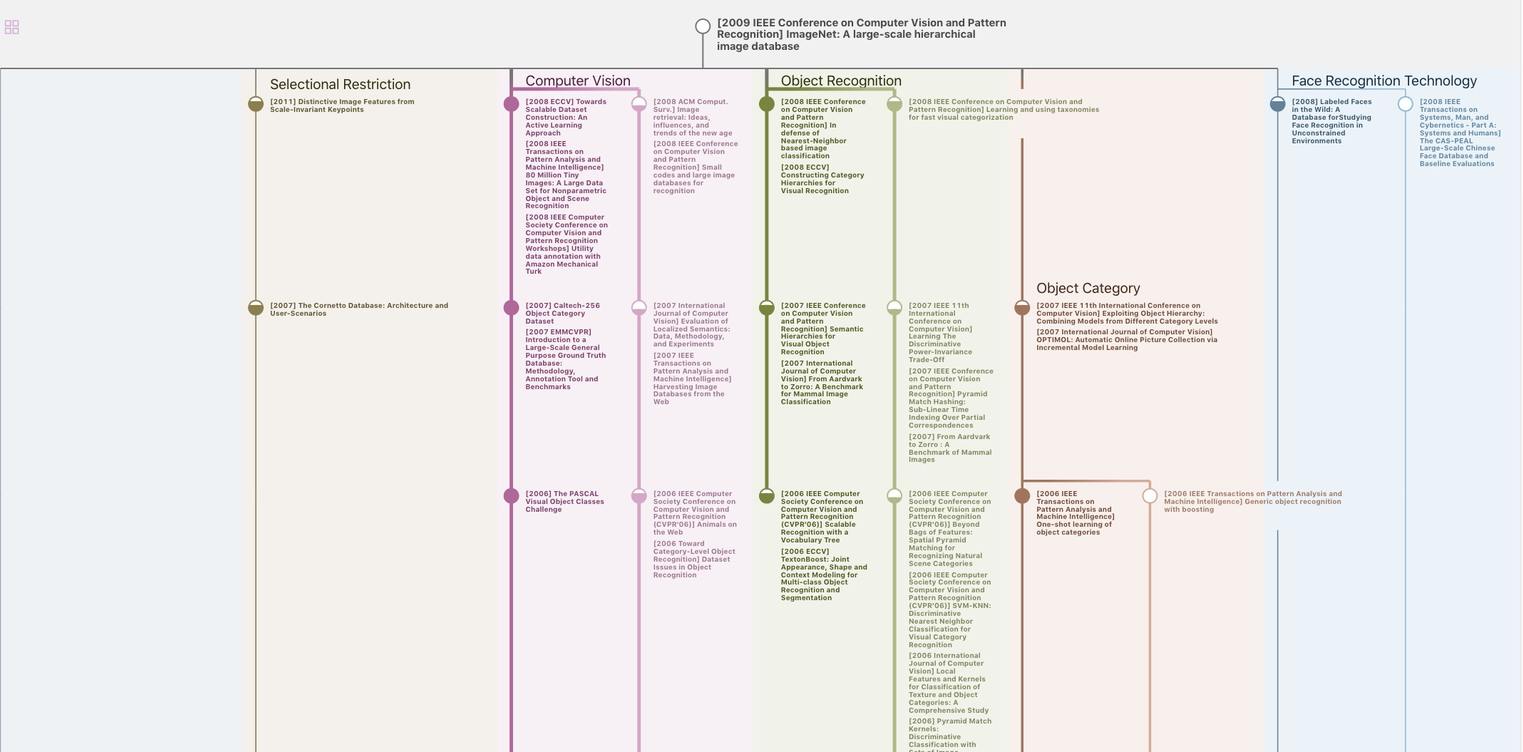
Generate MRT to find the research sequence of this paper
Chat Paper
Summary is being generated by the instructions you defined