RobustCalib: Robust Lidar-Camera Extrinsic Calibration with Consistency Learning
CoRR(2023)
摘要
Current traditional methods for LiDAR-camera extrinsics estimation depend on
offline targets and human efforts, while learning-based approaches resort to
iterative refinement for calibration results, posing constraints on their
generalization and application in on-board systems. In this paper, we propose a
novel approach to address the extrinsic calibration problem in a robust,
automatic, and single-shot manner. Instead of directly optimizing extrinsics,
we leverage the consistency learning between LiDAR and camera to implement
implicit re-calibartion. Specially, we introduce an appearance-consistency loss
and a geometric-consistency loss to minimizing the inconsitency between the
attrbutes (e.g., intensity and depth) of projected LiDAR points and the
predicted ones. This design not only enhances adaptability to various scenarios
but also enables a simple and efficient formulation during inference. We
conduct comprehensive experiments on different datasets, and the results
demonstrate that our method achieves accurate and robust performance. To
promote further research and development in this area, we will release our
model and code.
更多查看译文
AI 理解论文
溯源树
样例
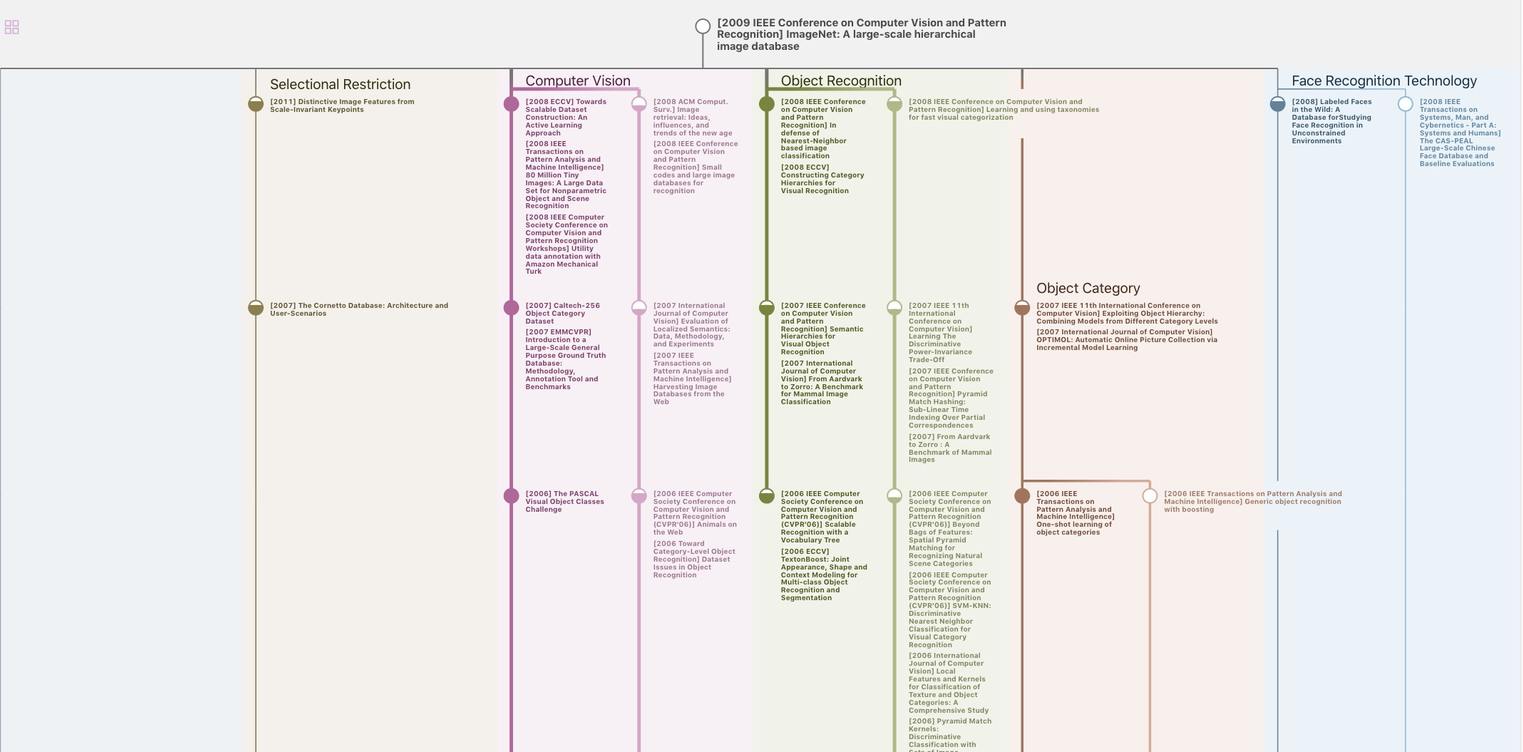
生成溯源树,研究论文发展脉络
Chat Paper
正在生成论文摘要