Acoustic Signal Analysis with Deep Neural Network for Detecting Fault Diagnosis in Industrial Machines
CoRR(2023)
摘要
Detecting machine malfunctions at an early stage is crucial for reducing
interruptions in operational processes within industrial settings. Recently,
the deep learning approach has started to be preferred for the detection of
failures in machines. Deep learning provides an effective solution in fault
detection processes thanks to automatic feature extraction. In this study, a
deep learning-based system was designed to analyze the sound signals produced
by industrial machines. Acoustic sound signals were converted into Mel
spectrograms. For the purpose of classifying spectrogram images, the
DenseNet-169 model, a deep learning architecture recognized for its
effectiveness in image classification tasks, was used. The model was trained
using the transfer learning method on the MIMII dataset including sounds from
four types of industrial machines. The results showed that the proposed method
reached an accuracy rate varying between 97.17% and 99.87% at different Sound
Noise Rate levels.
更多查看译文
AI 理解论文
溯源树
样例
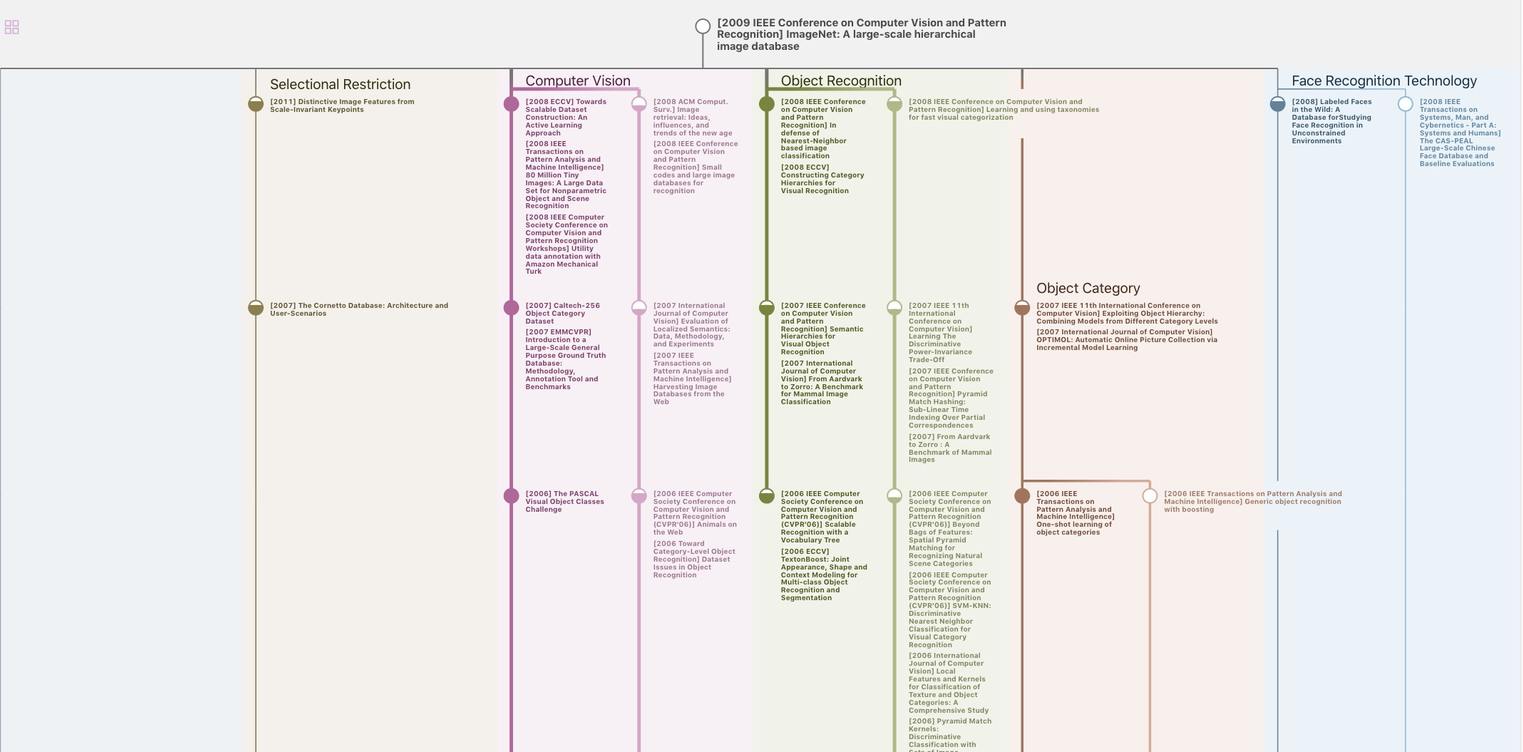
生成溯源树,研究论文发展脉络
Chat Paper
正在生成论文摘要